Google DeepMind Researchers Introduce RT-2: A Novel Imaginative and prescient-Language-Motion (VLA) Mannequin that Learns from each Net and Robotics Information and Turns it into Motion
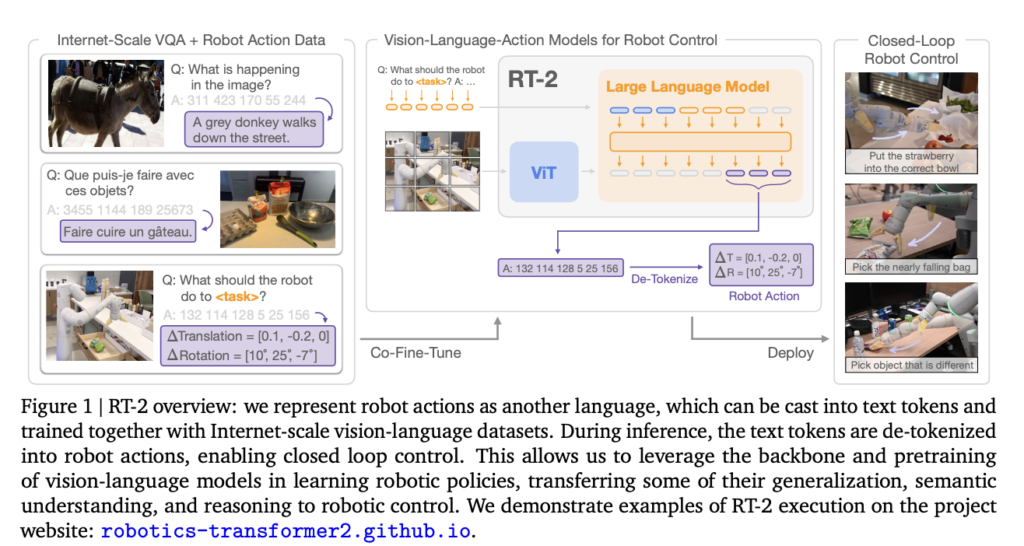
Massive language fashions can allow fluent textual content technology, emergent problem-solving, and artistic technology of prose and code. In distinction, vision-language fashions allow open-vocabulary visible recognition and may even make complicated inferences about object-agent interactions in pictures. One of the best ways for robots to be taught new expertise must be clarified. In comparison with the billions of tokens and photographs used to coach probably the most superior language and vision-language fashions on the net, the quantity of information collected from robots is unlikely to be comparable. Nonetheless, additionally it is difficult to right away adapt such fashions to robotic actions since these fashions purpose about semantics, labels, and textual prompts. In distinction, robots should be instructed in low-level actions, resembling these utilizing the Cartesian end-effector.
Google Deepmind’s analysis goals to enhance generalization and allow emergent semantic reasoning by straight incorporating vision-language fashions skilled on Web-scale knowledge into end-to-end robotic management. With the assistance of web-based language and vision-language knowledge, we intention to make a single, comprehensively skilled mannequin to be taught to hyperlink robotic observations to actions. They suggest fine-tuning state-of-the-art vision-language fashions collectively utilizing knowledge from robotic trajectories and large-scale visible question-answering workout routines performed over the Web. In distinction to different strategies, they suggest an easy, all-purpose recipe: specific robotic actions as textual content tokens and incorporate them straight into the mannequin’s coaching set as pure language tokens would. Researchers examine vision-language-action fashions (VLA), and RT-2 instantiates one such mannequin. By rigorous testing (6k evaluation trials), they may confirm that RT-2 acquired varied emergent expertise via Web-scale coaching and that the method led to performant robotic insurance policies.
Google DeepMind unveiled RT-2, a Transformer-based mannequin skilled on the web-sourced textual content and pictures that may straight carry out robotic operations, as a follow-up to its Robotics Transformer mannequin 1. They use robotic actions to signify a second language that may be transformed into textual content tokens and taught alongside large-scale vision-language datasets out there on-line. Inference entails de-tokenizing textual content tokens into robotic behaviors that may then be managed by way of a suggestions loop. This allows transferring a number of the generalization, semantic comprehension, and reasoning of vision-language fashions to studying robotic insurance policies. On the undertaking web site, accessible at https://robotics-transformer2.github.io/, the workforce behind RT-2 supplies stay demonstrations of its use.
The mannequin retains the power to deploy its bodily expertise in methods in keeping with the distribution discovered within the robotic knowledge. Nonetheless, it additionally learns to make use of these expertise in novel contexts by studying visuals and linguistic instructions utilizing information gathered from the net. Although semantic cues like exact numbers or icons aren’t included within the robotic knowledge, the mannequin can repurpose its realized pick-and-place expertise. No such relations had been provided within the robotic demos, but the mannequin may decide the right object and place it within the right location. As well as, the mannequin could make much more complicated semantic inferences if the command is supplemented with a series of thought prompting, resembling understanding {that a} rock is your best option for an improvised hammer or an power drink is your best option for somebody drained.
Google DeepMind’s key contribution is RT-2, a household of fashions created by fine-tuning big vision-language fashions skilled on web-scale knowledge to function generalizable and semantically conscious robotic guidelines. Experiments probe fashions with as a lot as 55B parameters, realized from publicly out there knowledge and annotated with robotic movement instructions. Throughout 6,000 robotic evaluations, they reveal that RT-2 permits appreciable advances in generalization over objects, scenes, and directions and shows a spread of emergent skills which might be a byproduct of web-scale vision-language pretraining.
Key Options
- The reasoning, image interpretation, and human identification capabilities of RT-2 can be utilized in a variety of sensible situations.
- The outcomes of RT-2 reveal that pretraining VLMs utilizing robotic knowledge can flip them into highly effective vision-language-action (VLA) fashions that may straight management a robotic.
- A promising route to pursue is to assemble a general-purpose bodily robotic that may suppose, problem-solve, and interpret info for finishing varied actions within the precise world, like RT-2.
- Its adaptability and effectivity in dealing with varied duties are displayed in RT-2’s capability to switch info from language and visible coaching knowledge to robotic actions.
Limitations
Regardless of its encouraging generalization properties, RT-2 suffers from a number of drawbacks. Though research recommend that incorporating web-scale pretraining via VLMs improves generalization throughout semantic and visible ideas, this doesn’t give the robotic any new skills relating to its capability to carry out motions. Although the mannequin can solely use the bodily skills discovered within the robotic knowledge in novel methods, it does be taught to make higher use of its skills. They attribute this to a necessity for extra variety within the pattern alongside the size of competence. New data-gathering paradigms, resembling movies of people, current an intriguing alternative for future analysis into buying new expertise.
To sum it up, Google DeepMind researchers demonstrated that huge VLA fashions might be run in real-time, however this was at a substantial computational expense. As these strategies are utilized to conditions requiring high-frequency management, real-time inference dangers turn into a big bottleneck. Quantization and distillation approaches that might let such fashions function sooner or on cheaper {hardware} are enticing areas for future examine. That is associated to a different current restriction in that comparatively few VLM fashions will be utilized to develop RT-2.
Researchers from Google DeepMind summarized the method of coaching vision-language-action (VLA) fashions by integrating pretraining with vision-language fashions (VLMs) and knowledge from robotics. They then launched two variants of VLAs (RT-2-PaLM-E and RT-2-PaLI-X) that PaLM-E and PaLI-X, respectively impressed. These fashions are fine-tuned with knowledge on robotic trajectories to generate robotic actions, that are tokenized as textual content. Extra crucially, they demonstrated that the method improves generalization efficiency and emergent capabilities inherited from web-scale vision-language pretraining, resulting in very efficient robotic insurance policies. In keeping with Google DeepMind, the self-discipline of robotic studying is now strategically positioned to revenue from enhancements in different fields because of this simple and common methodology.
Take a look at the Paper and Reference Article. All Credit score For This Analysis Goes To the Researchers on This Undertaking. Additionally, don’t overlook to hitch our 27k+ ML SubReddit, 40k+ Facebook Community, Discord Channel, and Email Newsletter, the place we share the newest AI analysis information, cool AI tasks, and extra.
Dhanshree Shenwai is a Pc Science Engineer and has a great expertise in FinTech corporations masking Monetary, Playing cards & Funds and Banking area with eager curiosity in purposes of AI. She is keen about exploring new applied sciences and developments in immediately’s evolving world making everybody’s life straightforward.