Google AI Proposes A Common Coverage (UniPi) That Addresses Environmental Variety And Reward Specification Challenges
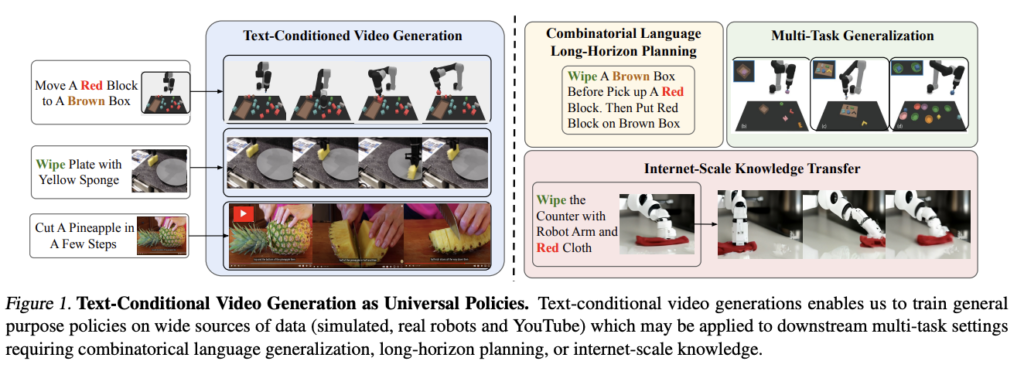
Whatever the trade they’re employed in, synthetic intelligence (AI) and machine studying (ML) applied sciences have all the time tried to enhance the standard of life for individuals. One of many main purposes of AI in latest instances is to design and create brokers that may accomplish decision-making duties throughout numerous domains. For example, massive language fashions like GPT-3 and PaLM and imaginative and prescient fashions like CLIP and Flamingo have confirmed to be exceptionally good at zero-shot studying of their respective fields. Nonetheless, there may be one prime disadvantage related to coaching such brokers. It’s because such brokers exhibit the inherent property of environmental variety throughout coaching. In easy phrases, coaching for various duties or environments necessitates using numerous state areas, which might sometimes impede studying, information switch, and the generalization potential of fashions throughout domains. Furthermore, for reinforcement studying (RL) primarily based duties, creating reward capabilities for particular duties throughout environments turns into tough.
Engaged on this downside assertion, a workforce from Google Analysis investigated whether or not such instruments can be utilized to assemble extra all-purpose brokers. For his or her analysis, the workforce particularly centered on text-guided picture synthesis, whereby the specified purpose within the type of textual content is fed to a planner, which creates a sequence of frames that characterize the meant plan of action, after which management actions are extracted from the generated video. The Google workforce, thus, proposed a Common Coverage (UniPi) that addresses challenges in environmental variety and reward specification of their latest paper titled “Studying Common Insurance policies by way of Textual content-Guided Video Era.” The UniPi coverage makes use of textual content as a common interface for activity descriptions and video as a common interface for speaking motion and commentary conduct in numerous conditions. Particularly, the workforce designed a video generator as a planner that accepts the present picture body and a textual content immediate stating the present purpose as enter to generate a trajectory within the type of a picture sequence or video. The generated video is then fed into an inverse dynamics mannequin that extracts underlying actions executed. This method stands out because it permits the common nature of language and video to be leveraged in generalizing to novel targets and duties throughout numerous environments.
Over the previous few years, vital progress has been achieved within the text-guided picture synthesis area, which has yielded fashions with an distinctive functionality of producing subtle pictures. This additional motivated the workforce to decide on this as their decision-making activity. The UniPi method proposed by Google researchers primarily consists of 4 parts: trajectory consistency by way of tiling, hierarchical planning, versatile conduct modulation, and task-specific motion adaptation, that are described intimately as follows:
1. Trajectory consistency by way of tiling:
Current text-to-video strategies typically produce movies with a considerably altering underlying setting state. Nonetheless, making certain the setting is fixed all through all timestamps is crucial to construct an correct trajectory planner. Thus, to implement setting consistency in conditional video synthesis, the researchers moreover present the noticed picture whereas denoising every body within the synthesized video. In an effort to retain the underlying setting state throughout time, UniPi immediately concatenates every noisy intermediate body with the conditioned noticed picture throughout sampling steps.
2. Hierarchical Planning:
It’s tough to generate all the required actions when planning in complicated and complex environments that require a whole lot of time and measures. Planning strategies overcome this difficulty by leveraging a pure hierarchy by creating tough plans in a smaller house and refining them into extra detailed plans. Equally, within the video era course of, UniPi first creates movies at a rough degree demonstrating the specified agent conduct after which improves them to make them extra reasonable by filling within the lacking frames and making them smoother. That is accomplished through the use of a hierarchy of steps, with every step bettering the video high quality till the specified degree of element is reached.
3. Versatile behavioral modulation:
Whereas planning a sequence of actions for a smaller purpose, one can simply embrace exterior constraints to change the generated plan. This may be accomplished by incorporating a probabilistic prior that displays the specified limitations primarily based on the properties of the plan. The prior could be described utilizing a discovered classifier or a Dirac delta distribution on a specific picture to information the plan towards particular states. This method can be suitable with UniPi. The researchers employed the video diffusion algorithm to coach the text-conditioned video era mannequin. This algorithm consists of encoded pre-trained language options from the Textual content-To-Textual content Switch Transformer (T5).
4. Activity-specific motion adaptation:
A small inverse dynamics mannequin is skilled to translate video frames into low-level management actions utilizing a set of synthesized movies. This mannequin is separate from the planner and could be skilled on a separate smaller dataset generated by a simulator. The inverse dynamics mannequin takes enter frames and textual content descriptions of the present targets, synthesizes the picture frames, and generates a sequence of actions to foretell future steps. An agent then executes these low-level management actions utilizing closed-loop management.
To summarize, the researchers from Google have made a powerful contribution by showcasing the worth of utilizing text-based video era to characterize insurance policies able to enabling combinatorial generalization, multi-task studying, and real-world switch. The researchers evaluated their method on a lot of novel language-based duties, and it was concluded that UniPi generalizes properly to each seen and unknown mixtures of language prompts, in comparison with different baselines equivalent to Transformer BC, Trajectory Transformer, and Diffuser. These encouraging findings spotlight the potential of using generative fashions and the huge information out there as invaluable sources for creating versatile decision-making methods.
Take a look at the Paper and Google Blog. Don’t neglect to affix our 19k+ ML SubReddit, Discord Channel, and Email Newsletter, the place we share the newest AI analysis information, cool AI initiatives, and extra. If in case you have any questions concerning the above article or if we missed something, be happy to e mail us at Asif@marktechpost.com
🚀 Check Out 100’s AI Tools in AI Tools Club
Khushboo Gupta is a consulting intern at MarktechPost. She is at present pursuing her B.Tech from the Indian Institute of Expertise(IIT), Goa. She is passionate concerning the fields of Machine Studying, Pure Language Processing and Net Growth. She enjoys studying extra concerning the technical discipline by collaborating in a number of challenges.