From Phrases to Worlds: Exploring Video Narration With AI Multi-Modal Fantastic-grained Video Description
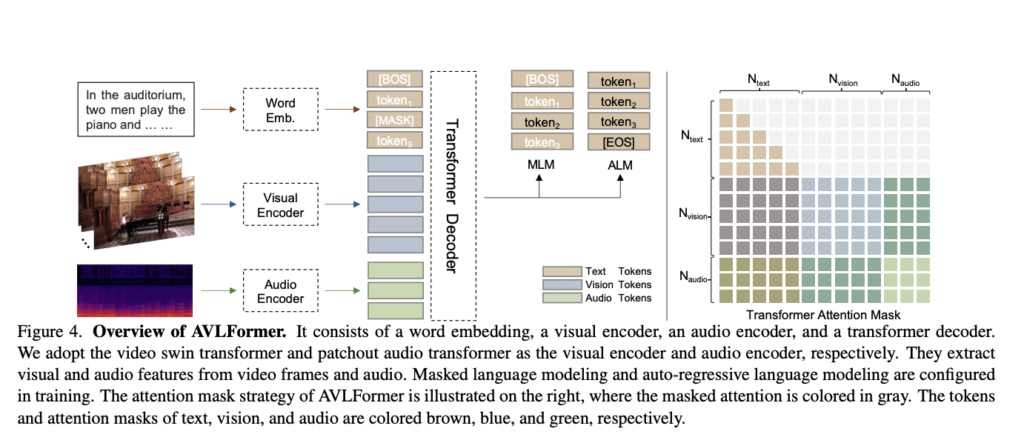
Language is the predominant mode of human interplay, providing extra than simply supplementary particulars to different colleges like sight and sound. It additionally serves as a proficient channel for transmitting data, resembling utilizing voice-guided navigation to steer us to a selected location. Within the case of visually impaired people, they will expertise a film by listening to its descriptive audio. The previous demonstrates how language can improve different sensory modes, whereas the latter highlights language’s capability to convey maximal data in numerous modalities.
Modern efforts in multi-modal modeling attempt to ascertain connections between language and varied different senses, encompassing duties like captioning photos or movies, producing textual representations from photos or movies, manipulating visible content material guided by textual content, and extra.
Nonetheless, in these undertakings, the language predominantly dietary supplements data regarding different sensory inputs. Consequently, these endeavors usually fail to comprehensively depict the intricate alternate of data between totally different sensory modes. They primarily deal with simplistic linguistic parts, resembling one-sentence captions.
Given the brevity of those captions, they solely handle to explain outstanding entities and actions. Consequently, the knowledge conveyed by way of these captions is significantly restricted in comparison with the wealth of data current in different sensory modalities. This discrepancy leads to a notable lack of data when trying to translate data from different sensory realms into language.
On this research, researchers see language as a technique to share data in multi-modal modeling. They create a brand new process known as “Fantastic-grained Audible Video Description” (FAVD), which differs from common video captioning. Often, brief captions of movies confer with the primary elements. FAVD as a substitute requests fashions to explain movies extra like how individuals would, beginning with a fast abstract after which including increasingly more detailed data. This strategy retains a sounder portion of video data inside the language framework.
Since movies enclose visible and auditory indicators, the FAVD process additionally incorporates audio descriptions to boost the excellent depiction. To assist the execution of this process, a brand new benchmark named Fantastic-grained Audible Video Description Benchmark (FAVDBench) has been constructed for supervised coaching. FAVDBench is a group of over 11,000 video clips from YouTube, curated throughout greater than 70 real-life classes. Annotations embody concise one-sentence summaries, adopted by 4-6 detailed sentences about visible features and 1-2 sentences about audio, providing a complete dataset.
To successfully consider the FAVD process, two novel metrics have been devised. The primary metric, termed EntityScore, evaluates the switch of data from movies to descriptions by measuring the comprehensiveness of entities inside the visible descriptions. The second metric, AudioScore, quantifies the standard of audio descriptions inside the function house of a pre-trained audio-visual-language mannequin.
The researchers furnish a foundational mannequin for the freshly launched process. This mannequin builds upon a longtime end-to-end video captioning framework, supplemented by an extra audio department. Furthermore, an enlargement is produced from a visual-language transformer to an audio-visual-language transformer (AVLFormer). AVLFormer is within the type of encoder-decoder buildings as depicted beneath.
Visible and audio encoders are tailored to course of the video clips and audio, respectively, enabling the amalgamation of multi-modal tokens. The visible encoder depends on the video swin transformer, whereas the audio encoder exploits the patchout audio transformer. These parts extract visible and audio options from video frames and audio knowledge. Different parts, resembling masked language modeling and auto-regressive language modeling, are integrated throughout coaching. Taking inspiration from earlier video captioning fashions, AVLFormer additionally employs textual descriptions as enter. It makes use of a phrase tokenizer and a linear embedding to transform the textual content into a selected format. The transformer processes this multi-modal data and outputs a fine-detailed description of the movies supplied as enter.
Some examples of qualitative outcomes and comparability with state-of-the-art approaches are reported beneath.
In conclusion, the researchers suggest FAVD, a brand new video captioning process for fine-grained audible video descriptions, and FAVDBench, a novel benchmark for supervised coaching. Moreover, they designed a brand new transformer-based baseline mannequin, AVLFormer, to deal with the FAVD process. In case you are and need to study extra about it, please be happy to confer with the hyperlinks cited beneath.
Take a look at the Paper and Project. All Credit score For This Analysis Goes To the Researchers on This Venture. Additionally, don’t overlook to affix our 29k+ ML SubReddit, 40k+ Facebook Community, Discord Channel, and Email Newsletter, the place we share the newest AI analysis information, cool AI tasks, and extra.
Should you like our work, please observe us on Twitter
Daniele Lorenzi obtained his M.Sc. in ICT for Web and Multimedia Engineering in 2021 from the College of Padua, Italy. He’s a Ph.D. candidate on the Institute of Info Know-how (ITEC) on the Alpen-Adria-Universität (AAU) Klagenfurt. He’s presently working within the Christian Doppler Laboratory ATHENA and his analysis pursuits embody adaptive video streaming, immersive media, machine studying, and QoS/QoE analysis.