This Paper from Alibaba Unveils DiffusionGAN3D: Revolutionizing 3D Portrait Era and Adaptation with Superior GANs and Textual content-to-Picture Diffusion Fashions
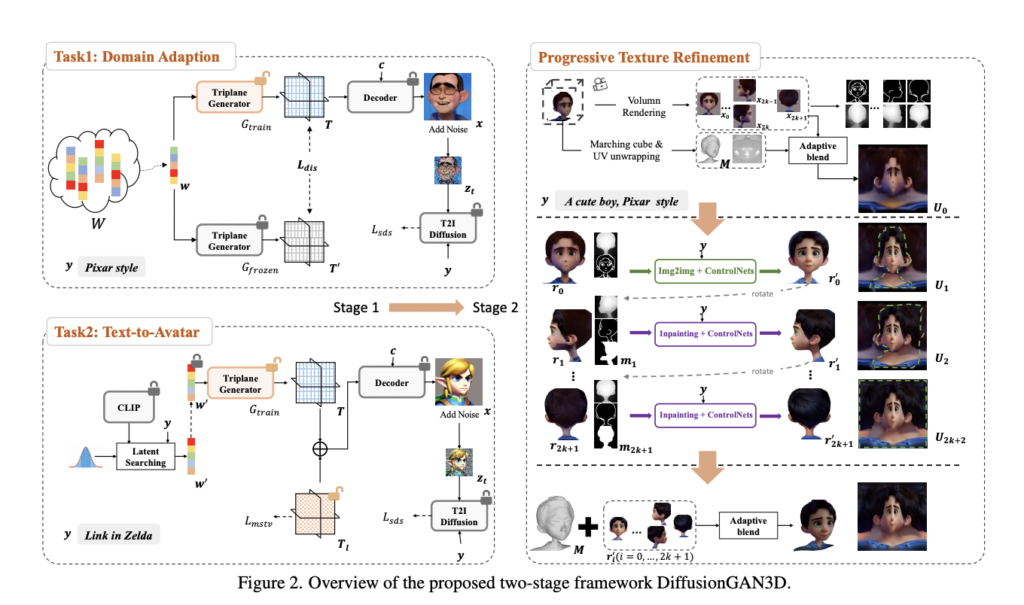
Within the quickly evolving digital imagery and 3D illustration panorama, a brand new milestone is ready by the revolutionary fusion of 3D Generative Adversarial Networks (GANs) with diffusion fashions. The importance of this improvement lies in its capability to deal with longstanding challenges within the subject, significantly the shortage of 3D coaching knowledge and the complexities related to the variable geometry and look of digital avatars.
Historically, 3D stylization and avatar creation methods have leaned closely on switch studying from pre-trained 3D GAN mills. Whereas these strategies introduced spectacular outcomes, they had been tormented by posing bias and demanding computational necessities. Though promising, adversAlthough promising, adversarial finetuning strategies confronted their points in text-image correspondence. The non-adversarial finetuning strategies provided some respite however weren’t with out their limitations, typically struggling to steadiness variety with the diploma of fashion switch.
The introduction of DiffusionGAN3D by researchers from Alibaba Group marks a big leap on this area. The framework ingeniously integrates pre-trained 3D generative fashions with text-to-image diffusion fashions, establishing a sturdy basis for secure and high-quality avatar technology instantly from textual content inputs. This integration is not only about combining two applied sciences; it’s a harmonious mix that leverages every part’s strengths to beat the opposite part’s strengths to beat different’s limitations and highly effective priors, guiding the 3D generator’s finetuning flexibly and effectively.
A deeper dive into the methodology reveals a relative distance loss. This novel addition is essential in enhancing variety throughout area adaption, addressing the lack of variety typically seen with the SDS approach. The framework additionally employs a diffusion-guided reconstruction loss, a strategic transfer designed to enhance texture high quality for area adaption and avatar technology duties. These methodological enhancements are pivotal in addressing earlier shortcomings, providing a extra refined and efficient method to 3D technology.
The efficiency of the DiffusionGAN3D framework is nothing in need of spectacular. In depth experiments showcase its superior efficiency in area adaption and avatar technology, outshining present strategies concerning technology high quality and effectivity. The framework demonstrates outstanding capabilities in producing secure, high-quality avatars and adapting domains with vital element and constancy. Its success is a testomony to the ability of integrating totally different technological approaches to create one thing better than the sum of its components.
In conclusion, the important thing takeaways from this improvement embody:
- DiffusionGAN3D units a brand new commonplace in 3D avatar technology and area adaption.
- Integrating 3D GANs with diffusion fashions addresses longstanding challenges within the subject.
- Revolutionary options like relative distance loss and diffusion-guided reconstruction loss considerably improve the framework’s efficiency.
- The framework outperforms present strategies, considerably advancing digital imagery and 3D illustration.
Try the Paper and Project. All credit score for this analysis goes to the researchers of this challenge. Additionally, don’t neglect to hitch our 35k+ ML SubReddit, 41k+ Facebook Community, Discord Channel, LinkedIn Group, and Email Newsletter, the place we share the most recent AI analysis information, cool AI tasks, and extra.
If you like our work, you will love our newsletter..
Hi there, My title is Adnan Hassan. I’m a consulting intern at Marktechpost and shortly to be a administration trainee at American Specific. I’m presently pursuing a twin diploma on the Indian Institute of Know-how, Kharagpur. I’m captivated with expertise and need to create new merchandise that make a distinction.