Google AI Presents PaLI-3: A Smaller, Quicker, and Stronger Imaginative and prescient Language Mannequin (VLM) that Compares Favorably to Related Fashions which can be 10x Bigger
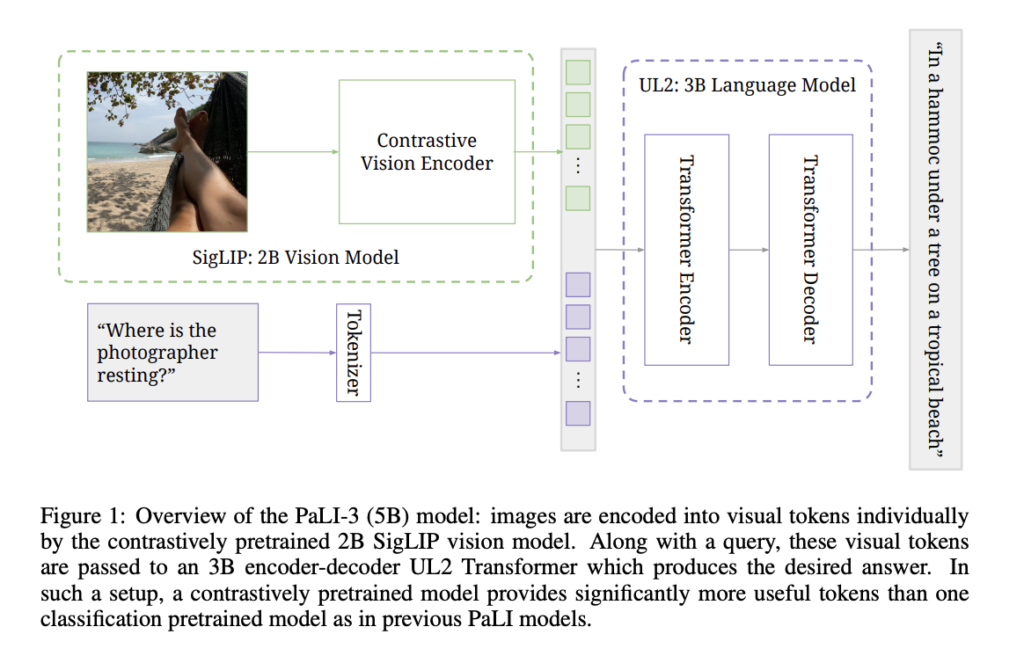
Imaginative and prescient Language Mannequin (VLM) is a sophisticated synthetic intelligence system that mixes pure language understanding with picture recognition capabilities. Like OpenAI’s CLIP and Google’s BigGAN, VLMs can comprehend textual descriptions and interpret photographs, enabling numerous functions in fields corresponding to laptop imaginative and prescient, content material technology, and human-computer interplay. They’ve demonstrated spectacular capabilities in understanding and producing textual content in context with visible content material, making them a pivotal know-how within the AI panorama.
Researchers from Google Analysis, Google DeepMind, and Google Cloud contrasts Imaginative and prescient Transformer (ViT) fashions pre-trained with classification versus contrastive goals, with contrastive pretrained fashions, significantly SigLIP-based PaLI, outperforming in multimodal duties, notably localization and textual content understanding. The researchers scaled the SigLIP picture encoder to 2 billion parameters, reaching a brand new multilingual cross-modal retrieval state-of-the-art. Their examine advocates pre-training visible encoders on web-scale image-text knowledge as a substitute of classification-style knowledge. Their strategy reveals the advantages of scaling up classification pretrained picture encoders, as demonstrated by PaLI-X in giant Imaginative and prescient Language Fashions.
Their examine delves into scaling VLM whereas underscoring the significance of smaller-scale fashions for practicality and environment friendly analysis. It introduces PaLI-3, a 5-billion-parameter VLM with aggressive outcomes. PaLI-3’s coaching course of entails contrastive pre-training of the picture encoder on web-scale knowledge, improved dataset mixing, and higher-resolution coaching. A 2-billion-parameter multilingual contrastive imaginative and prescient mannequin is launched. Ablation research affirm the prevalence of contrastively pretrained fashions, particularly in duties associated to localization and visually-situated textual content understanding.
Their strategy employs a pre-trained ViT mannequin because the picture encoder, particularly ViT-G14, utilizing the SigLIP coaching recipe. ViT-G14 has round 2 billion parameters and serves because the imaginative and prescient spine for PaLI-3. Contrastive pre-training entails embedding photographs and texts individually and classifying their correspondence. Visible tokens from ViT’s output are projected and mixed with textual content tokens. These inputs are then processed by a 3 billion parameter UL2 encoder-decoder language mannequin for textual content technology, usually pushed by task-specific prompts like VQA questions.
PaLI-3 excels in comparison with bigger counterparts, significantly in localization and visually located textual content understanding. The SigLIP-based PaLI mannequin, with contrastive picture encoder pre-training, establishes a brand new multilingual cross-modal retrieval state-of-the-art. The total PaLI-3 mannequin outperforms the state-of-the-art in referring expression segmentation and maintains low error charges throughout subgroups in detection duties. Contrastive pre-training proves simpler for localization duties. The ViT-G picture encoder of PaLI-3 excels in a number of classification and cross-modal retrieval duties.
In conclusion, their analysis emphasizes the advantages of contrastive pre-training, exemplified by the SigLIP strategy, for enhanced and environment friendly VLMs. The smaller 5-billion-parameter SigLIP-based PaLI-3 mannequin excels in localization and textual content understanding, outperforming bigger counterparts on numerous multimodal benchmarks. Contrastive pre-training of the picture encoder in PaLI-3 additionally achieves a brand new multilingual cross-modal retrieval state-of-the-art. Their examine underscores the necessity for complete investigations into numerous elements of VLM coaching past picture encoder pre-training to reinforce mannequin efficiency additional.
Try the Paper. All Credit score For This Analysis Goes To the Researchers on This Venture. Additionally, don’t overlook to affix our 31k+ ML SubReddit, 40k+ Facebook Community, Discord Channel, and Email Newsletter, the place we share the newest AI analysis information, cool AI initiatives, and extra.
If you like our work, you will love our newsletter..
We’re additionally on WhatsApp. Join our AI Channel on Whatsapp..
Sana Hassan, a consulting intern at Marktechpost and dual-degree scholar at IIT Madras, is obsessed with making use of know-how and AI to deal with real-world challenges. With a eager curiosity in fixing sensible issues, he brings a contemporary perspective to the intersection of AI and real-life options.