Will LLMs Exchange Information Graphs? Meta Researchers Suggest ‘Head-to-Tail’: A New Benchmark to Measure the Factual Information of Massive Language Fashions
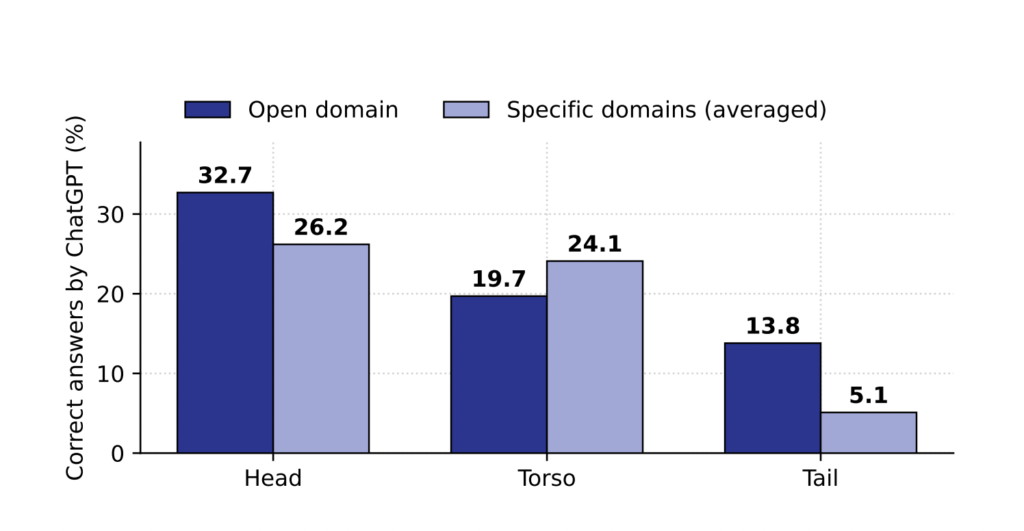
Massive Language Fashions have gathered quite a lot of appreciation for his or her tremendous superb capabilities. They can imitate people and generate content material similar to a human would do. Pre-trained giant language fashions (LLMs), comparable to ChatGPT and LLaMA, have demonstrated astounding aptitudes for understanding the fabric and responding to frequent queries. A number of research have demonstrated their aptitude for internalizing information and responding to inquiries. Although LLMs have considerably superior, they continuously lack a complicated understanding of domain-specific nuances and are susceptible to producing incorrect info, referred to as hallucinations. This highlights the numerous obstacles to bettering LLM accuracy and lowering the incidence of hallucinating responses.
Dialogue associated to LLMs has majorly centered on three primary areas, that are lowering hallucinations in LLM-generated responses, bettering the factual accuracy of LLMs, and speculating on whether or not LLMs may finally exchange Information Graphs (KGs) as a method of storing world information in a symbolic format. Lately, a crew of researchers from Meta Actuality Labs have opted for a recent strategy to reply these questions by making an attempt to find out how a lot info LLMs really possess.
Whereas answering the query of how well-versed LLMs are when it comes to information, the crew has mentioned two facets. Firstly, it may be tough to immediately query the information contained inside an LLM at first. Even when the information is already integrated within the mannequin’s parameters, hallucinations might be brought on by a lack of know-how or a malfunctioning generative mannequin. The research suggests utilizing correctness as a metric to roughly gauge the diploma of data inside an LLM. This entails assessing the mannequin’s capability to reply clear, correct questions like “The place was basketball participant Michael Jordan born?” The LLM can be requested to supply succinct responses and admit uncertainty through the use of the phrase ‘not sure’ when its confidence is low.
Secondly, there is no such thing as a readily accessible benchmark that precisely displays the variety of consumer pursuits or the breadth of data on the planet. Even probably the most complete information graphs present gaps in information, notably with regards to much less well-known information. The question logs from main LLMs or search engines like google and yahoo will not be publicly obtainable.
To deal with all the restrictions, the crew has launched a benchmark they’ve created known as “Head-to-Tail.” This benchmark consists of a set of 18,000 question-answer (QA) pairs which were divided into head, torso, and tail information based mostly on the recognition of their respective topics. Totally different public familiarity ranges are mirrored in these classes. The crew has created an automatic analysis methodology and a set of measures that intently mirror the breadth of data that an LLM has competently assimilated so as to consider the information maintained by LLMs.
The analysis’s core is the analysis of 14 LLMs which might be obtainable to most people. The outcomes confirmed that present LLMs nonetheless want to enhance considerably when it comes to perfecting their comprehension of factual knowledge. That is very true for info that falls throughout the torso-to-tail space and considerations much less well-known organizations.
In conclusion, this analysis examines the factual information of LLMs utilizing a lately proposed benchmark and cutting-edge analysis methods. The work makes a considerable contribution to the persevering with dialogue relating to the dependability and potential developments of massive language fashions in incorporating factual info by addressing vital analysis issues and outlining particular findings.
Take a look at the Paper. All Credit score For This Analysis Goes To the Researchers on This Undertaking. Additionally, don’t overlook to affix our 29k+ ML SubReddit, 40k+ Facebook Community, Discord Channel, and Email Newsletter, the place we share the most recent AI analysis information, cool AI initiatives, and extra.
If you like our work, you will love our newsletter..
Tanya Malhotra is a closing 12 months undergrad from the College of Petroleum & Power Research, Dehradun, pursuing BTech in Pc Science Engineering with a specialization in Synthetic Intelligence and Machine Studying.
She is a Knowledge Science fanatic with good analytical and important pondering, together with an ardent curiosity in buying new abilities, main teams, and managing work in an organized method.