Superhuman Efficiency on the Atari 100K Benchmark: The Energy of BBF – A New Worth-Based mostly RL Agent from Google DeepMind, Mila, and Universite de Montreal
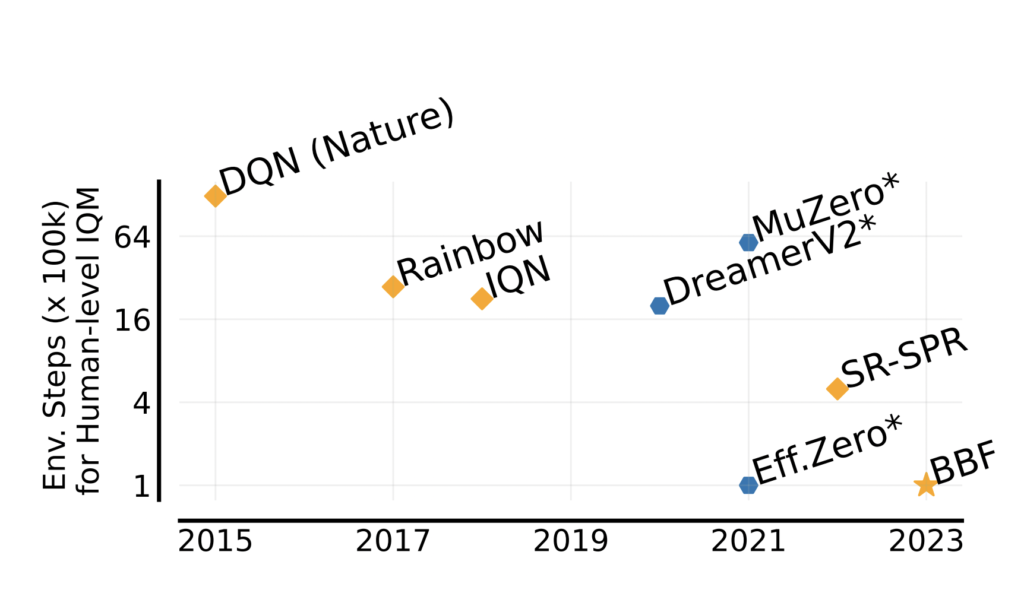
Deep reinforcement studying (RL) has emerged as a robust machine studying algorithm for tackling complicated decision-making duties. To beat the problem of reaching human-level pattern effectivity in deep RL coaching, a crew of researchers from Google DeepMind, Mila, and Universite de Montreal has launched a novel value-based RL agent referred to as “quicker, higher, quicker” (BBF). Of their current paper, “Bigger, Better, Faster: Human-level Atari with human-level efficiency,” the crew presents the BBF agent, demonstrating super-human efficiency on the Atari 100K benchmark utilizing a single GPU.
Addressing the Scaling Difficulty
The analysis crew’s main focus was to handle the scaling subject of neural networks in deep RL when there are restricted samples. Constructing upon the SR-SPR agent developed by D’Oro et al. (2023), which employs a shrink-and-perturb technique, BBF perturbs 50 p.c of the parameters of the convolutional layers towards a random goal. In distinction, SR-SPR perturbs solely 20 p.c of the parameters. This modification ends in improved efficiency of the BBF agent.
Scaling Community Capability
To scale community capability, the researchers make the most of the Impala-CNN community and enhance the dimensions of every layer by 4 occasions. It was noticed that BBF constantly outperforms SR-SPR because the width of the community is elevated, whereas SR-SPR reaches its peak at 1-2 occasions the unique measurement.
Enhancements for Higher Efficiency
BBF introduces an replace horizon part that exponentially decreases from 10 to three. Surprisingly, this modification yields a stronger agent than fixed-value brokers like Rainbow and SR-SPR. Moreover, the researchers apply a weight decay technique and enhance the low cost issue throughout studying to alleviate statistical overfitting points.
Empirical Examine and Outcomes
Of their empirical examine, the analysis crew compares the efficiency of the BBF agent in opposition to a number of baseline RL brokers, together with SR-SPR, SPR, DrQ (eps), and IRIS, on the Atari 100K benchmark. BBF surpasses all opponents when it comes to each efficiency and computational value. Particularly, BBF achieves a 2x enchancment in efficiency over SR-SPR whereas using practically the identical computational assets. Moreover, BBF demonstrates comparable efficiency to the model-based EfficientZero method however with greater than a 4x discount in runtime.
Future Implications and Availability
The introduction of the BBF agent represents a major development in reaching super-human efficiency in deep RL, significantly on the Atari 100K benchmark. The analysis crew hopes their work will encourage future endeavors to push the boundaries of pattern effectivity in deep RL. The code and knowledge related to the BBF agent are publicly out there on the venture’s GitHub repository, enabling researchers to discover and construct upon their findings.
With the introduction of the BBF agent, Google DeepMind and its collaborators have demonstrated outstanding progress in deep reinforcement studying. By addressing the problem of pattern effectivity and leveraging developments in community scaling and efficiency enhancements, the BBF agent achieves super-human efficiency on the Atari 100K benchmark. This work opens up new prospects for enhancing the effectivity and effectiveness of RL algorithms, paving the best way for additional developments within the area.
Verify Out The Paper and Github. Don’t neglect to affix our 23k+ ML SubReddit, Discord Channel, and Email Newsletter, the place we share the newest AI analysis information, cool AI initiatives, and extra. If in case you have any questions relating to the above article or if we missed something, be happy to e-mail us at Asif@marktechpost.com
🚀 Check Out 100’s AI Tools in AI Tools Club
Niharika is a Technical consulting intern at Marktechpost. She is a 3rd 12 months undergraduate, at present pursuing her B.Tech from Indian Institute of Know-how(IIT), Kharagpur. She is a extremely enthusiastic particular person with a eager curiosity in Machine studying, Knowledge science and AI and an avid reader of the newest developments in these fields.