Learnings from a Machine Studying Engineer — Half 1: The Knowledge | by David Martin | Jan, 2025
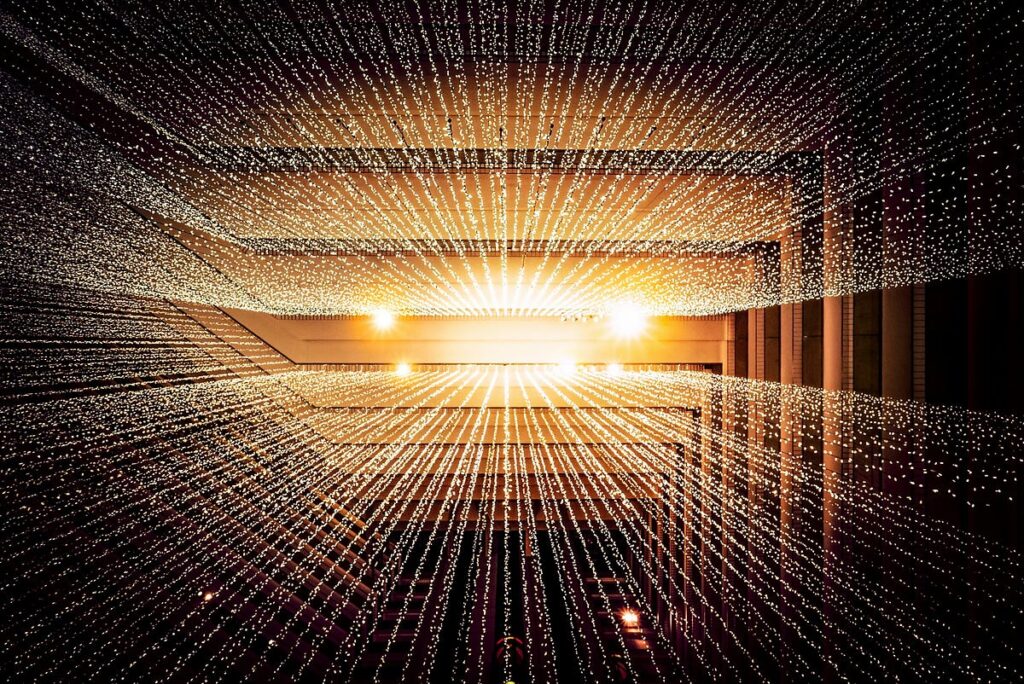
It’s stated that to ensure that a machine studying mannequin to achieve success, it is advisable to have good knowledge. Whereas that is true (and just about apparent), this can be very tough to outline, construct, and maintain good knowledge. Let me share with you the distinctive processes that I’ve realized over a number of years constructing an ever-growing picture classification system and how one can apply these methods to your personal software.
With persistence and diligence, you’ll be able to keep away from the traditional “rubbish in, rubbish out”, maximize your mannequin accuracy, and show actual enterprise worth.
On this sequence of articles, I’ll dive into the care and feeding of a multi-class, single-label picture classification app and what it takes to achieve the best stage of efficiency. I received’t get into any coding or particular consumer interfaces, simply the primary ideas that you could incorporate to fit your wants with the instruments at your disposal.
Here’s a temporary description of the articles. You’ll discover that the mannequin is final on the listing since we have to deal with curating the info firstly:
- Half 1 — The Knowledge — Labelling requirements, lessons and sub-classes