Machine Studying vs. Conventional Analytics: When to Use Which?
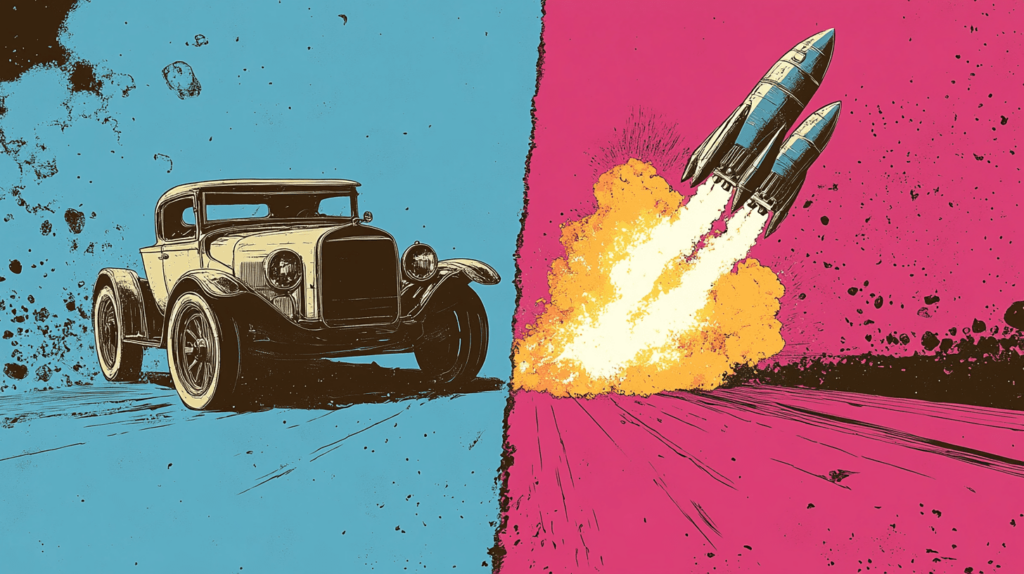
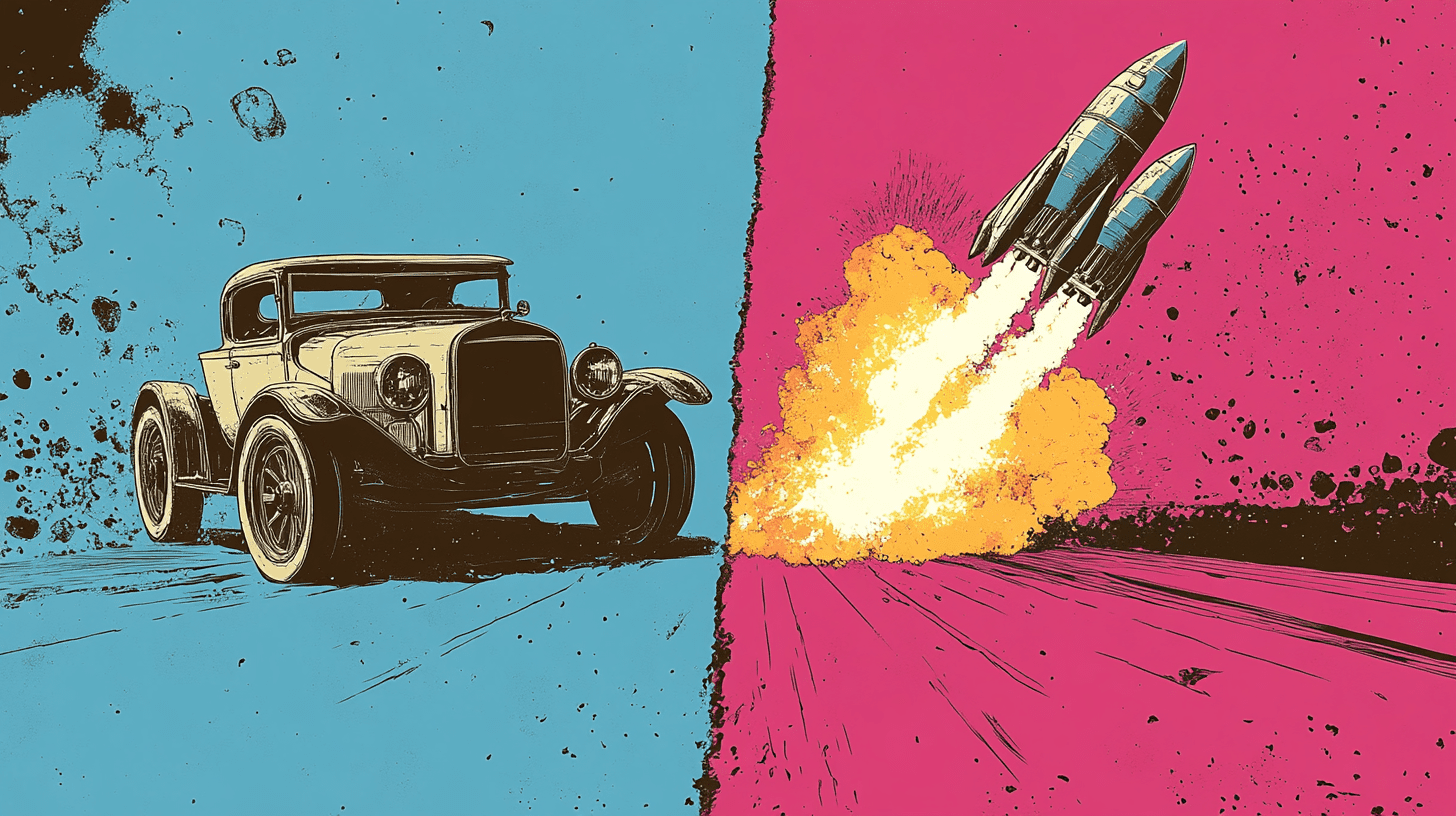
Machine Studying vs. Conventional Analytics: When to Use Which?
Picture by Editor | Midjourney
This text focuses on demystifying the distinction between conventional knowledge analytics strategies vs. machine-learning-driven ones, not with out offering firstly a transparent understanding of what’s — and what’s not — knowledge analytics in comparison with different knowledge phrases usually used interchangeably. After gaining such understanding, the publish offers clear and succinct tips on when to make use of knowledge analytics approaches guided by machine studying modeling vs. utilizing extra conventional approaches inherited from statistics.
What Precisely is Information Analytics vs. Different “Information” Fields?
Let’s be clear about one factor: there may be rather a lot of confusion between knowledge analytics and different associated knowledge fields like knowledge science, huge knowledge, enterprise intelligence, and even knowledge evaluation (sure, knowledge analytics ≠ knowledge evaluation!). So, earlier than leaping into the core query raised on this publish, it’s handy to make clear what knowledge analytics is in comparison with different knowledge phrases.
- Information Evaluation: it focuses on inspecting knowledge to establish developments, patterns, and relationships, usually utilizing a mix of statistical and visualization strategies. Conventional speculation assessments, regression evaluation, variance evaluation, and time collection evaluation methods fall below the umbrella of knowledge evaluation.
- Information Analytics: it concentrates on predictive, descriptive, and prescriptive evaluation, i.e. discovering future patterns and developments to help enterprise decision-making. In essence, knowledge analytics is a refined contextualization and extra domain-specific conceptualization of knowledge evaluation: the distinction is only semantic and utility context-oriented, however underlying strategies are almost an identical in each fields. Buyer segmentation and buyer retention evaluation in advertising and retail will be categorized inside the scope of knowledge analytics processes.
- Information Science: it focuses on the research and engineering processes of complicated knowledge to realize deep insights and data. It usually entails using superior algorithms and modeling methods below the machine studying umbrella, in addition to cleansing, warehousing, and presenting knowledge.
- Large Information: this time period refers back to the means of managing and analyzing big and complicated datasets that can not be successfully handled through the use of conventional knowledge processing approaches. It largely focuses on using {hardware} and distributing computing applied sciences (due to instruments like Hadoop and Spark) for environment friendly, high-performance knowledge dealing with at scale.
- Enterprise Intelligence: it entails the triple means of
gathering, analyzing — often from a descriptive vantage level — and visually presenting knowledge in organizations for supporting choices. While similar to knowledge analytics, enterprise intelligence places extra concentrate on the creation of stories and interactive dashboards to supply related info to decision-makers. Energy BI, Tableau, and QlikView are well-known BI instruments in the marketplace.
Hopefully, the above checklist dispelled a part of the doubts you may need encountered about these carefully interrelated areas. If not, let the beneath diagram do the job!

Variations and overlaps between data-related fields
Picture by Writer
Information Analytics and Machine Studying: When to Use Which?
Now we’re in a greater place for evaluating knowledge analytics and machine studying. Machine studying (ML) is a subarea of synthetic intelligence, whereby software program fashions fueled by knowledge and able to studying by themselves to carry out a activity are constructed. ML fashions carry out duties like classifications, regression, clustering, and so forth, by being uncovered to knowledge used for studying, a.okay.a. coaching knowledge.
ML can typically be used as a knowledge analytics instrument by companies in sure use circumstances, usually of a forecasting nature: predicting gross sales developments, buyer churn, or detecting fraud. In these situations, ML fashions for classification, regression, and anomaly detection, amongst others, can represent highly effective knowledge analytic instruments. Bear in mind, the notion of knowledge analytics is not decided by the methods used -whether ML or not- however by the conjunction of knowledge evaluation approaches plus an utility contextualization of enterprise decision-making help.
This implies many trendy knowledge analytics processes and methodologies make use of ML methods as a part of them. However not all. ML just isn’t at all times the go-to method for knowledge analytics in enterprise settings. And that is the place the preliminary query that gave a title to this text arises: when to make use of which? Now that we now have a strong understanding of those two phrases and different very interrelated ones, we’re within the perfect place to reply the query and wrap up the article.
When to Use Machine Studying
The usage of ML for analytics functions is inspired when:
- There’s a have to make predictions or automate choices from giant and complicated datasets: situations embrace buyer segmentation upon complicated buyer conduct knowledge, recommender programs that predict and counsel probably wanted or favored merchandise to a buyer primarily based on analyzing their purchasing habits, and so forth.
- Patterns to be discovered beneath the info are too intricate for guide evaluation: that is particularly the case in duties involving unstructured knowledge, like picture recognition and classification, and pure language processing e.g. to categorizing the sentiment in buyer critiques.
When to Use Conventional Analytics
Alternatively, using conventional analytics strategies like statistics-based ones is a greater choice when:
- There’s the objective of understanding historic knowledge, figuring out developments, or testing hypotheses utilizing approaches like variance evaluation and regression evaluation.
- Working with smaller and easier datasets the place the main target is on explaining relationships and correlations between knowledge variables clearly and interpretably, e.g. correlations between merchandise bought, gross sales developments, and so on.
Wrapping Up
With these tips and the gained understanding of the phrases and fields surrounding knowledge analytics and ML, the following time you might be confronted with the necessity for making use of knowledge analytics strategies as a part of a enterprise case, you’ll absolutely be in a terrific place to decide on the appropriate plan of action.