Google AI Analysis Examines Random Circuit Sampling (RCS) for Evaluating Quantum Pc Efficiency within the Presence of Noise
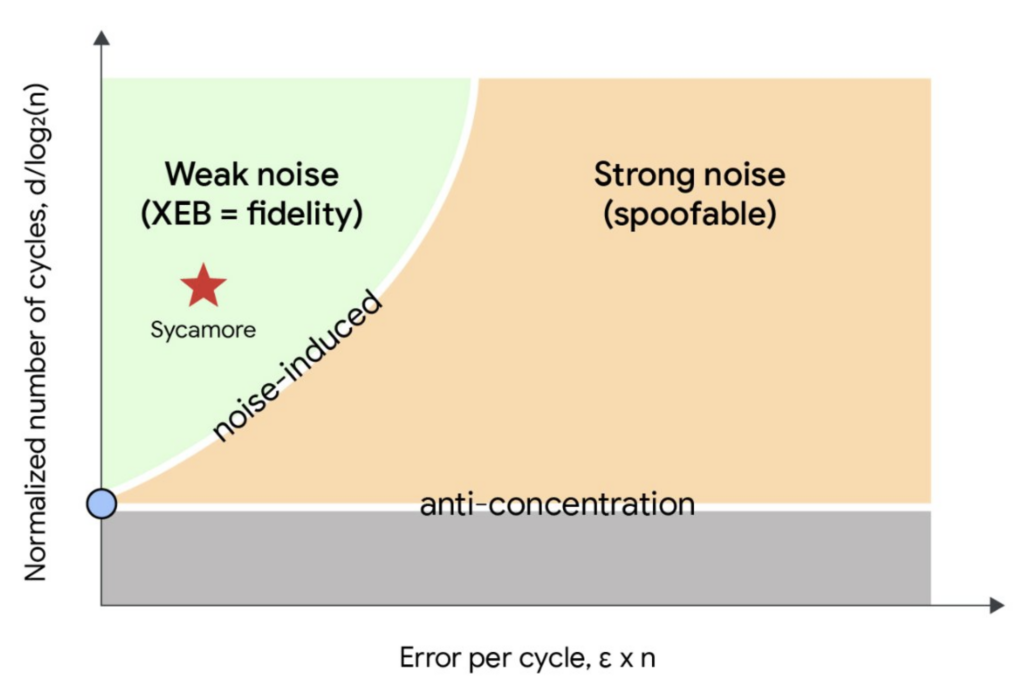
Quantum computer systems are a revolutionary know-how that harnesses the rules of quantum mechanics to carry out calculations that will be infeasible for classical computer systems. Evaluating the efficiency of quantum computer systems has been a difficult activity on account of their sensitivity to noise, the complexity of quantum algorithms, and the restricted availability of highly effective quantum {hardware}. Decoherence and errors launched by noise can considerably have an effect on the accuracy of quantum computations. Researchers have made a number of makes an attempt to investigate how noise impacts the power of quantum computer systems to carry out helpful computations.
Google researchers tackle the problem of evaluating quantum laptop efficiency within the noisy intermediate-scale quantum (NISQ) period, the place quantum processors are extremely vulnerable to noise. The elemental downside is figuring out whether or not quantum techniques, regardless of their noise limitations, can outperform classical supercomputers in particular computational duties. The analysis focuses on understanding how quantum computer systems behave below noise and whether or not they can nonetheless show quantum benefit—a key milestone in quantum computing.
Random circuit sampling (RCS) has emerged as a number one methodology to guage quantum processors and was launched in 2019. RCS duties are computationally arduous for classical computer systems as a result of exponential progress of data as quantum circuits scale. The important thing downside is that classical computer systems battle to simulate or pattern from a quantum circuit’s output distribution as circuit quantity will increase. RCS measures quantum circuit quantity, a key indicator of efficiency, which helps determine when quantum techniques can surpass classical supercomputers, even within the presence of noise. Google’s analysis confirmed a twofold improve in circuit quantity whereas sustaining the identical constancy as earlier benchmarks. These developments counsel that noisy quantum techniques can nonetheless provide sensible worth by performing duties past classical capabilities.
The proposed methodology includes benchmarking quantum gadgets utilizing RCS to estimate constancy, measuring how intently the noisy quantum processor mimics a perfect, noise-free system. Researchers launched patch cross-entropy benchmarking (XEB), a way to confirm constancy by dividing the total quantum processor into smaller patches. XEB calculations for these patches present a possible solution to estimate constancy for bigger circuits. The research confirms that regardless of the noise, present quantum processors like Sycamore are able to reaching beyond-classical outcomes, doubling the circuit quantity in comparison with earlier experiments whereas sustaining constancy. It additionally identifies part transitions in RCS conduct based mostly on noise power and circuit depth, additional validating the reliability of RCS for assessing quantum computer systems.
Together with the affect of noise on quantum processors, Google researchers found two distinct noise-induced part transitions. In low-noise situations, quantum computer systems can obtain full computational energy. Nevertheless, excessive noise ranges can create uncorrelated subsystems, making it simpler for classical computer systems to simulate their outcomes. This part transition helps decide if quantum computer systems are actually outperforming classical computer systems. The Sycamore processor operates in a low-noise regime, confirming its quantum benefit.
In conclusion, Google researchers present a major step in the direction of fault-tolerant quantum computing by demonstrating how random circuit sampling can successfully measure quantum efficiency within the presence of noise. The invention of noise-induced part transitions presents a brand new solution to perceive the conduct of quantum processors below completely different situations.
Try the Paper and Details here. All credit score for this analysis goes to the researchers of this challenge. Additionally, don’t overlook to comply with us on Twitter and be a part of our Telegram Channel and LinkedIn Group. In the event you like our work, you’ll love our newsletter.. Don’t Neglect to hitch our 50k+ ML SubReddit.
[Upcoming Live Webinar- Oct 29, 2024] The Best Platform for Serving Fine-Tuned Models: Predibase Inference Engine (Promoted)
Pragati Jhunjhunwala is a consulting intern at MarktechPost. She is presently pursuing her B.Tech from the Indian Institute of Expertise(IIT), Kharagpur. She is a tech fanatic and has a eager curiosity within the scope of software program and information science purposes. She is all the time studying in regards to the developments in numerous subject of AI and ML.