Past the Mud: Datasets, Benchmarks, and Strategies for Pc Imaginative and prescient in Off-Street Racing – Machine Studying Weblog | ML@CMU

TL;DR: Off-the-shelf textual content recognizing and re-identification fashions fail in primary off-road racing settings, much more so throughout muddy occasions. Making issues worse, there aren’t any public datasets to guage or enhance fashions on this area. To this finish, we introduce datasets, benchmarks, and strategies for the difficult off-road racing setting.
Within the dynamic world of sports activities analytics, machine studying (ML) techniques play a pivotal function, remodeling huge arrays of visible information into actionable insights. These techniques are adept at navigating by means of hundreds of images to tag athletes, enabling followers and members alike to swiftly find pictures of particular racers or moments from occasions. This know-how has seamlessly built-in into various sports, considerably enhancing the spectator expertise and operational effectivity. But, not all sports activities environments cater equally to the capabilities of present ML fashions. Off-road motorbike racing, characterised by its unpredictable and untamed wilderness settings, poses distinctive challenges that push the boundaries of what current laptop imaginative and prescient techniques can deal with.
Think about the circumstances beneath which off-road races are carried out: racers blitz by means of waist-deep mud holes, endure torrential rains, navigate by means of blinding mud clouds, and way more. Such excessive environmental elements introduce variables like mud occlusion, complicated poses (racers steadily crash), glare, movement blur, and variable lighting circumstances, which considerably degrade the efficiency of typical text spotting and person re-identification (ReID) fashions. Typical fashions, skilled on extra ‘sterile’ circumstances, falter when confronted with the duty of figuring out racers and their numbers within the chaotic and mud-splattered scenes typical of off-road racing occasions. Take, for instance, these pictures of the identical racer, taken solely minutes aside:
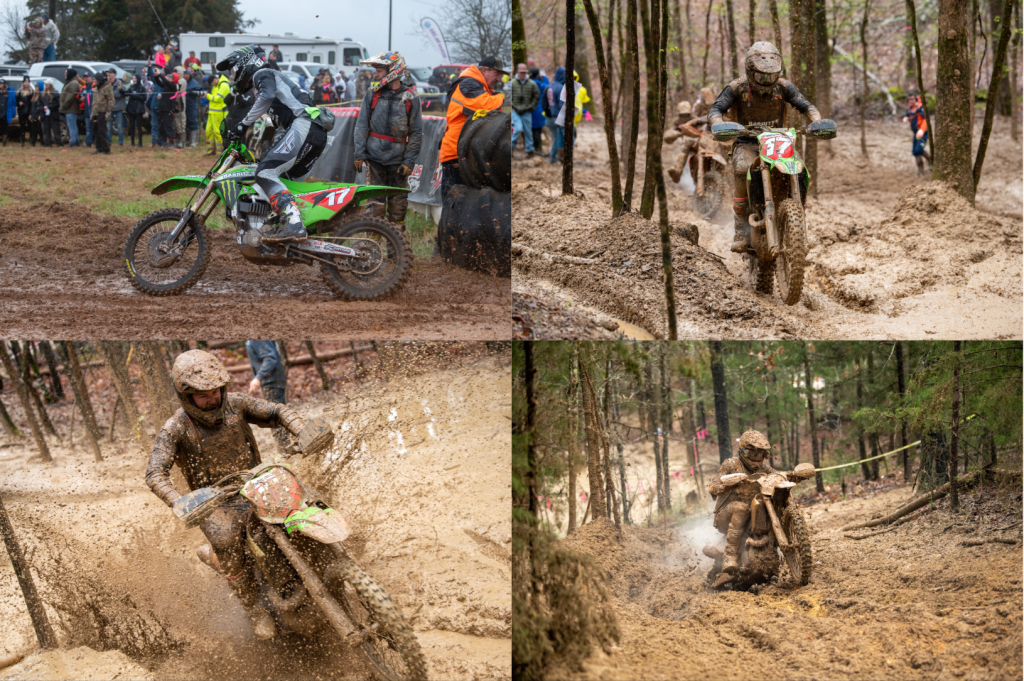
The dearth of public datasets tailor-made to those rugged circumstances exacerbates the issue, leaving researchers and practitioners with out the sources wanted to guage and improve fashions for higher efficiency in off-road racing, or equally unconstrained, eventualities. Recognizing this hole, our work goals to bridge it by introducing new datasets and benchmarks particularly designed for the difficult setting of off-road motorbike racing. This weblog publish will delve into the distinctive challenges introduced by off-road racing environments, describe our efforts in creating datasets that seize these circumstances, and focus on strategies and benchmarks for enhancing laptop imaginative and prescient fashions to robustly deal with the intense variability inherent in off-road racing. I’ll even give a short overview of some new weakly supervised strategies for enhancing fashions in these difficult areas, with little or no labeled information. Take part as we discover the uncharted territories of machine studying functions in off-road motorbike racing, pushing the boundaries of what’s potential in sports activities analytics and past.
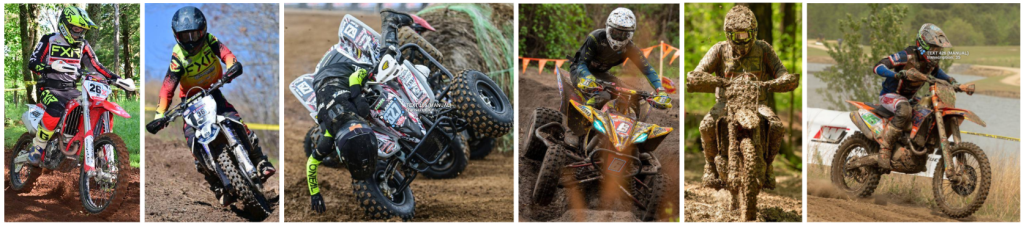
Off-road motorbike racing is an adrenaline-pumping sport that takes athletes and their machines by means of a few of the most difficult terrains nature has to supply. In contrast to the comparatively predictable environments of monitor racing or city marathons, off-road racing is fraught with unpredictability and excessive circumstances. The very essence of what makes it thrilling for members and spectators alike—mud, mud, water, uneven terrain—presents a formidable problem for laptop imaginative and prescient techniques. Right here, we delve into the particular hurdles that these circumstances pose for textual content recognizing and re-identification fashions in off-road racing eventualities.
Filth is pervasive in off-road racing, manifesting itself as mud or mud. As races progress, automobiles and riders grow to be more and more coated in filth, which may obscure important figuring out options equivalent to racer numbers or distinguishing gear colours. The dynamic nature of off-road racing implies that athletes are not often in easy, upright poses. As an alternative, they navigate the course by means of jumps, sharp turns, and even crashes. The outside settings of off-road races usually transfer quickly from deep darkish forests to shiny evident fields, thus introducing variable lighting circumstances. Equally, the excessive speeds at which racers transfer mixed with the stylistic selections of some photographers can result in movement blur. In every of those circumstances, conventional optical character recognition (OCR) and re-identification (ReID) fashions, skilled totally on clear, unobstructed pictures, wrestle to acknowledge textual content or establish people.
To sort out the formidable challenges introduced by off-road motorbike racing, we launched into a mission to create datasets that precisely seize the essence and extremities of this sport. Recognizing the hole in current laptop imaginative and prescient sources, our datasets—off-road Racer Quantity Dataset (RND) and MUddy Racer re-iDentification Dataset (MUDD)—are meticulously curated to function a sturdy basis for growing and benchmarking fashions able to working within the harsh, unpredictable circumstances of off-road racing. These datasets, in addition to benchmarking code, are publically accessible for each of those datasets. You’ll find RND here and MUDD here.
Determine 3 particulars the textual content recognizing outcomes on the RND dataset. Outcomes are damaged down by the assorted forms of occlusion within the dataset. Even on the cleanest information (i.e. the information with no occlusion), the most effective fine-tuned fashions attain a most E2E F1 rating of 0.6, leaving quite a bit to be desired. Introducing any of the aforementioned challenges (i.e.) reduces this even additional, right down to the more serious end-to-end F1 rating of 0.29. The fashions examined had been the Yet Another Mask Text Spotter (YAMTS) and Swin Text Spotter, and YAMTS was persistently the most effective performing. Nice-tuning reduces the adverse impact of the assorted occlusion varieties (i.e. the blue bar modifications much less as a proportion of efficiency than the orange throughout the assorted occlusions), but occlusion nonetheless causes vital efficiency degradation.
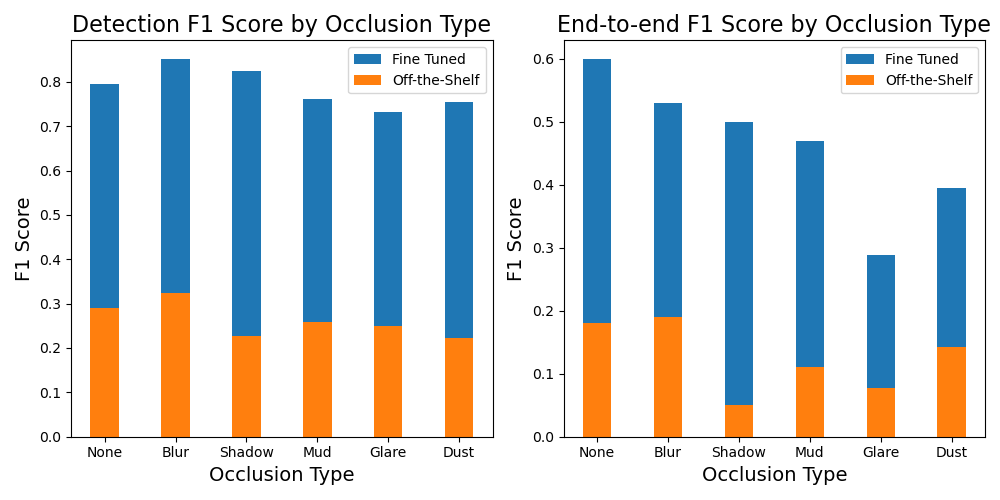
Determine 4 breaks down the efficiency of our greatest ReID fashions. In the usual ReID analysis setting, a pattern from a question set is used to return a rating over a gallery set. We report the rank1 accuracy together with the imply common precision (mAP). Determine 2 seems to be at two variations of the question and gallery units, one question set of all of the muddy pictures, and one with out, and the identical for the gallery set. Within the easiest setting (No Mud -> No Mud), mannequin efficiency is getting moderately good, round 0.9 mAP. Nonetheless, mud drops this efficiency by as a lot as 30%. The fashions examined had been the Omni-Scale Network (OSNet) and Resnet 50. Determine 4 stories outcomes from OSNet because it was essentially the most performant.
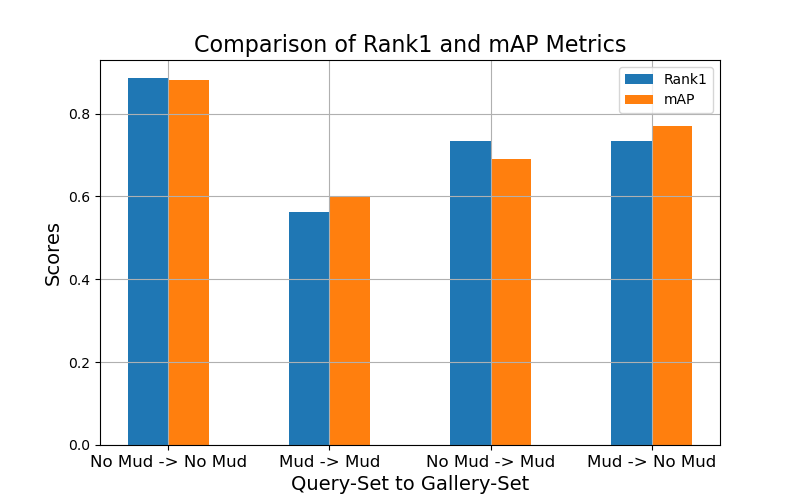
In abstract, the off-road racing setting is troublesome, even in the most effective case. As soon as filth and dirt enter the equation, fashions require development earlier than they attain the edge of usability in a real-world utility.
A “Mud-Like” Knowledge Augmentation
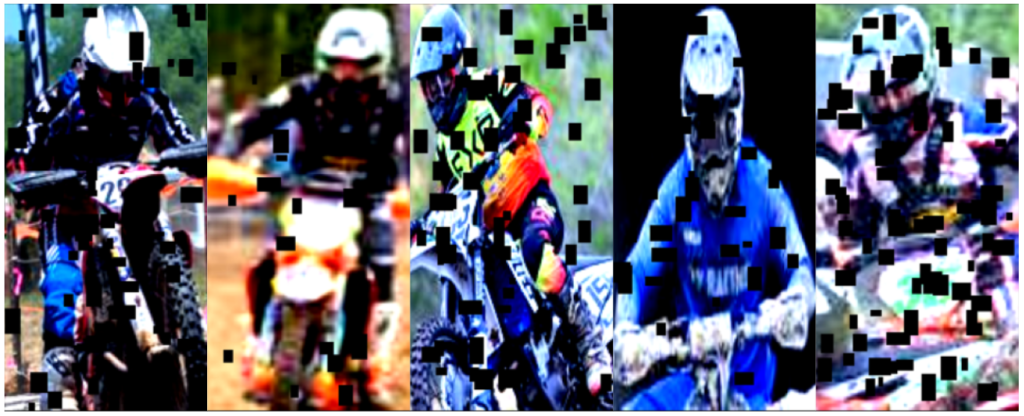
Step one in constructing robustness to mud is to introduce an information augmentation technique: speckling. As proven in earlier examples, mud usually accumulates in small chunks. To emulate this, we introduce speckling, the place we randomly change many small patches of the enter imagery into the pixel imply. That is much like random erasing however at a a lot smaller scale with numerous patches being erased in every picture. This system results in a 4% enchancment in Rank-1 accuracy for individual re-identification on the MUDD dataset, and whereas it doesn’t meaningfully have an effect on the detection F1 rating of textual content recognizing on RND, it does enhance the end-to-end F1 rating by 7%. Whereas we additionally use the usual coloration jitter information augmentation to assist robustness to the colour modifications induced as a racer will get soiled, extra analysis is required to find out if a extra particular coloration augmentation can show helpful.
Studying from Weak Labels
One other intricacy of sports activities imagery that we are able to reap the benefits of is the pure groupings that usually exist. For instance, prior marathon imagery has been manually grouped by people, such that every group (which we are going to consult with as a bag) consists of pictures that every one include a selected particular person. Nonetheless, which particular particular person is the considered one of curiosity in every picture is unknown. In motorbike racing, we’ve the identical information, in addition to buyer buy historical past. Most clients buy images of a single racer, due to this fact the listing of bought images once more turns into a bag of a selected particular person, though which people within the picture is unknown. This sort of label is visualized in Determine 4.
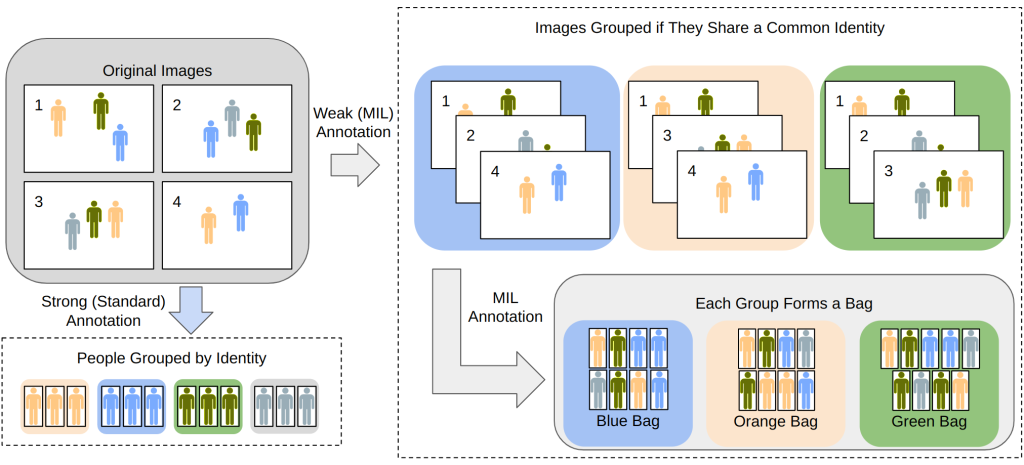
We introduce Contrastive A number of Occasion Studying (CMIL) to deal with this problem. This methodology works by producing bag representations from the entire occasion representations that comprise that bag. Then, the bag representations are used to optimize a mannequin by way of triplet loss or classification loss. In different phrases, we optimize the mannequin to precisely classify luggage, not people. This doesn’t align with our take a look at time objective, nonetheless, of classifying people. However surprisingly, our bag classification fashions naturally generate helpful particular person representations. Determine 5 offers an summary of the CMIL mannequin. On the MUDD dataset, CMIL improves over the next-best weakly labeled person re-identification methodology by 4% rank-1 accuracy, and over a mannequin that trusts the bag-level labels to be correct person-level labels by over 20%.
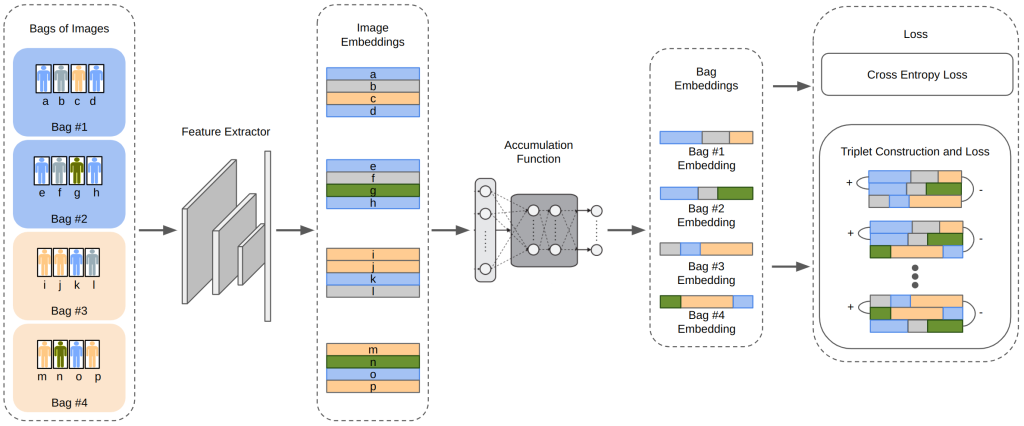
Off-road racing poses main challenges to current textual content recognizing and individual re-identification strategies and fashions, rendering them unfit for sensible utility. Our first steps at enhancing laptop efficiency in these areas embrace introducing two datasets for the corresponding issues, introducing a brand new information augmentation method, and bringing contrastive studying to the a number of occasion studying framework. We hope that these preliminary works spur extra innovation in off-road functions.
For extra data, you could find the papers and code this weblog publish relies on right here:
– Beyond the Mud: Datasets and Benchmarks for Computer Vision in Off-Road Racing (code)
– Contrastive Multiple Instance Learning for Weakly Supervised Person ReID (code)