Meet the Matryoshka Embedding Fashions that Produce Helpful Embeddings of Numerous Dimensions
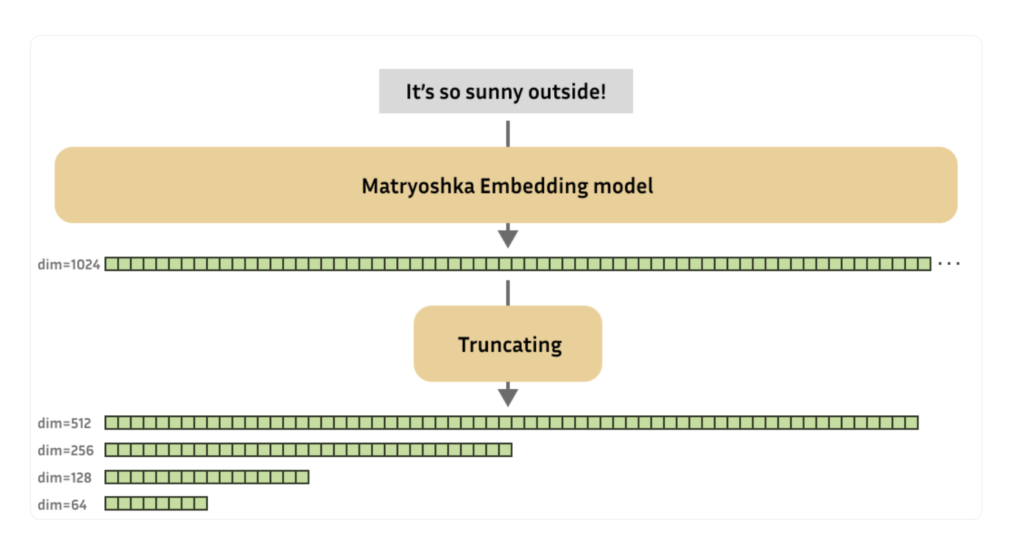
Within the considerably growing subject of Pure Language Processing (NLP), embedding fashions are important for changing sophisticated gadgets like textual content, photographs, and audio into numerical representations that computer systems can comprehend and interpret. These embeddings, that are primarily fixed-size dense vectors, kind the idea for a lot of completely different functions, similar to clustering, advice techniques, and similarity searches.
Nonetheless, as these fashions turn into extra complicated, the dimensions of the embeddings they generate additionally will increase, which causes effectivity issues for jobs that come after. To handle this, a staff of researchers has offered a brand new strategy referred to as Matryoshka Embeddings, fashions that produce helpful embeddings of assorted dimensions. Matryoshka Embeddings have been named so as a result of they resemble the Russian nesting dolls that maintain smaller dolls inside, and thus, they function on the same concept.
These fashions are made to generate embeddings that seize a very powerful data within the embedding’s preliminary dimensions, permitting them to be truncated to smaller sizes with out considerable loss in efficiency. Variable-size embeddings that may be scaled in accordance with storage necessities, processing velocity necessities, and efficiency trade-offs are made potential by this attribute.
Matryoshka Embeddings has a number of functions. For instance, decreased embeddings can be utilized to rapidly shortlist candidates earlier than doing extra computationally demanding evaluation using the whole embeddings, as within the case of closest neighbor search shortlisting. This flexibility in controlling the embeddings’ measurement with out considerably sacrificing accuracy affords an enormous profit by way of financial system and scalability
.
The staff has shared that as in comparison with typical fashions, Matryoshka Embedding fashions require a extra subtle strategy throughout coaching. The process includes evaluating the standard of embeddings at completely different decreased sizes in addition to at their full measurement. The mannequin prioritizes a very powerful data within the first dimensions with the assistance of a specialised loss operate that assesses the embeddings at a number of dimensions.
Matryoshka’s fashions are supported by frameworks similar to Sentence Transformers, which makes it comparatively straightforward to place this technique into follow. These fashions may be educated with little overhead by utilizing a Matryoshka-specific loss operate throughout varied truncations of the embeddings.
When utilizing Matryoshka Embeddings in follow, embeddings are generated usually, however they are often optionally truncated to the required measurement. This method reduces the computational load, which drastically improves the effectivity of downstream actions with out slowing down the formation of embeddings.
Two fashions educated on the AllNLI dataset have been used for instance to point out the effectiveness of Matryoshka Embeddings. First, in comparison with an everyday mannequin, the Matryoshka mannequin performs higher on numerous completely different facets. The Matryoshka mannequin maintains round 98% of its performance even when decreased to barely over 8% of its preliminary measurement, demonstrating its effectiveness and providing the opportunity of appreciable processing and storage time financial savings.
The chances of Matryoshka Embeddings have additionally been demonstrated by the staff in an interactive demo that lets customers dynamically change an embedding mannequin’s output dimensions and see the way it impacts retrieval efficiency. This sensible demonstration not solely highlights the adaptability of Matryoshka Embeddings but additionally highlights how they’ve the power to remodel the effectiveness of embedding-based functions fully.
In conclusion, Matryoshka Embeddings has helped resolve the rising drawback of sustaining the effectivity of embedding fashions as they scale in measurement and complexity. These fashions present new alternatives for optimizing NLP functions in varied domains, as they allow the dynamic scaling of embedding sizes with out important loss in accuracy.
Try the Models. All credit score for this analysis goes to the researchers of this mission. Additionally, don’t neglect to observe us on Twitter and Google News. Be a part of our 38k+ ML SubReddit, 41k+ Facebook Community, Discord Channel, and LinkedIn Group.
Should you like our work, you’ll love our newsletter..
Don’t Neglect to hitch our Telegram Channel
You might also like our FREE AI Courses….
Tanya Malhotra is a ultimate 12 months undergrad from the College of Petroleum & Power Research, Dehradun, pursuing BTech in Laptop Science Engineering with a specialization in Synthetic Intelligence and Machine Studying.
She is a Knowledge Science fanatic with good analytical and demanding considering, together with an ardent curiosity in buying new abilities, main teams, and managing work in an organized method.