Discover ways to assess the danger of AI techniques
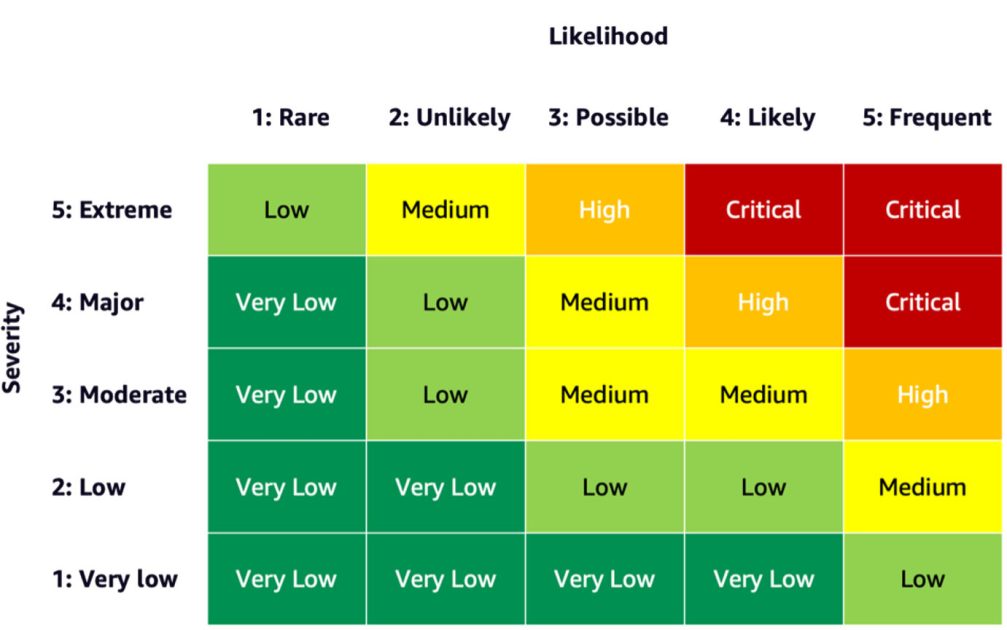
Synthetic intelligence (AI) is a quickly evolving discipline with the potential to enhance and remodel many facets of society. In 2023, the tempo of adoption of AI applied sciences has accelerated additional with the event of highly effective basis fashions (FMs) and a ensuing development in generative AI capabilities.
At Amazon, we have now launched a number of generative AI companies, equivalent to Amazon Bedrock and Amazon CodeWhisperer, and have made a spread of extremely succesful generative fashions out there by way of Amazon SageMaker JumpStart. These companies are designed to help our clients in unlocking the rising capabilities of generative AI, together with enhanced creativity, customized and dynamic content material creation, and modern design. They will additionally allow AI practitioners to make sense of the world as by no means earlier than—addressing language limitations, local weather change, accelerating scientific discoveries, and extra.
To comprehend the complete potential of generative AI, nonetheless, it’s necessary to rigorously replicate on any potential dangers. Firstly, this advantages the stakeholders of the AI system by selling accountable and protected improvement and deployment, and by encouraging the adoption of proactive measures to handle potential impression. Consequently, establishing mechanisms to evaluate and handle danger is a vital course of for AI practitioners to think about and has turn out to be a core element of many rising AI trade requirements (for instance, ISO 42001, ISO 23894, and NIST RMF) and laws (equivalent to EU AI Act).
On this submit, we talk about the right way to assess the potential danger of your AI system.
What are the completely different ranges of danger?
Whereas it is perhaps simpler to begin taking a look at a person machine studying (ML) mannequin and the related dangers in isolation, it’s necessary to think about the small print of the precise utility of such a mannequin and the corresponding use case as a part of a whole AI system. In actual fact, a typical AI system is prone to be based mostly on a number of completely different ML fashions working collectively, and a corporation is perhaps trying to construct a number of completely different AI techniques. Consequently, dangers will be evaluated for every use case and at completely different ranges, specifically mannequin danger, AI system danger, and enterprise danger.
Enterprise danger encompasses the broad spectrum of dangers that a corporation might face, together with monetary, operational, and strategic dangers. AI system danger focuses on the impression related to the implementation and operation of AI techniques, whereas ML mannequin danger pertains particularly to the vulnerabilities and uncertainties inherent in ML fashions.
On this submit, we deal with AI system danger, primarily. Nevertheless, it’s necessary to notice that every one completely different ranges of danger administration inside a corporation must be thought-about and aligned.
How is AI system danger outlined?
Danger administration within the context of an AI system could be a path to attenuate the impact of uncertainty or potential damaging impacts, whereas additionally offering alternatives to maximise optimistic impacts. Danger itself isn’t a possible hurt however the impact of uncertainty on aims. Based on the NIST Risk Management Framework (NIST RMF), danger will be estimated as a multiplicative measure of an occasion’s likelihood of occurring timed by the magnitudes of the implications of the corresponding occasion.
There are two facets to danger: inherent danger and residual danger. Inherent danger represents the quantity of danger the AI system displays in absence of mitigations or controls. Residual danger captures the remaining dangers after factoring in mitigation methods.
At all times remember that danger evaluation is a human-centric exercise that requires organization-wide efforts; these efforts vary from guaranteeing all related stakeholders are included within the evaluation course of (equivalent to product, engineering, science, gross sales, and safety groups) to assessing how social views and norms affect the perceived probability and penalties of sure occasions.
Why ought to your group care about danger analysis?
Establishing danger administration frameworks for AI techniques can profit society at massive by selling the protected and accountable design, improvement and operation of AI techniques. Danger administration frameworks may profit organizations by way of the next:
- Improved decision-making – By understanding the dangers related to AI techniques, organizations could make higher choices about the right way to mitigate these dangers and use AI techniques in a protected and accountable method
- Elevated compliance planning – A danger evaluation framework might help organizations put together for danger evaluation necessities in related legal guidelines and rules
- Constructing belief – By demonstrating that they’re taking steps to mitigate the dangers of AI techniques, organizations can present their clients and stakeholders that they’re dedicated to utilizing AI in a protected and accountable method
assess danger?
As a primary step, a corporation ought to take into account describing the AI use case that must be assessed and establish all related stakeholders. A use case is a selected situation or state of affairs that describes how customers work together with an AI system to attain a selected purpose. When making a use case description, it may be useful to specify the enterprise drawback being solved, listing the stakeholders concerned, characterize the workflow, and supply particulars concerning key inputs and outputs of the system.
On the subject of stakeholders, it’s straightforward to miss some. The next determine is an efficient place to begin to map out AI stakeholder roles.
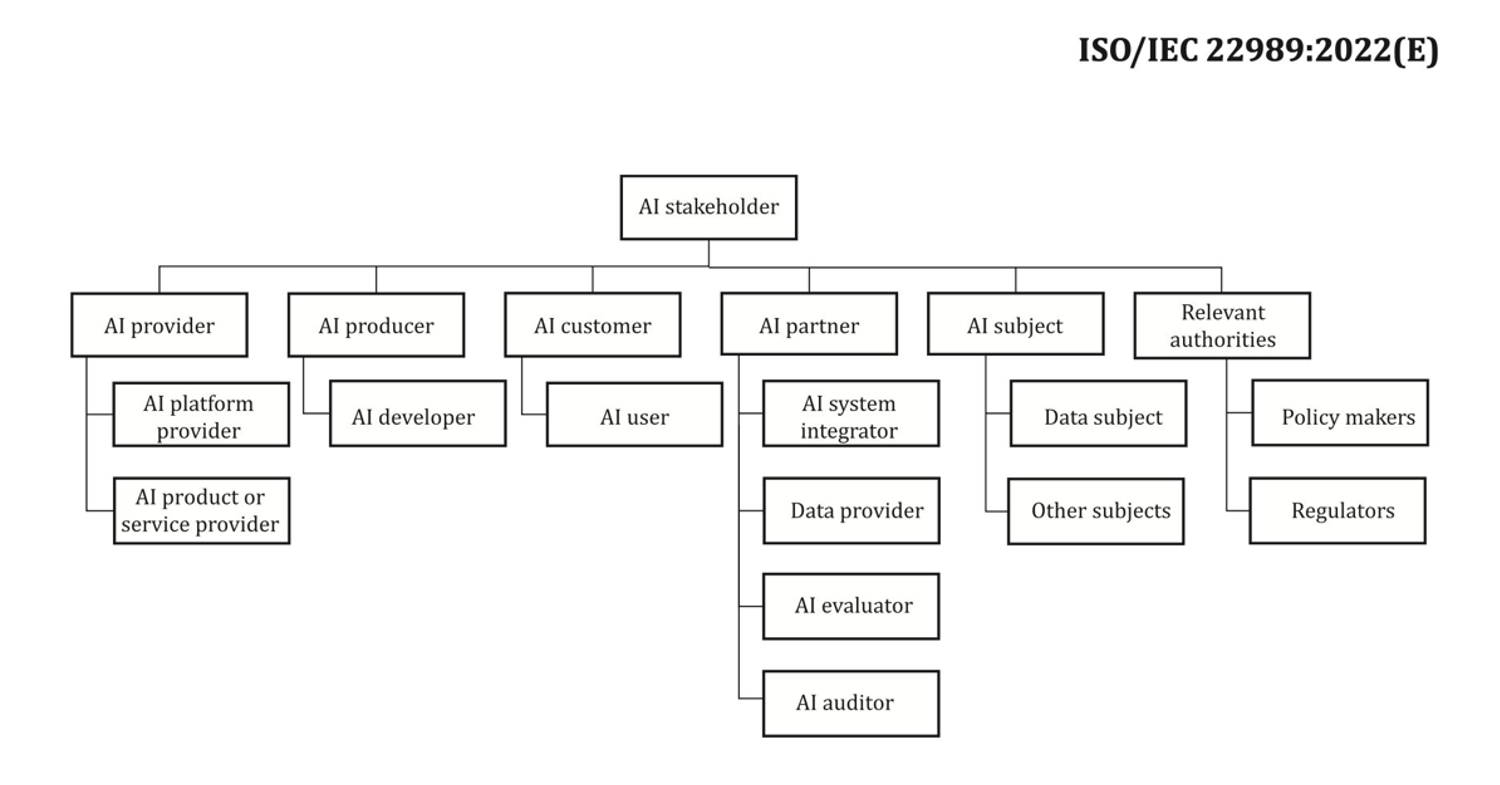
Supply: “Info expertise – Synthetic intelligence – Synthetic intelligence ideas and terminology”.
An necessary subsequent step of the AI system danger evaluation is to establish probably dangerous occasions related to the use case. In contemplating these occasions, it may be useful to replicate on completely different dimensions of accountable AI, equivalent to equity and robustness, for instance. Totally different stakeholders is perhaps affected to completely different levels alongside completely different dimensions. For instance, a low robustness danger for an end-user may very well be the results of an AI system exhibiting minor disruptions, whereas a low equity danger may very well be brought on by an AI system producing negligibly completely different outputs for various demographic teams.
To estimate the danger of an occasion, you need to use a probability scale together with a severity scale to measure the likelihood of incidence in addition to the diploma of penalties. A useful place to begin when creating these scales is perhaps the NIST RMF, which suggests utilizing qualitative nonnumerical classes starting from very low to very excessive danger or semi-quantitative assessments ideas, equivalent to scales (equivalent to 1–10), bins, or in any other case consultant numbers. After you’ve gotten outlined the probability and severity scales for all related dimensions, you need to use a danger matrix scheme to quantify the general danger per stakeholders alongside every related dimension. The next determine reveals an instance danger matrix.
Utilizing this danger matrix, we are able to take into account an occasion with low severity and uncommon probability of occurring as very low danger. Remember that the preliminary evaluation shall be an estimate of inherent danger, and danger mitigation methods might help decrease the danger ranges additional. The method can then be repeated to generate a ranking for any remaining residual danger per occasion. If there are a number of occasions recognized alongside the identical dimension, it may be useful to choose the best danger degree amongst all to create a closing evaluation abstract.
Utilizing the ultimate evaluation abstract, organizations must outline what danger ranges are acceptable for his or her AI techniques in addition to take into account related rules and insurance policies.
AWS dedication
By means of engagements with the White House and UN, amongst others, we’re dedicated to sharing our information and experience to advance the accountable and safe use of AI. Alongside these traces, Amazon’s Adam Selipsky just lately represented AWS on the AI Safety Summit with heads of state and trade leaders in attendance, additional demonstrating our dedication to collaborating on the accountable development of synthetic intelligence.
Conclusion
As AI continues to advance, danger evaluation is turning into more and more necessary and helpful for organizations trying to construct and deploy AI responsibly. By establishing a danger evaluation framework and danger mitigation plan, organizations can scale back the danger of potential AI-related incidents and earn belief with their clients, in addition to reap advantages equivalent to improved reliability, improved equity for various demographics, and extra.
Go forward and get began in your journey of creating a danger evaluation framework in your group and share your ideas within the feedback.
Additionally try an summary of generative AI dangers revealed on Amazon Science: Responsible AI in the generative era, and discover the vary of AWS companies that may help you in your danger evaluation and mitigation journey: Amazon SageMaker Clarify, Amazon SageMaker Model Monitor, AWS CloudTrail, in addition to the model governance framework.
Concerning the Authors
Mia C. Mayer is an Utilized Scientist and ML educator at AWS Machine Studying College; the place she researches and teaches security, explainability and equity of Machine Studying and AI techniques. All through her profession, Mia established a number of college outreach applications, acted as a visitor lecturer and keynote speaker, and introduced at quite a few massive studying conferences. She additionally helps inside groups and AWS clients get began on their accountable AI journey.
Denis V. Batalov is a 17-year Amazon veteran and a PhD in Machine Studying, Denis labored on such thrilling tasks as Search Contained in the Ebook, Amazon Cell apps and Kindle Direct Publishing. Since 2013 he has helped AWS clients undertake AI/ML expertise as a Options Architect. At present, Denis is a Worldwide Tech Chief for AI/ML chargeable for the functioning of AWS ML Specialist Options Architects globally. Denis is a frequent public speaker, you may comply with him on Twitter @dbatalov.
Dr. Sara Liu is a Senior Technical Program Supervisor with the AWS Accountable AI workforce. She works with a workforce of scientists, dataset leads, ML engineers, researchers, in addition to different cross-functional groups to lift the accountable AI bar throughout AWS AI companies. Her present tasks contain creating AI service playing cards, conducting danger assessments for accountable AI, creating high-quality analysis datasets, and implementing high quality applications. She additionally helps inside groups and clients meet evolving AI trade requirements.