Introducing TPU v4: Googles Slicing Edge Supercomputer for Giant Language Fashions

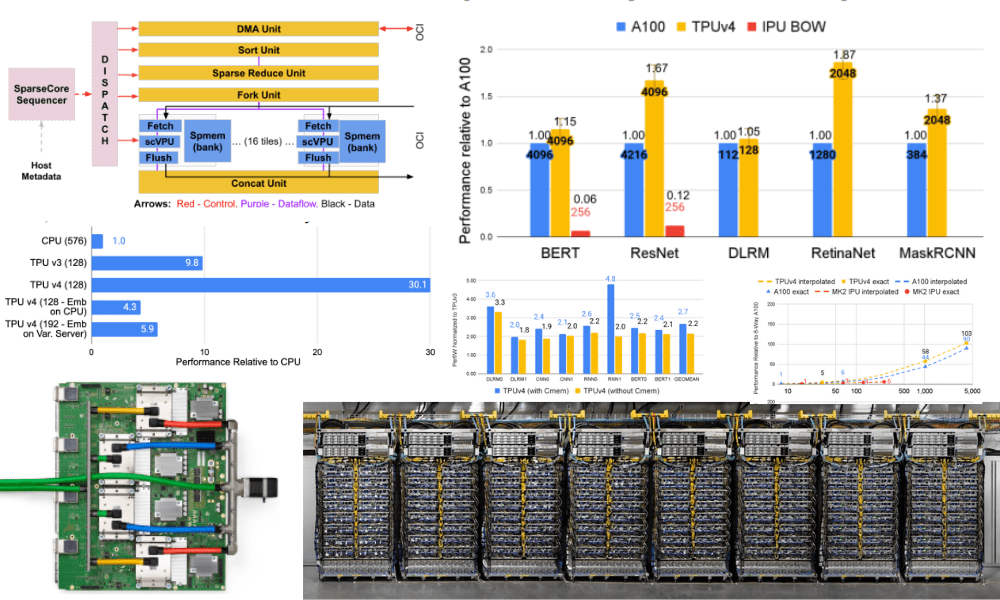
Picture by Editor
Machine studying and synthetic intelligence appear to be rising at a speedy charge that a few of us may even sustain with. As these machine-learning fashions get higher at what they do, they’ll require higher infrastructure and {hardware} help to maintain them going. The development of machine studying has a direct result in scaling computing efficiency. So let’s study extra about TPU v4.
TPU stands for Tensor Processing Unit they usually have been designed for machine studying and deep studying functions. TPU was invented by Google and was constructed in a manner that it has the power to have the ability to deal with the excessive computational wants of machine studying and synthetic intelligence.
When Google designed the TPU, they created it as a domain-specific structure, which suggests they designed it as a matrix processor, as an alternative of it being a general-purpose processor in order that it focuses on neural community workloads. This solves Google’s subject of reminiscence entry drawback which slows down GPUs and CPUs, inflicting them to make use of extra processing energy.
So there’s been TPU v2, v3, and now v4. So what’s v2 all about?
The TPU v2 chip incorporates two TensorCores, 4 MXUs, a vector unit, and a scalar unit. See the picture beneath:
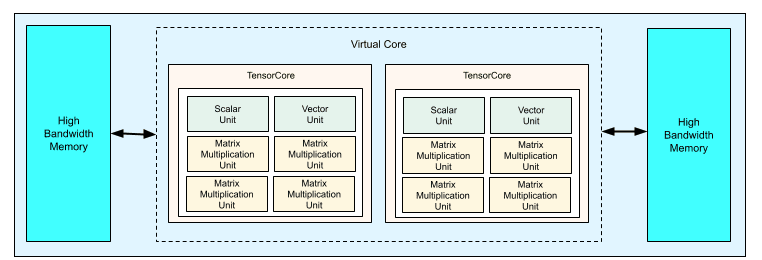
Picture by Google
Optical Circuit Switches (OCSes)
TPU v4 is the primary supercomputer to deploy reconfigurable optical circuit switches. Optical circuit switches (OCS) are thought-about to be simpler. They scale back congestion present in earlier networks as a result of they’re transmitted as they happen. OCS improves scalability, availability, modularity, deployment, safety, energy, efficiency, and extra.
OCSes and different optical elements in TPU v4 make up lower than 5% of TPU v4’s system value and fewer than 5% of system energy.
SparseCores
TPU v4 is also the primary supercomputer with {hardware} help for embedding. Neural networks prepare effectively on dense vectors, and embeddings are the simplest technique to remodel categorical function values into dense vectors. TPU v4 embody third-generation SparseCores, that are dataflow processes that speed up machine studying fashions which can be reliant on embedding.
For instance, the embedding operate can translate a phrase in English, which might be thought-about a big categorical house right into a smaller dense house of a 100-vector illustration of every phrase. Embedding is a key aspect to Deep Studying Suggestion Fashions (DLRMs), that are a part of our on a regular basis lives and are utilized in promoting, search rating, YouTube, and extra.
The picture beneath reveals the efficiency of advice fashions on CPUs, TPU v3, TPU v4 (utilizing SparseCore), and TPU v4 with embeddings in CPU reminiscence (not utilizing SparseCore). As you possibly can see the TPU v4 SparseCore is 3X sooner than TPU v3 on advice fashions, and 5–30X sooner than methods utilizing CPUs.

Picture by Google
Efficiency
TPU v4 outperforms TPU v3 by 2.1x and has an improved efficiency/Watt by 2.7x. TPU v4 is 4x bigger at 4096 chips, making it 10x sooner. The implementation and suppleness of OCS are additionally main assist for giant language fashions.
The efficiency and availability of TPU v4 supercomputers are being closely thought-about to enhance massive language fashions similar to LaMDA, MUM, and PaLM. PaLM, the 540B-parameter mannequin was skilled on TPU v4 for over 50 days and had a exceptional 57.8% {hardware} floating level efficiency.
TPU v4 additionally has multidimensional model-partitioning methods that allow low-latency, high-throughput inference for giant language fashions.
Vitality Effectivity
With extra legal guidelines and rules being put in place for firms globally to do higher to enhance their total power effectivity, TPU v4 is doing an honest job. TPU v4s inside Google Cloud use ~2-6x much less power and produce ~20x much less CO2e than modern DSAs in typical on-premise information centres.
So now you realize a bit extra about TPU v4, you is perhaps questioning how briskly machine studying workloads truly change with TPU v4.
The beneath desk reveals the workload by deep neural community mannequin sort and the % TPUs used. Over 90% of coaching at Google is on TPUs, and this desk reveals the quick change in manufacturing workloads at Google.
There’s a drop in recurrent neural networks (RNN), as it’s because RNNs course of the enter slightly than sequentially, compared to transforms that are identified for pure language translation and textual content summarization.

To study extra about TPU v4 capabilities, learn the analysis paper TPU v4: An Optically Reconfigurable Supercomputer for Machine Learning with Hardware Support for Embeddings.
Final yr, TPU v4 supercomputers have been accessible to AI researchers and builders at Google Cloud’s ML cluster in Oklahoma. The creator of this paper claims that the TPU v4 is quicker and makes use of much less energy than Nvidia A100. Nonetheless, they haven’t been capable of evaluate the TPU v4 to the newer Nvidia H100 GPUs on account of their restricted availability and its 4nm structure, whereas TPU v4 has a 7nm structure.
What do you assume TPU v4 is able to, its limitations, and is it higher than Nvidia A100 GPU?
Nisha Arya is a Information Scientist, Freelance Technical Author and Neighborhood Supervisor at KDnuggets. She is especially considering offering Information Science profession recommendation or tutorials and principle based mostly data round Information Science. She additionally needs to discover the alternative ways Synthetic Intelligence is/can profit the longevity of human life. A eager learner, in search of to broaden her tech data and writing abilities, while serving to information others.