Pure sciences – Google AI Weblog
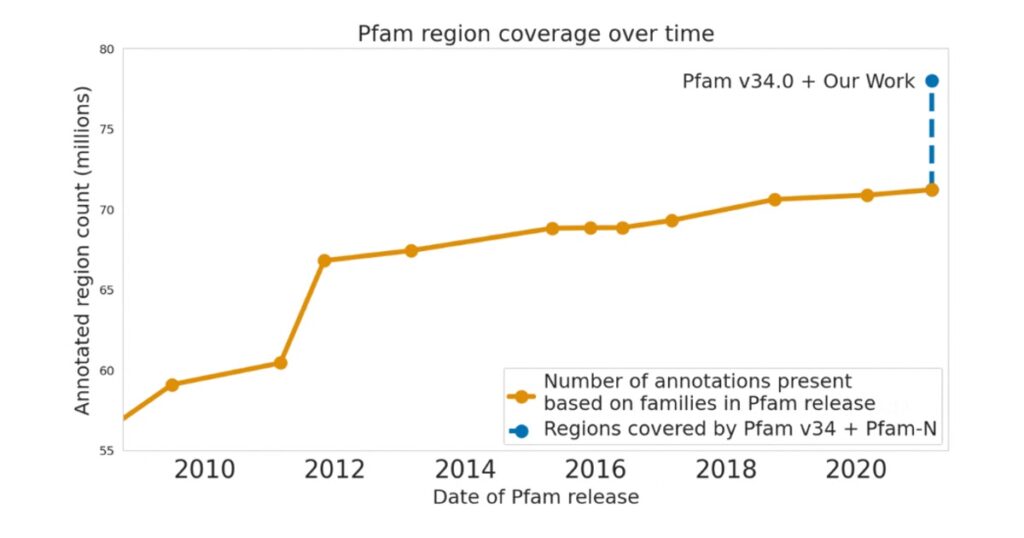
It is an extremely thrilling time to be a scientist. With the wonderful advances in machine studying (ML) and quantum computing, we now have highly effective new instruments that allow us to behave on our curiosity, collaborate in new methods, and radically speed up progress towards breakthrough scientific discoveries.
Since becoming a member of Google Analysis eight years in the past, I’ve had the privilege of being a part of a group of gifted researchers fascinated by making use of cutting-edge computing to push the boundaries of what’s doable in utilized science. Our groups are exploring subjects throughout the bodily and pure sciences. So, for this 12 months’s weblog submit I wish to concentrate on high-impact advances we’ve made just lately within the fields of biology and physics, from serving to to arrange the world’s protein and genomics data to learn individuals’s lives to enhancing our understanding of the character of the universe with quantum computer systems. We’re impressed by the good potential of this work.
Utilizing machine studying to unlock mysteries in biology
A lot of our researchers are fascinated by the extraordinary complexity of biology, from the mysteries of the mind, to the potential of proteins, and to the genome, which encodes the very language of life. We’ve been working alongside scientists from different main organizations all over the world to sort out necessary challenges within the fields of connectomics, protein function prediction, and genomics, and to make our improvements accessible and helpful to the larger scientific group.
Neurobiology
One thrilling utility of our Google-developed ML methods was to discover how data travels by means of the neuronal pathways in the brains of zebrafish, which offers perception into how the fish interact in social conduct like swarming. In collaboration with researchers from the Max Planck Institute for Biological Intelligence, we have been capable of computationally reconstruct a portion of zebrafish brains imaged with 3D electron microscopy — an thrilling advance in using imaging and computational pipelines to map out the neuronal circuitry in small brains, and one other step ahead in our long-standing contributions to the sector of connectomics.
![]() |
Reconstruction of the neural circuitry of a larval zebrafish mind, courtesy of the Max Planck Institute for Organic Intelligence. |
The technical advances obligatory for this work could have functions even past neuroscience. For instance, to handle the problem of working with such massive connectomics datasets, we developed and launched TensorStore, an open-source C++ and Python software library designed for storage and manipulation of n-dimensional information. We sit up for seeing the methods it’s utilized in different fields for the storage of enormous datasets.
We’re additionally utilizing ML to make clear how human brains carry out outstanding feats like language by comparing human language processing and autoregressive deep language models (DLMs). For this examine, a collaboration with colleagues at Princeton University and New York University Grossman School of Medicine, members listened to a 30-minute podcast whereas their mind exercise was recorded utilizing electrocorticography. The recordings recommended that the human mind and DLMs share computational rules for processing language, together with steady next-word prediction, reliance on contextual embeddings, and calculation of post-onset shock primarily based on phrase match (we will measure how stunned the human mind is by the phrase, and correlate that shock sign with how effectively the phrase is predicted by the DLM). These outcomes present new insights into language processing within the human mind, and counsel that DLMs can be utilized to disclose useful insights concerning the neural foundation of language.
Biochemistry
ML has additionally allowed us to make vital advances in understanding organic sequences. In 2022, we leveraged current advances in deep studying to accurately predict protein function from uncooked amino acid sequences. We additionally labored in shut collaboration with the European Molecular Biology Laboratory’s European Bioinformatics Institute (EMBL-EBI) to rigorously assess model performance and add hundreds of millions of functional annotations to the general public protein databases UniProt, Pfam/InterPro, and MGnify. Human annotation of protein databases is usually a laborious and gradual course of and our ML strategies enabled an enormous leap ahead — for instance, rising the variety of Pfam annotations by a bigger quantity than all different efforts throughout the previous decade mixed. The hundreds of thousands of scientists worldwide who entry these databases every year can now use our annotations for his or her analysis.
![]() |
Google Analysis contributions to Pfam exceed in dimension all enlargement efforts made to the database over the past decade. |
Though the primary draft of the human genome was launched in 2003, it was incomplete and had many gaps as a consequence of technical limitations within the sequencing applied sciences. In 2022 we celebrated the outstanding achievements of the Telomere-2-Telomere (T2T) Consortium in resolving these beforehand unavailable areas — together with 5 full chromosome arms and almost 200 million base pairs of novel DNA sequences — that are fascinating and necessary for questions of human biology, evolution, and illness. Our open source genomics variant caller, DeepVariant, was one of the tools utilized by the T2T Consortium to organize their launch of a complete 3.055 billion base pair sequence of a human genome. The T2T Consortium can be utilizing our newer open source methodology DeepConsensus, which offers on-device error correction for Pacific Biosciences long-read sequencing devices, of their latest research towards complete pan-genome sources that may signify the breadth of human genetic range.
Utilizing quantum computing for brand spanking new physics discoveries
With regards to making scientific discoveries, quantum computing remains to be in its infancy, however has quite a lot of potential. We’re exploring methods of advancing the capabilities of quantum computing in order that it could turn out to be a device for scientific discovery and breakthroughs. In collaboration with physicists from all over the world, we’re additionally beginning to use our present quantum computer systems to create fascinating new experiments in physics.
For instance of such experiments, take into account the issue the place a sensor measures one thing, and a pc then processes the information from the sensor. Historically, this implies the sensor’s information is processed as classical data on our computer systems. As an alternative, one thought in quantum computing is to straight course of quantum information from sensors. Feeding information from quantum sensors on to quantum algorithms with out going by means of classical measurements might present a big benefit. In a recent Science paper written in collaboration with researchers from a number of universities, we present that quantum computing can extract data from exponentially fewer experiments than classical computing, so long as the quantum pc is coupled on to the quantum sensors and is working a studying algorithm. This “quantum machine learning” can yield an exponential benefit in dataset dimension, even with immediately’s noisy intermediate-scale quantum computer systems. As a result of experimental information is usually the limiting think about scientific discovery, quantum ML has the potential to unlock the huge energy of quantum computer systems for scientists. Even higher, the insights from this work are additionally relevant to studying on the output of quantum computations, such because the output of quantum simulations that will in any other case be troublesome to extract.
Even with out quantum ML, a robust utility of quantum computer systems is to experimentally discover quantum programs that might be in any other case inconceivable to look at or simulate. In 2022, the Quantum AI staff used this method to look at the first experimental evidence of multiple microwave photons in a bound state using superconducting qubits. Photons sometimes don’t work together with each other, and require an extra ingredient of non-linearity to trigger them to work together. The outcomes of our quantum computer simulations of these interactions stunned us — we thought the existence of those certain states relied on fragile circumstances, however as an alternative we discovered that they have been sturdy even to comparatively robust perturbations that we utilized.
![]() |
Occupation chance versus discrete time step for n-photon certain states. We observe that almost all of the photons (darker colours) stay certain collectively. |
Given the preliminary successes now we have had in making use of quantum computing to make physics breakthroughs, we’re hopeful about the opportunity of this know-how to allow future groundbreaking discoveries that might have as vital a societal impression because the creation of transistors or GPS. The way forward for quantum computing as a scientific device is thrilling!
Acknowledgements
I wish to thank everybody who labored arduous on the advances described on this submit, together with the Google Utilized Sciences, Quantum AI, Genomics and Mind groups and their collaborators throughout Google Analysis and externally. Lastly, I wish to thank the numerous Googlers who offered suggestions within the writing of this submit, together with Lizzie Dorfman, Erica Model, Elise Kleeman, Abe Asfaw, Viren Jain, Lucy Colwell, Andrew Carroll, Ariel Goldstein and Charina Chou.
Google Analysis, 2022 & past
This was the seventh weblog submit within the “Google Analysis, 2022 & Past” sequence. Different posts on this sequence are listed within the desk beneath: