OLAPH: A Easy and Novel AI Framework that Allows the Enchancment of Factuality by Automated Evaluations
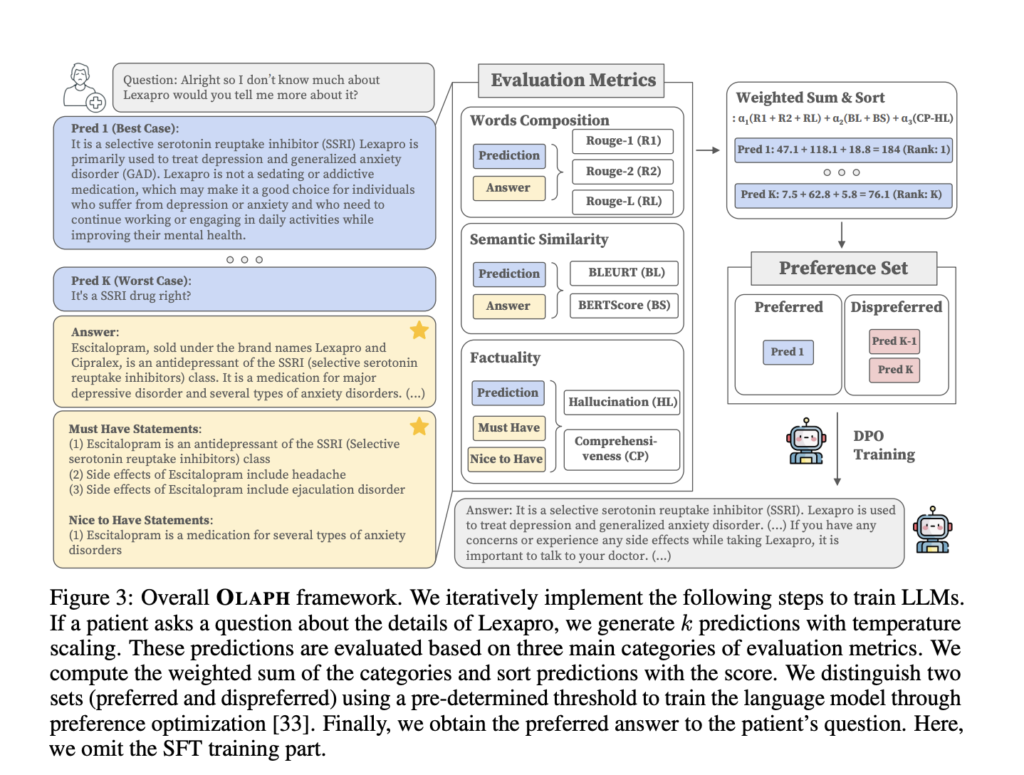
Massive Language Fashions (LLMs) are getting into scientific and medical fields as they develop in functionality and flexibility. These fashions have an a variety of benefits, together with the capability to complement and even exchange the work that docs usually do. This embrace offering medical info, protecting monitor of affected person info, and holding consultations with sufferers.
Within the medical occupation, one of many fundamental benefits of LLMs is their capability to provide long-form textual content, which is important for giving thorough responses to affected person inquiries. Responses which might be correct and instructive are important, notably in medical conditions when offering false info might need detrimental results. As an illustration, when a affected person asks in regards to the origins of a white tongue, the LLM should reply in truth about potential causes, together with bacterial accumulation, with out spreading myths, comparable to the concept the situation is invariably harmful and irreversible.
Within the medical space, there are quite a few situations through which producing complete, prolonged solutions is important. That is notably essential when answering inquiries from sufferers, as the small print given should be true and factual. To make sure the accuracy and consistency of those solutions, an automatic course of for assessing the assertions made by LLMs is required.
To dive into this, in a current examine, a staff of researchers has produced MedLFQA, a specialised benchmark dataset derived from pre-existing long-form question-answering datasets within the biomedical space. The objective of MedLFQA is to make it simpler to mechanically assess the factual accuracy of responses produced by LLMs. This dataset helps in figuring out the accuracy and dependability of the details supplied in these prolonged responses.
The staff has supplied a singular framework referred to as OLAPH (Optimizing Massive language fashions’ Solutions with Preferences of decreasing Hallucination). OLAPH makes use of a sequence of automated assessments to enhance the factual accuracy of LLMs. The methodology makes use of an iterative coaching course of to show the LLM to favor responses with the best factual and evaluation metrics scores.
For every query, the OLAPH framework generates a number of response samples. Then, utilizing predetermined evaluation standards, the response with the best rating is chosen. The LLM is then additional skilled utilizing this most popular response, bringing its subsequent responses nearer to the right and most popular solutions. The mannequin would in any other case produce false info, however this iterative strategy helps to restrict the problem of hallucinations.
The outcomes have proven appreciable enhancements in factual accuracy for LLMs skilled with the OLAPH framework, even when measured towards measures not expressly included within the coaching process. A 7-billion parameter LLM skilled with OLAPH produced long-form responses on par with skilled medical responses by way of high quality.
The staff has summarized their major contributions as follows.
- The staff has launched MedLFQA, a reorganized benchmark dataset for automated evaluation of the long-text era produced by LLMs within the biomedical discipline.
- With a purpose to consider the veracity of medical claims offered in long-form responses, the staff has developed two distinct statements that supply a complete image of the LLMs’ capability to provide correct information.
- OLAPH framework has been launched, which boosts LLM replies by iterative studying and computerized analysis.
- It has been demonstrated that LLMs with 7 billion parameters when skilled utilizing the OLAPH framework, can produce long-form solutions which might be comparable in factual accuracy to these offered by medical consultants.
In conclusion, this examine proposes the OLAPH structure to boost long-form medical responses by iterative coaching, and it introduces MedLFQA as a baseline for assessing the factual accuracy of those responses produced by LLMs. The findings present that OLAPH has the potential to drastically enhance LLMs’ dependability in producing correct medical info, which might be essential for numerous medical purposes.
Take a look at the Paper and Github. All credit score for this analysis goes to the researchers of this challenge. Additionally, don’t neglect to comply with us on Twitter. Be a part of our Telegram Channel, Discord Channel, and LinkedIn Group.
Should you like our work, you’ll love our newsletter..
Don’t Overlook to affix our 42k+ ML SubReddit
Tanya Malhotra is a last yr undergrad from the College of Petroleum & Vitality Research, Dehradun, pursuing BTech in Pc Science Engineering with a specialization in Synthetic Intelligence and Machine Studying.
She is a Information Science fanatic with good analytical and significant considering, together with an ardent curiosity in buying new abilities, main teams, and managing work in an organized method.