Remodeling Multi-Dimensional Information Processing with MambaMixer: A Leap In direction of Environment friendly and Scalable Machine Studying Fashions
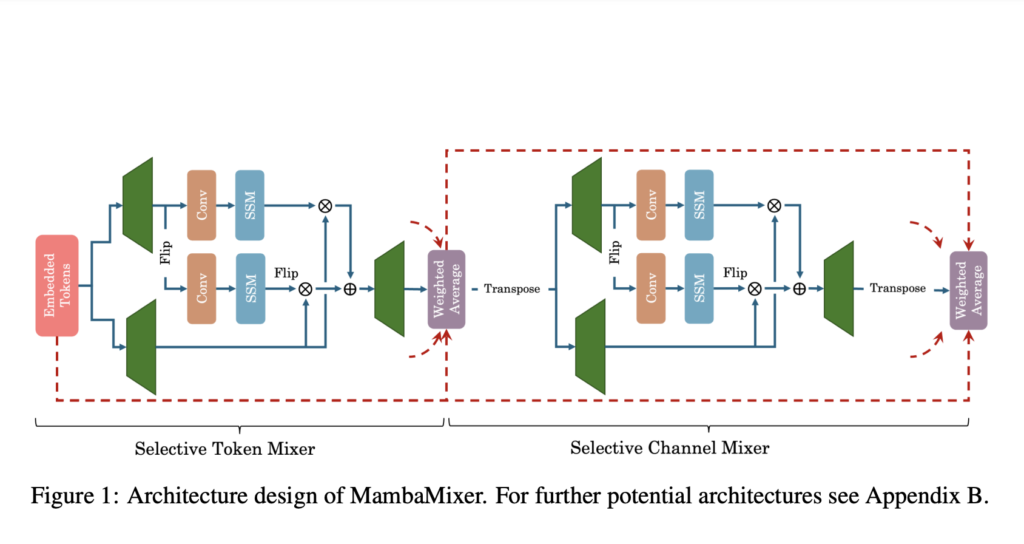
The seek for fashions that may effectively course of multidimensional information, starting from photos to complicated time collection, has turn into more and more essential. Earlier Transformer fashions, famend for his or her capacity to deal with varied duties, usually battle with lengthy sequences resulting from their quadratic computational complexity. This limitation has sparked a surge of curiosity in creating architectures that scale higher and improve efficiency when coping with large-scale datasets.
The effectivity of dealing with lengthy information sequences is pivotal, particularly as the quantity and complexity of knowledge in purposes equivalent to picture processing and time collection forecasting proceed to develop. The computational calls for of current strategies pose vital challenges, pushing researchers to innovate architectures that streamline processing with out sacrificing accuracy. Selective State Area Fashions (S6) have emerged as a promising resolution, selectively focusing computational assets on essentially the most informative information segments, probably revolutionizing the effectivity and effectiveness of knowledge processing.
Researchers from Cornell College and the NYU Grossman Faculty of Medication current MambaMixer, a novel structure that includes data-dependent weights. This structure leverages a singular twin choice mechanism, the Selective Token and Channel Mixer, to effectively navigate tokens and channels. A weighted averaging course of additional augments this twin choice mechanism to make sure seamless data stream throughout the mannequin’s layers for optimizing processing effectivity and mannequin efficiency.
The utility and effectiveness of the MambaMixer structure are exemplified in its specialised purposes: the Imaginative and prescient MambaMixer (ViM2) for image-related duties and the Time Collection MambaMixer (TSM2) for forecasting time collection information. These implementations spotlight the structure’s versatility and energy. As an illustration, in difficult benchmarks like ImageNet, ViM2 achieves aggressive efficiency in opposition to well-established fashions. Nonetheless, it surpasses SSM-based imaginative and prescient fashions, demonstrating superior effectivity and accuracy in picture classification, object detection, and semantic segmentation duties.
ViM2 has demonstrated aggressive efficiency in difficult benchmarks like ImageNet. It achieved top-1 classification accuracies of 82.7%, 83.7%, and 83.9% for its Tiny, Small, and Base variants, respectively, outperforming well-established fashions like ViT, MLP-Mixer, and ConvMixer in sure configurations. A weighted averaging mechanism enhances the knowledge stream and captures the complicated dynamics of options, contributing to its state-of-the-art efficiency. TSM2 showcases groundbreaking leads to time collection forecasting, setting new data in varied benchmarks. As an illustration, its software to the M5 dataset demonstrates an enchancment in WRMSSE scores.
The structure’s achievements, for example, in semantic segmentation duties on the ADE20K dataset, ViM2 fashions confirmed mIoU (single-scale) enhancements of 1.3, 3.7, and 4.2 for the Tiny, Small, and Medium configurations, respectively, when in comparison with different main fashions. These outcomes underscore the structure’s capability to course of data selectively and effectively.

In conclusion, as datasets proceed to develop in dimension and complexity, the event of fashions like MambaMixer, which might effectively and selectively course of data, turns into more and more important. This structure represents a vital step ahead, providing a scalable and efficient framework for tackling the challenges of contemporary machine-learning duties. Its success in each imaginative and prescient and time collection modeling duties demonstrates its potential and conjures up additional analysis and growth in environment friendly information processing strategies.
Try the Paper. All credit score for this analysis goes to the researchers of this undertaking. Additionally, don’t overlook to observe us on Twitter. Be a part of our Telegram Channel, Discord Channel, and LinkedIn Group.
In case you like our work, you’ll love our newsletter..
Don’t Overlook to affix our 39k+ ML SubReddit
Whats up, My identify is Adnan Hassan. I’m a consulting intern at Marktechpost and shortly to be a administration trainee at American Categorical. I’m at present pursuing a twin diploma on the Indian Institute of Expertise, Kharagpur. I’m obsessed with expertise and need to create new merchandise that make a distinction.