Apple Researchers Introduce Matryoshka Diffusion Fashions(MDM): An Finish-to-Finish Synthetic Intelligence Framework for Excessive-Decision Picture and Video Synthesis
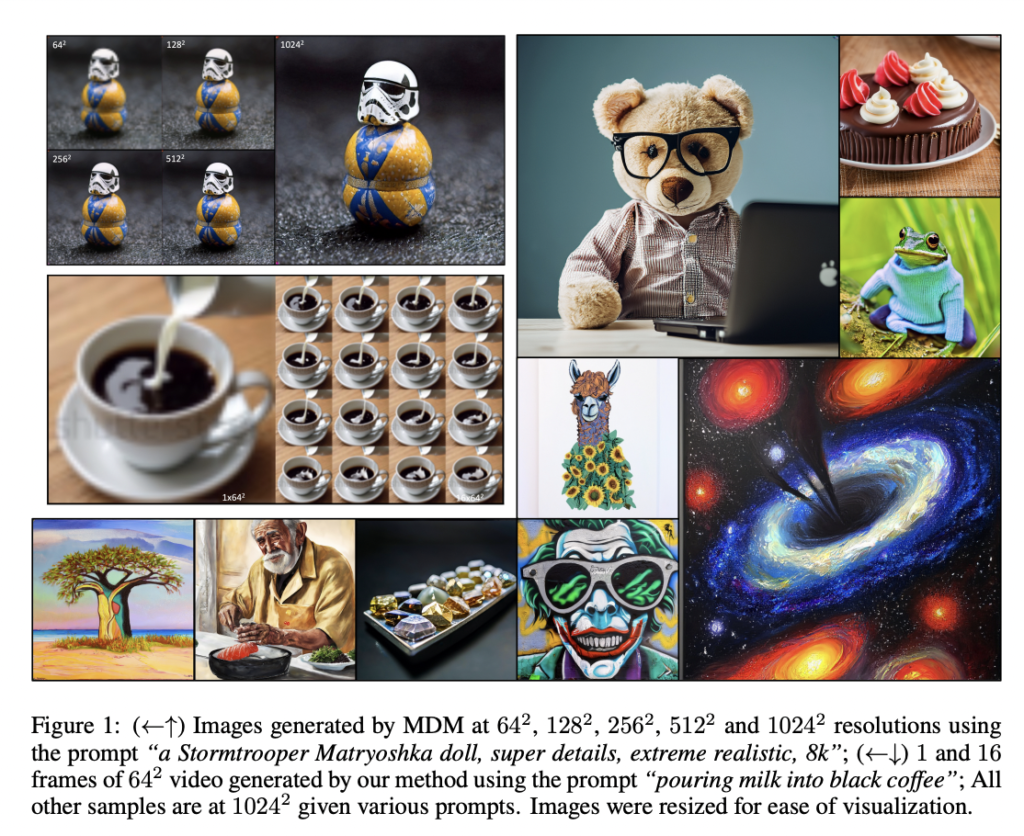
Giant Language Fashions have proven wonderful capabilities in latest instances. Diffusion fashions, specifically, have been broadly utilized in numerous generative functions, from 3D modelling and textual content technology to picture and video technology. Although these fashions cater to numerous duties, they encounter important difficulties when coping with high-resolution information. It takes plenty of processing energy and reminiscence to scale them to excessive decision since every step necessitates re-encoding the entire high-resolution enter.
Deep architectures with consideration blocks are often employed to beat these points, though they enhance computational and reminiscence calls for and complicate optimisation. Researchers have been placing in efforts to develop efficient community designs for high-resolution images. The present approaches fall in need of customary methods like DALL-E 2 and IMAGEN by way of output high quality and haven’t demonstrated aggressive outcomes past 512×512 decision.
These broadly used methods cut back computation by fusing many independently educated super-resolution diffusion fashions with a low-resolution mannequin. Conversely, latent diffusion strategies (LDMs) depend on a high-resolution autoencoder that has been individually educated, and so they solely practice low-resolution diffusion fashions. Each methods necessitate using multi-stage pipelines and meticulous hyperparameter optimisation.
In latest analysis, a workforce of researchers from Apple has launched Matryoshka Diffusion Fashions (MDM), a household of diffusion fashions which were designed for end-to-end high-resolution picture and video synthesis. MDM works on the concept of together with the low-resolution diffusion course of as a vital part of high-resolution technology. This method has been impressed by Generative Adversarial Networks (GANs) multi-scale studying, and the workforce has achieved this by using a Nested UNet structure to hold out a mixed diffusion course of throughout a number of resolutions.
Among the main elements of this method are as follows.
- Multi-Decision Diffusion Course of: MDM features a diffusion course of that denoises inputs at a number of resolutions without delay, which means that it could actually concurrently course of and produce photos with totally different ranges of element. For this, MDM makes use of a Nested UNet structure.
- NestedUNet Structure: Smaller scale enter options and parameters are nested inside bigger scale enter options and parameters within the Nested UNet structure. With this nesting, data may be shared successfully throughout scales, bettering the mannequin’s capability to seize effective options whereas preserving computational effectivity.
- Progressive Coaching Plan: MDM presents a coaching plan that progresses regularly to greater resolutions, starting at a lesser decision. Through the use of this coaching methodology, the optimisation course of is enhanced, and the mannequin is healthier in a position to discover ways to produce high-resolution content material.
The workforce has shared the efficiency and efficacy of this method by way of numerous benchmark checks, corresponding to text-to-video functions, high-resolution text-to-image manufacturing, and class-conditioned image technology. MDM has demonstrated that it could actually practice a single pixel-space mannequin at as much as 1024 × 1024 pixel decision. Contemplating that this accomplishment was made utilizing a relatively small dataset (CC12M), which consists of simply 12 million images, this can be very exceptional. MDM reveals strong zero-shot generalisation, which permits it to provide high-quality data for resolutions that it hasn’t been particularly educated on. In conclusion, Matryoshka Diffusion Fashions (MDM) represents an unbelievable step ahead within the realm of high-resolution picture and video synthesis.
Try the Paper. All Credit score For This Analysis Goes To the Researchers on This Venture. Additionally, don’t overlook to affix our 32k+ ML SubReddit, 40k+ Facebook Community, Discord Channel, and Email Newsletter, the place we share the newest AI analysis information, cool AI tasks, and extra.
If you like our work, you will love our newsletter..
We’re additionally on Telegram and WhatsApp.
Tanya Malhotra is a closing 12 months undergrad from the College of Petroleum & Vitality Research, Dehradun, pursuing BTech in Laptop Science Engineering with a specialization in Synthetic Intelligence and Machine Studying.
She is a Knowledge Science fanatic with good analytical and significant considering, together with an ardent curiosity in buying new abilities, main teams, and managing work in an organized method.