Researchers from NVIDIA Introduce Retro 48B: The Largest LLM Pretrained with Retrieval earlier than Instruction Tuning
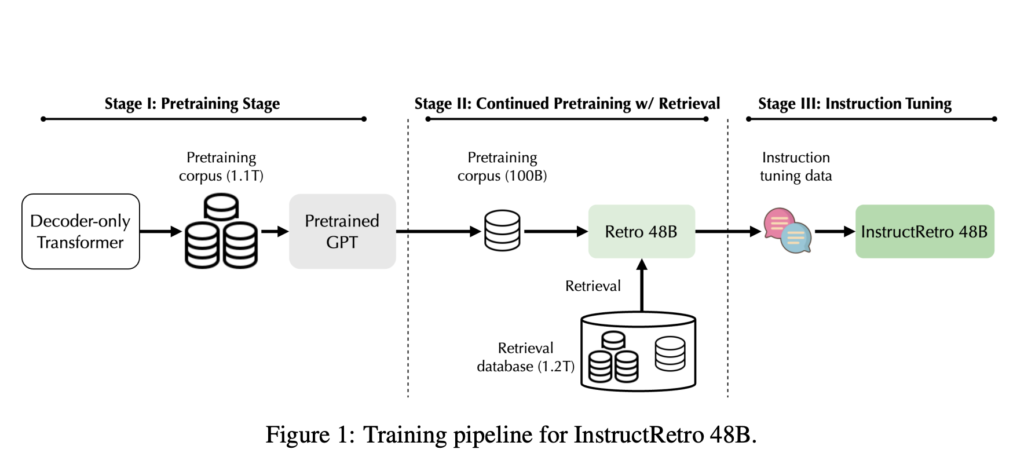
Researchers from Nvidia and the College of Illinois at Urbana Champaign introduce Retro 48B, a considerably bigger language mannequin than earlier retrieval-augmented fashions like Retro (7.5B parameters). Retro 48B is pre-trained with retrieval on an in depth corpus, resulting in improved perplexity. The encoder in InstructRetro could be ablated, suggesting that continued retrieval-augmented pre-training enhances the decoder’s efficiency in query answering.
Retrieval-augmented language fashions are well-established for open-domain query answering, benefiting each throughout pre-training and inference. Their strategy reduces mannequin perplexity, improves factuality, and enhances process efficiency post-fine-tuning. Present retrieval-augmented fashions are constrained in measurement in comparison with decoder-only fashions, limiting their zero-shot generalization potential after instruction tuning. Instruction tuning, very important for pure language understanding, has gained help from high-quality datasets like FLAN, OpenAssistant, and Dolly, enabling superior efficiency in chat and question-answering duties.
Pretraining language fashions with retrieval, akin to Retro, has proven promise in lowering perplexity and enhancing factual accuracy. Nevertheless, current retrieval-augmented fashions want extra parameters and coaching knowledge, impacting their efficiency in instruction tuning and different duties typical of huge language fashions. Their research introduces Retro 48B, the biggest retrieval-augmented mannequin, persevering with to pretrain a 43B GPT mannequin with further tokens. InstructRetro, obtained from this course of, considerably improves zero-shot query answering in comparison with conventional GPT fashions. InstructRetro’s decoder achieves comparable outcomes when the encoder is ablated, demonstrating the retrieval-augmented pre-training’s effectiveness in context incorporation for query answering.
Their research explores an in depth course of involving pretraining a GPT mannequin to create Retro 48B, instructing it to boost its zero-shot question-answering talents, and evaluating its efficiency in varied duties. It introduces a novel 48B-sized retrieval-augmented language mannequin, InstructRetro, which considerably outperforms the usual GPT mannequin in zero-shot question-answering duties after instruction tuning. This scaling-up strategy demonstrates the potential of bigger retrieval-augmented fashions in pure language understanding.
Retro 48B, a language mannequin pre-trained with retrieval, surpasses the unique GPT mannequin in perplexity. After instruction tuning, known as InstructRetro, it considerably enhances zero-shot query answering, with a median enchancment of seven% on short-form and 10% on long-form QA duties in comparison with its GPT counterpart. Surprisingly, InstructRetro’s decoder spine alone delivers comparable outcomes, indicating the effectiveness of retrieval-based pretraining in context incorporation for QA.
Introducing InstructRetro 48B, the biggest retrieval-augmented language mannequin, considerably enhances zero-shot accuracy in a variety of open-ended QA duties in comparison with its GPT counterpart. Pretraining with retrieval utilizing the Retro augmentation methodology improved perplexity. Their research’s outcomes recommend that continued pre-training with restoration earlier than instruction tuning affords a promising route for enhancing GPT decoders in QA. Surprisingly, the decoder achieves comparable accuracy, showcasing the effectiveness of pretraining for context incorporation. InstructRetro excels in long-form QA duties, highlighting retrieval-augmented pretraining’s potential for difficult duties.
Try the Paper. All Credit score For This Analysis Goes To the Researchers on This Mission. Additionally, don’t overlook to hitch our 31k+ ML SubReddit, 40k+ Facebook Community, Discord Channel, and Email Newsletter, the place we share the newest AI analysis information, cool AI initiatives, and extra.
If you like our work, you will love our newsletter..
We’re additionally on WhatsApp. Join our AI Channel on Whatsapp..
Whats up, My identify is Adnan Hassan. I’m a consulting intern at Marktechpost and shortly to be a administration trainee at American Categorical. I’m at the moment pursuing a twin diploma on the Indian Institute of Expertise, Kharagpur. I’m obsessed with expertise and wish to create new merchandise that make a distinction.