Apple and CMU Researchers Unveil the Unending UI Learner: Revolutionizing App Accessibility By way of Steady Machine Studying
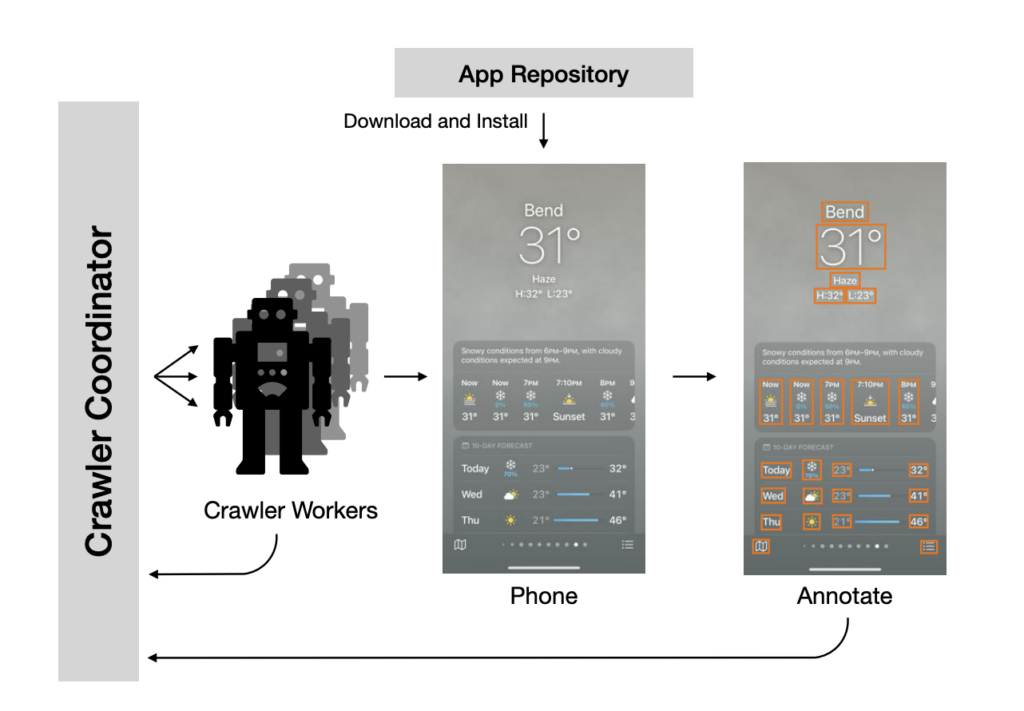
Machine studying is turning into more and more built-in throughout a variety of fields. Its widespread use extends to all industries, together with the world of person interfaces (UIs), the place it’s essential for anticipating semantic information. This software not solely improves accessibility and simplifies testing but in addition helps automate UI-related duties, leading to extra streamlined and efficient functions.
At the moment, many fashions primarily depend on datasets of static screenshots that people have rated. However this strategy is pricey and exposes unanticipated inclinations towards errors in some actions. As a result of they can’t work together with the UI factor within the dwell app to verify their conclusions, human annotators should rely solely on visible clues when evaluating if a UI factor is tappable from a snapshot.
Regardless of the drawbacks of utilizing datasets that solely file fastened snapshots of cell software views, they’re costly to make use of and preserve. Nevertheless, because of their abundance of information, these datasets proceed to be invaluable for coaching Deep Neural Networks (DNNs).
Consequently, Apple researchers have developed the By no means-Ending UI Learner AI system in collaboration with Carnegie Mellon College. This method interacts frequently with precise cell functions, permitting it to repeatedly enhance its understanding of UI design patterns and new tendencies. It autonomously downloads apps from app shops for cell units and totally investigates every one to seek out recent and tough coaching situations.
The By no means-Ending UI Learner has explored over 5,000 gadget hours to date, performing greater than 500,000 actions throughout 6,000 apps. Because of this extended interplay, three completely different pc imaginative and prescient fashions will likely be skilled: one for predicting tappability, one other for predicting draggability, and a 3rd for figuring out display screen similarity.
It performs quite a few interactions, resembling faucets and swipes, on elements contained in the person interface of every app throughout this analysis. The researchers emphasize that it classifies UI components utilizing designed heuristics, figuring out traits like whether or not a button could also be touched or a picture will be moved.
With the assistance of the collected information, fashions that forecast the tappability and draggability of UI components and the similarity of seen screens are skilled. The top-to-end process doesn’t require any extra human-labeled examples, even when the method can start with a mannequin skilled on human-labeled information.
The researchers emphasised that this technique of actively investigating apps has a profit. It assists the machine in figuring out difficult circumstances that typical human-labeled datasets may overlook. Sometimes, individuals might not discover the whole lot that may be touched on a display screen as a result of the pictures aren’t all the time very clear. Nevertheless, the crawler can faucet on gadgets and instantly watch what occurs, offering clearer and higher data.
The researchers demonstrated how fashions skilled on this information enhance over time, with tappability prediction reaching 86% accuracy after 5 coaching rounds.
The researchers highlighted that functions targeted on accessibility repairs may profit from extra frequent updates to catch delicate adjustments. On the flip facet, longer intervals permitting the buildup of extra important UI adjustments could possibly be preferable for duties like summarizing or mining design patterns. Determining the perfect schedules for retraining and updates would require additional analysis.
This work emphasizes the potential of endless studying, enabling programs to adapt and advance as they absorb extra information repeatedly. Whereas the present system focuses on modeling easy semantics like tappability, Apple hopes to use comparable rules to study extra subtle representations of cell UIs and interplay patterns.
Try the Paper. All Credit score For This Analysis Goes To the Researchers on This Challenge. Additionally, don’t neglect to affix our 31k+ ML SubReddit, 40k+ Facebook Community, Discord Channel, and Email Newsletter, the place we share the most recent AI analysis information, cool AI initiatives, and extra.
If you like our work, you will love our newsletter..
We’re additionally on WhatsApp. Join our AI Channel on Whatsapp..