Can Low-Value Quadrupedal Robots Grasp Parkour? Unveiling a Revolutionary Studying System for Agile Robotic Motion
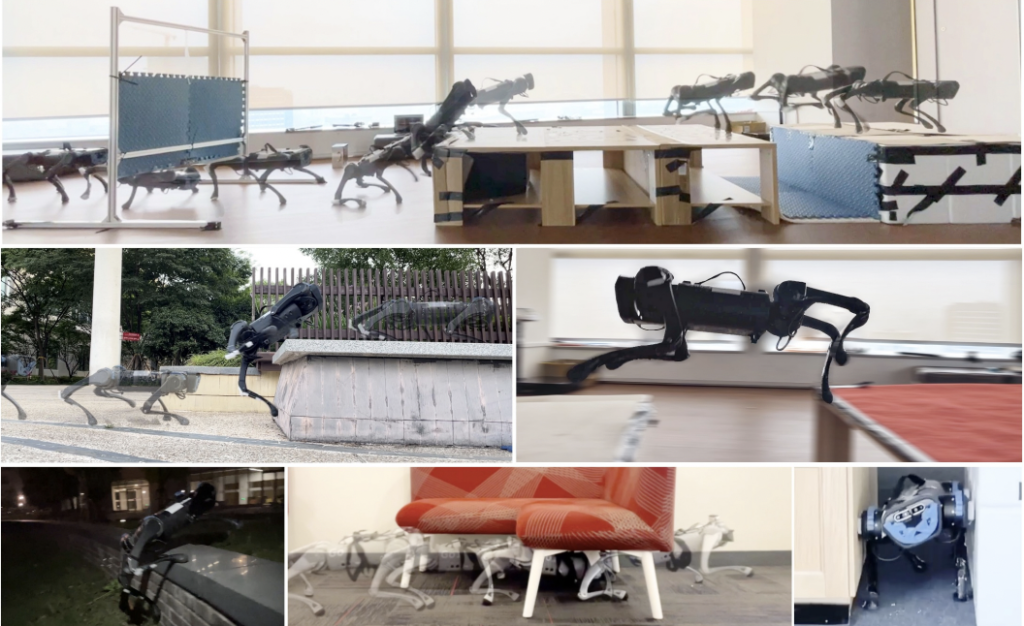
The hunt to make robots carry out advanced bodily duties, equivalent to navigating difficult environments, has been a long-standing problem in robotics. Some of the demanding duties on this area is parkour, a sport that includes traversing obstacles with pace and agility. Parkour requires a mix of expertise, together with climbing, leaping, crawling, and tilting, which is especially difficult for robots as a result of want for exact coordination, notion, and decision-making. The first downside this paper and article goal to handle is tips on how to effectively educate robots these agile parkour expertise, enabling them to navigate by means of various real-world eventualities.
Earlier than delving into the proposed answer, it’s important to grasp the present cutting-edge in robotic locomotion. Conventional strategies usually contain manually designing management methods, which may be extremely labor-intensive and want extra adaptability to completely different eventualities. Reinforcement studying (RL) has proven promise in instructing robots advanced duties. Nevertheless, RL strategies face challenges associated to exploration and transferring realized expertise from simulation to the actual world.
Now, let’s discover the progressive method launched by a analysis staff to sort out these challenges. The researchers have developed a two-stage RL technique designed to successfully educate parkour expertise to robots. The distinctiveness of their method lies in integrating “smooth dynamics constraints” throughout the preliminary coaching part, which is essential for environment friendly talent acquisition.
The researchers’ method includes a number of key parts contributing to its effectiveness.
1. Specialised Talent Insurance policies: The tactic’s basis includes developing specialised talent insurance policies important for parkour. These insurance policies are created utilizing a mix of recurrent neural networks (GRU) and multilayer perceptrons (MLP) that output joint positions. They think about numerous sensory inputs, together with depth pictures, proprioception (consciousness of the physique’s place), earlier actions, and extra. This mix of inputs permits robots to make knowledgeable selections based mostly on their setting.
2. Comfortable Dynamics Constraints: The method’s progressive side is utilizing “smooth dynamics constraints” throughout the preliminary coaching part. These constraints information the educational course of by offering robots with vital details about their setting. By introducing smooth dynamics constraints, the researchers be sure that robots can discover and study parkour expertise effectively. This leads to sooner studying and improved efficiency.
3. Simulated Environments: The researchers make use of simulated environments created with IsaacGym to coach the specialised talent insurance policies. These environments include 40 tracks, every containing 20 obstacles of various difficulties. The obstacles’ properties, equivalent to top, width, and depth, enhance linearly in complexity throughout the tracks. This setup permits robots to study progressively difficult parkour expertise.
4. Reward Buildings: Reward constructions are essential in reinforcement studying. The researchers meticulously outline reward phrases for every specialised talent coverage. These reward phrases align with particular goals, equivalent to velocity, power conservation, penetration depth, and penetration quantity. The reward constructions are fastidiously designed to incentivize and discourage undesirable behaviors.
5. Area Adaptation: Transferring expertise realized in simulation to the actual world is a considerable problem in robotics. The researchers make use of area adaptation strategies to bridge this hole. Robots can apply their parkour talents in sensible settings by adapting the talents acquired in simulated environments to real-world eventualities.
6. Imaginative and prescient as a Key Part: Imaginative and prescient performs a pivotal position in enabling robots to carry out parkour with agility. Imaginative and prescient sensors, equivalent to depth cameras, present robots with vital details about their environment. This visible notion permits robots to sense impediment properties, put together for agile maneuvers, and make knowledgeable selections whereas approaching obstacles.
7. Efficiency: The proposed technique surpasses a number of baseline strategies and ablations. Notably, the two-stage RL method with smooth dynamics constraints accelerates studying considerably. Robots skilled utilizing this technique obtain increased success charges in duties requiring exploration, together with climbing, leaping, crawling, and tilting. Moreover, recurrent neural networks show indispensable for expertise that demand reminiscence, equivalent to climbing and leaping.
In conclusion, this analysis addresses the problem of effectively instructing robots agile parkour expertise. The progressive two-stage RL method with smooth dynamics constraints has revolutionized how robots purchase these expertise. It leverages imaginative and prescient, simulation, reward constructions, and area adaptation, opening up new potentialities for robots to navigate advanced environments with precision and agility. Imaginative and prescient’s integration underscores its significance in robotic dexterity, permitting real-time notion and dynamic decision-making. In abstract, this progressive method marks a big development in robotic locomotion, fixing the issue of instructing parkour expertise and increasing robots’ capabilities in advanced duties.
Take a look at the Paper, Code, and Project Page. All Credit score For This Analysis Goes To the Researchers on This Challenge. Additionally, don’t neglect to affix our 30k+ ML SubReddit, 40k+ Facebook Community, Discord Channel, and Email Newsletter, the place we share the newest AI analysis information, cool AI initiatives, and extra.
If you like our work, you will love our newsletter..
Madhur Garg is a consulting intern at MarktechPost. He’s at present pursuing his B.Tech in Civil and Environmental Engineering from the Indian Institute of Expertise (IIT), Patna. He shares a powerful ardour for Machine Studying and enjoys exploring the newest developments in applied sciences and their sensible purposes. With a eager curiosity in synthetic intelligence and its various purposes, Madhur is decided to contribute to the sphere of Knowledge Science and leverage its potential affect in numerous industries.