NTU Singapore Researchers Suggest IT3D: A New Plug-and-Play Refinement AI Methodology for Textual content-to-3D Era
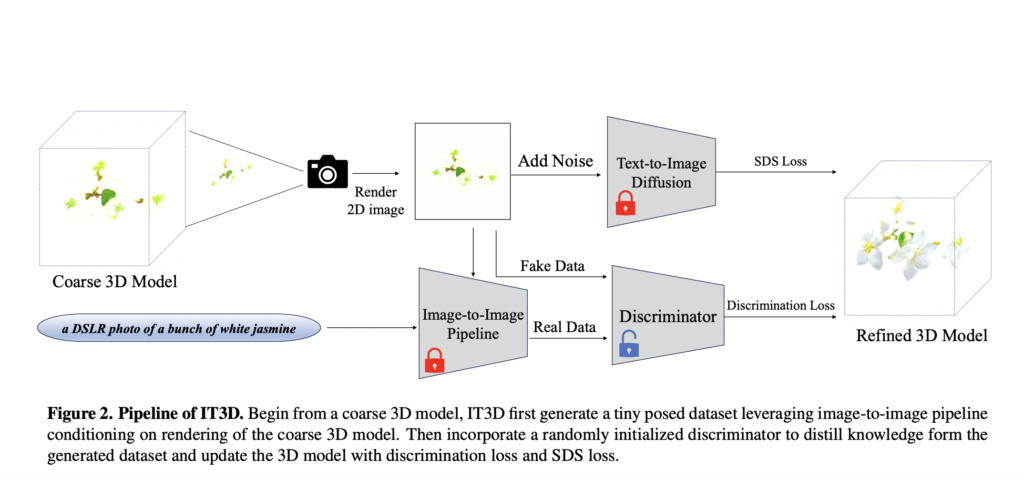
There was notable progress within the text-to-image area, sparking a surge of enthusiasm inside the analysis group to develop into 3D era. This pleasure is basically because of the emergence of approaches that make use of pre-trained 2D text-to-image diffusion fashions.
An necessary growth on this space is the inventive work finished by Dreamfusion. They introduced in a brand new methodology referred to as the Rating Distillation Sampling (SDS) algorithm, which has made an enormous distinction as a result of it might probably create quite a few completely different 3D objects simply from textual content directions. Regardless of its revolutionary method, it comes with its set of challenges. A big limitation is its management over the geometry and texture of the generated fashions, usually resulting in points like oversaturation and the multi-face look of fashions.
Moreover, researchers have observed that making an attempt to make the fashions higher by simply making the textual content directions stronger doesn’t enhance efficacy.
To fight these challenges, researchers have launched an enhanced methodology for this 3D era. This methodology facilities on creating a number of photographs from completely different angles of the specified 3D mannequin and utilizing these photographs to reconstruct the 3D object. This course of begins through the use of an current text-to-3D era mannequin, like DreamFusion, to create a primary illustration of the thing. By making these preliminary fashions, we get a primary understanding of the thing’s form and the way it’s organized in area. Then, this methodology improves the pictures of the views utilizing an image-to-image (I2I) era course of.
IT3D affords help for various 3D output representations, akin to meshes and NeRFs, and its further power lies in its environment friendly capability to vary the looks of 3D fashions utilizing textual content inputs. The above picture presents the IT3D pipeline. Starting from a rough 3D mannequin, IT3D first generates a tiny posed dataset leveraging image-to-image pipeline
conditioning on rendering of the coarse 3D mannequin. Then incorporates a randomly initialised discriminator to distil information type the generated dataset and replace the 3D mannequin with discrimination loss and SDS loss.
Furthermore, evaluation exhibits that this methodology can pace up the coaching course of, resulting in fewer obligatory coaching steps and comparable complete coaching time. This methodology can tolerate excessive variance datasets as we observe from the above picture. Lastly, the empirical findings show that the proposed methodology considerably improves the baseline fashions by way of texture element, geometry, and constancy between textual content prompts and the ensuing 3D objects.
This method has certainly supplied us with a recent perspective on text-to-3D era and has turn out to be the primary analysis work finished as an amalgamation of GAN and diffusion previous to bettering the text-to3D process.
Take a look at the Paper and GitHub link. All Credit score For This Analysis Goes To the Researchers on This Venture. Additionally, don’t overlook to affix our 29k+ ML SubReddit, 40k+ Facebook Community, Discord Channel, and Email Newsletter, the place we share the newest AI analysis information, cool AI initiatives, and extra.
If you like our work, you will love our newsletter..
Janhavi Lande, is an Engineering Physics graduate from IIT Guwahati, class of 2023. She is an upcoming information scientist and has been working on the planet of ml/ai analysis for the previous two years. She is most fascinated by this ever altering world and its fixed demand of people to maintain up with it. In her pastime she enjoys touring, studying and writing poems.