Meet KITE: An AI Framework for Semantic Manipulation Utilizing Keypoints as a Illustration for Visible Grounding and Exact Motion Inference
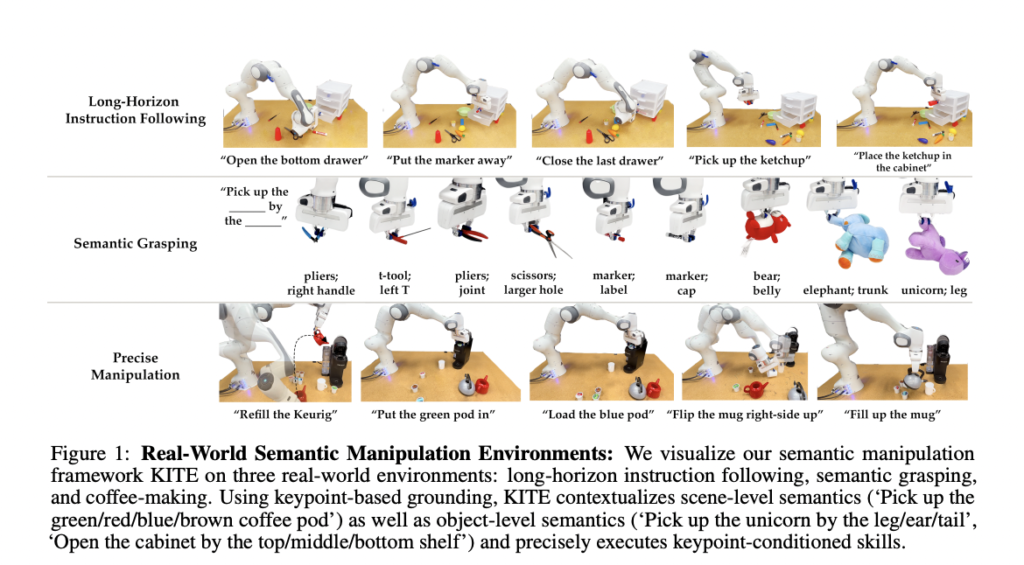
With the rising development within the area of Synthetic Intelligence, AI know-how is getting began to mix with robotics. From Laptop Imaginative and prescient and Pure Language Processing to Edge computing, AI is getting built-in with robotics to develop significant and efficient options. AI robots are machines that act in the actual world. You will need to contemplate the opportunity of language as a way of communication between folks and robots. Nonetheless, two essential points forestall fashionable robots from effectively dealing with free-form language inputs. The primary problem is of enabling a robotic to motive about what it wants to control based mostly on the directions supplied. One other is pick-and-place duties through which cautious discernment is required when selecting up objects like teddy animals by their ears versus their legs or cleaning soap bottles by their dispensers versus their sides.
Robots should extract scene and object semantics from enter directions and plan correct low-level actions in accordance to carry out semantic manipulation. To beat these challenges, researchers from Stanford College have launched KITE (Keypoints + Directions to Execution), a two-step framework for semantic manipulation. Scene semantics and object semantics are each taken into consideration in KITE. Whereas object semantics exactly localizes numerous parts inside an object occasion, scene semantics entails discriminating between numerous objects in a visible scene.
KITE’s first part entails using 2D image key factors to floor an enter instruction in a visible context. For subsequent motion inference, this process affords a really exact object-centric bias. Robotic develops a exact comprehension of the objects and their pertinent options by mapping the command to key factors within the scene. The second step of KITE entails executing a discovered keypoint-conditioned talent based mostly on the RGB-D scene commentary. The robotic makes use of these parameterized abilities to hold out the supplied instruction. Keypoints and parameterized expertise work collectively to offer fine-grained manipulation and generalization to variations in scenes and objects.
For analysis, the crew has assessed KITE’s efficiency in three precise environments: high-precision coffee-making, semantic greedy, and long-horizon 6-DoF tabletop manipulation. KITE completed the duty of getting ready espresso with successful price of 71%, successful price of 70% for semantic greedy, and successful price of 75% for instruction-following within the tabletop manipulation situation. KITE outperformed frameworks that use keypoint-based grounding versus pre-trained visible language fashions. It carried out higher than frameworks that emphasize end-to-end visuomotor management over the utilization of expertise.
KITE achieved these outcomes regardless of having had the identical or fewer demonstrations all through coaching, demonstrating its effectiveness and effectivity. To map a picture and a language phrase to a saliency heatmap and produce a key level, KITE employs a CLIPort-style method. With a purpose to output talent waypoints, the expert structure modifies PointNet++ to just accept an enter multi-view level cloud annotated with a key level. 2D key factors allow KITE to exactly attend to visible options, whereas 3D level clouds present the required 6DoF context for planning.
In conclusion, the KITE framework presents a promising resolution to the longstanding problem of enabling robots to interpret and comply with pure language instructions within the context of manipulation. It achieves fine-grained semantic manipulation with excessive precision and generalization by using the ability of key factors and instruction grounding.
Try the Paper and Project. Don’t overlook to affix our 25k+ ML SubReddit, Discord Channel, and Email Newsletter, the place we share the newest AI analysis information, cool AI initiatives, and extra. When you’ve got any questions concerning the above article or if we missed something, be happy to e mail us at Asif@marktechpost.com
🚀 Check Out 100’s AI Tools in AI Tools Club
Tanya Malhotra is a closing 12 months undergrad from the College of Petroleum & Vitality Research, Dehradun, pursuing BTech in Laptop Science Engineering with a specialization in Synthetic Intelligence and Machine Studying.
She is a Information Science fanatic with good analytical and important considering, together with an ardent curiosity in buying new expertise, main teams, and managing work in an organized method.