Closing the Hole Between Human Understanding and Machine Studying: Explainable AI as a Answer
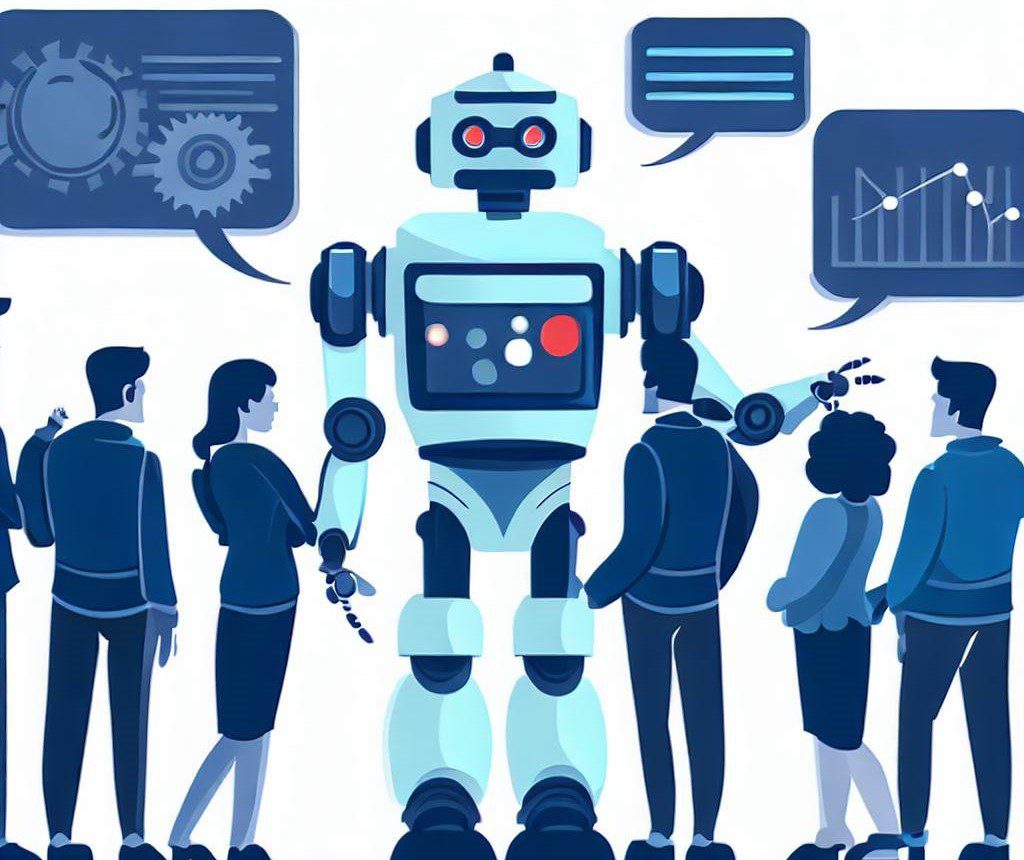

Picture by Bing Picture Creator
Have you ever ever opened your favourite procuring app and the very first thing you see is a advice for a product that you just didn’t even know you wanted, however you find yourself shopping for because of the well timed advice? Or have you ever opened your go-to music app and been delighted to see a forgotten gem by your favourite artist really helpful proper on the highest as one thing “you would possibly like”? Knowingly, or unknowingly, all of us encounter selections, actions, or experiences which were generated by Synthetic Intelligence (AI) at the moment. Whereas a few of these experiences are pretty innocuous (spot-on music suggestions, anybody?), some others would possibly typically trigger some unease (“How did this app know that I’ve been considering of doing a weight reduction program?”). This unease escalates to fret and mistrust with regards to issues of privateness about oneself and one’s family members. Nonetheless, realizing how or why one thing was really helpful to you’ll be able to assist with a few of that unease.
That is the place Explainable AI, or XAI, is available in. As AI-enabled techniques grow to be increasingly more ubiquitous, the necessity to perceive how these techniques make selections is rising. On this article, we are going to discover XAI, talk about the challenges in interpretable AI fashions, developments in making these fashions extra interpretable and supply pointers for corporations and people to implement XAI of their merchandise to foster person belief in AI.
Explainable AI (XAI) is the power of AI techniques to have the ability to present explanations for his or her selections or actions. XAI bridges the vital hole between an AI system deciding and the top person understanding why that call was made. Earlier than the appearance of AI, techniques would most frequently be rule-based (e.g., if a buyer buys pants, advocate belts. Or if an individual switches on their “Good TV”, hold rotating the #1 advice between mounted 3 choices). These experiences supplied a way of predictability. Nonetheless, as AI grew to become mainstream, connecting the dots backward from why one thing will get proven or why some determination is made by a product isn’t simple. Explainable AI may also help in these situations.
Explainable AI (XAI) permits customers to know why an AI system determined one thing and what components went into the choice. For instance, while you open your music app, you would possibly see a widget referred to as “Since you like Taylor Swift” adopted by suggestions which can be pop music and much like Taylor Swift’s songs. Otherwise you would possibly open a procuring app and see “Suggestions primarily based in your current procuring historical past” adopted by child product suggestions since you purchased some child toys and garments within the current few days.
XAI is especially vital in areas the place high-stakes selections are made by AI. For instance, algorithmic buying and selling and different monetary suggestions, healthcare, autonomous automobiles, and extra. Having the ability to present a proof for selections may also help customers perceive the rationale, determine any biases launched within the mannequin’s decision-making due to the information on which it’s educated, right errors within the selections, and assist construct belief between people and AI. Moreover, with growing regulatory pointers and authorized necessities which can be rising, the significance of XAI is barely set to develop.
If XAI supplies transparency to customers, then why not make all AI fashions interpretable? There are a number of challenges that stop this from taking place.
Superior AI fashions like deep neural networks have a number of hidden layers between the inputs and output. Every layer takes within the enter from a earlier layer, performs computation on it, and passes it on because the enter to the subsequent layer. The complicated interactions between layers make it arduous to hint the decision-making course of so as to make it explainable. That is the rationale why these fashions are also known as black bins.
These fashions additionally course of high-dimensional information like pictures, audio, textual content, and extra. Having the ability to interpret the affect of every characteristic so as to have the ability to decide which characteristic contributed essentially the most to a choice is difficult. Simplifying these fashions to make them extra interpretable leads to a lower of their efficiency. For instance, less complicated and extra “comprehensible” fashions like determination timber would possibly sacrifice predictive efficiency. In consequence, buying and selling off efficiency and accuracy for the sake of predictability can also be not acceptable.
With the rising want for XAI to proceed constructing human belief in AI, there have been strides in current occasions on this space. For instance, there are some fashions like determination timber, or linear fashions, that make interpretability pretty apparent. There are additionally symbolic or rule-based AI fashions that target the specific illustration of data and data. These fashions usually want people to outline guidelines and feed area data to the fashions. With the lively growth taking place on this area, there are additionally hybrid fashions that mix deep studying with interpretability, minimizing the sacrifice made on efficiency.
Empowering customers to know increasingly more why AI fashions resolve what they resolve may also help foster belief and transparency concerning the fashions. It could result in improved, and symbiotic, collaboration between people and machines the place the AI mannequin helps people in decision-making with transparency and people assist tune the AI mannequin to take away biases, inaccuracies, and errors.
Under are some methods by which corporations and people can implement XAI of their merchandise:
- Choose an Interpretable Mannequin the place you’ll be able to – The place they suffice and serve properly, interpretable AI fashions needs to be chosen over these that aren’t interpretable simply. For instance, in healthcare, less complicated fashions like determination timber may also help docs perceive why an AI mannequin really helpful a sure prognosis, which may also help foster belief between the physician and the AI mannequin. Characteristic engineering methods like one-hot coding or characteristic scaling that enhance interpretability needs to be used.
- Use Submit-hoc Explanations – Use methods like characteristic significance and a focus mechanisms to generate post-hoc explanations. For instance, LIME (Native Interpretable Mannequin-agnostic Explanations) is a way that explains the predictions of fashions. It generates characteristic significance scores to spotlight each characteristic’s contribution to a mannequin’s determination. For instance, if you find yourself “liking” a selected playlist advice, the LIME methodology would attempt to add and take away sure songs from the playlist and predict the probability of your liking the playlist and conclude that the artists whose songs are within the playlist play a giant position in your liking or disliking the playlist.
- Communication with Customers – Strategies like LIME or SHAP (SHapley Additive exPlanations) can be utilized to supply a helpful rationalization about particular native selections or predictions with out essentially having to clarify all of the complexities of the mannequin total. Visible cues like activation maps or consideration maps will also be leveraged to spotlight what inputs are most related to the output generated by a mannequin. Latest applied sciences like Chat GPT can be utilized to simplify complicated explanations in easy language that may be understood by customers. Lastly, giving customers some management to allow them to work together with the mannequin may also help construct belief. For instance, customers might strive tweaking inputs in several methods to see how the output modifications.
- Steady Monitoring – Corporations ought to implement mechanisms to watch the efficiency of fashions and routinely detect and alarm when biases or drifts are detected. There needs to be common updating and fine-tuning of fashions, in addition to audits and evaluations to make sure that the fashions are compliant with regulatory legal guidelines and assembly moral requirements. Lastly, even when sparingly, there needs to be people within the loop to supply suggestions and corrections as wanted.
In abstract, as AI continues to develop, it turns into crucial to construct XAI so as to preserve person belief in AI. By adopting the rules articulated above, corporations and people can construct AI that’s extra clear, comprehensible, and easy. The extra corporations undertake XAI, the higher the communication between customers and AI techniques can be, and the extra customers will really feel assured about letting AI make their lives higher
Ashlesha Kadam leads a worldwide product crew at Amazon Music that builds music experiences on Alexa and Amazon Music apps (net, iOS, Android) for hundreds of thousands of consumers throughout 45+ international locations. She can also be a passionate advocate for girls in tech, serving as co-chair for the Human Pc Interplay (HCI) observe for Grace Hopper Celebration (greatest tech convention for girls in tech with 30K+ individuals throughout 115 international locations). In her free time, Ashlesha loves studying fiction, listening to biz-tech podcasts (present favourite – Acquired), climbing within the stunning Pacific Northwest and spending time along with her husband, son and 5yo Golden Retriever.