This AI Analysis Proposes PerSAM: A Coaching-Free Personalization Strategy For The Phase Something Mannequin (SAM)
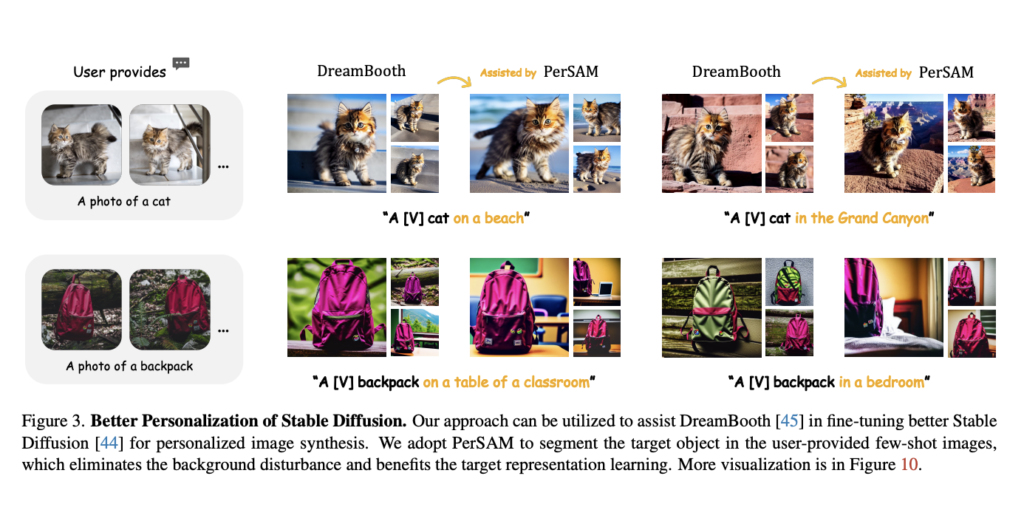
In depth availability of pre-training knowledge and computing sources, basis fashions in imaginative and prescient, language, and multi-modality have turn out to be extra frequent. They exhibit different interactions, together with human suggestions and distinctive generalization energy in zero-shot settings. Phase Something (SAM) creates a fragile knowledge engine for gathering 11M image-mask knowledge, then trains a potent segmentation basis mannequin referred to as SAM, utilizing inspiration from the successes of big language fashions. It begins by defining a brand-new promptable segmentation paradigm, which inputs a constructed immediate and outputs the anticipated masks. Any object in a visible atmosphere could also be segmented utilizing SAM’s acceptable immediate, which incorporates factors, containers, masks, and free-form phrases.

Nonetheless, SAM is unable to partition sure visible notions by nature. Think about eager to take away the clock from a shot of your bed room or crop out your cute pet canine from a photograph album. Utilizing the usual SAM mannequin would take a variety of effort and time. You should discover the goal merchandise in every picture in varied positions or conditions earlier than activating SAM and giving it particular directions for segmentation. Subsequently, they inquire whether or not they can shortly customise SAM to partition distinctive graphic notions. To do that, researchers from Shanghai Synthetic Intelligence Laboratory, CUHK MMLab, Tencent Youtu Lab, CFCS, Faculty of CS and Peking College counsel PerSAM, a customization technique for the Phase Something Mannequin that requires no coaching. Utilizing solely one-shot knowledge—a user-provided picture and a crude masks denoting the private idea—their method successfully customizes SAM.
They current three approaches to releasing SAM’s decoder’s personalization potential whereas processing the check picture. To be extra exact, they first encode the goal object’s embedding within the reference image utilizing SAM’s picture encoder and the equipped masks. The characteristic similarity between the merchandise and every pixel within the new check image is then calculated. The estimated characteristic similarity directs every token-to-image cross-attention layer within the SAM decoder. Moreover, two factors are chosen because the positive-negative pair and encoded as immediate tokens to supply SAM with a location beforehand.
Because of this, for environment friendly characteristic interplay, the immediate tokens are pressured to focus totally on entrance goal areas.
• Targeted, directed consideration
• Goal-specific Prompting
• Caledonia Put up-refinement
They implement a two-step post-refinement method for ends in sharper segmentation. They use SAM to enhance the produced masks steadily. It solely provides 100ms to the method.
As proven in Determine 2, PerSAM reveals good customized segmentation efficiency for a single participant in a variety of positions or settings when utilizing the designs above. Nonetheless, there could sometimes be failure situations when the topic has hierarchical buildings that must be segmented, equivalent to the highest of a container, the pinnacle of a toy robotic, or a cap on prime of a teddy bear.

Provided that SAM could settle for each the native element and the worldwide kind as acceptable masks on the pixel degree, this uncertainty makes it troublesome for PerSAM to decide on the best dimension for the segmentation output. To ease this, additionally they current PerSAM-F, a fine-tuning variation of their methodology. They fine-tune two parameters inside 10 seconds whereas freezing the whole SAM to take care of its pre-trained information. They particularly permit SAM to supply quite a few segmentation outcomes with varied masks scales. They use learnable relative weights for every scale and a weighted summation as the ultimate masks output to decide on the optimum scale for various gadgets adaptively.
As may be seen in Determine 2 (Proper), PerSAM-T shows improved segmentation accuracy due to this efficient one-shot coaching. The anomaly downside may be successfully managed by weighting multi-scale masks relatively than immediate tuning or adapters. Additionally they be aware that their methodology can let DreamBooth higher fine-tune Steady Diffusion for personalized text-to-image manufacturing. DreamBooth and its related works take a small set of images having a selected visible notion, like your favourite cat, and switch them into an identifier within the phrase embedding house that’s subsequently used to symbolize the goal merchandise within the phrase. Nonetheless, the identifier consists of visible particulars concerning the offered images’ backgrounds, equivalent to stairs.
This could override the brand new backgrounds within the generated pictures and disturb the illustration studying of the goal object. Subsequently, they suggest to leverage their PerSAM to section the goal object effectively and solely supervise Steady Diffusion by the foreground space within the few-shot pictures, enabling extra numerous and higher-fidelity synthesis. They summarize the contributions of their paper as follows:
• Personalised Segmentation Activity. From a brand new standpoint, they examine tips on how to customise segmentation basis fashions into customized situations with minimal expense, i.e., from basic to personal functions.
• Environment friendly Adaption of SAM. They examine for the primary time tips on how to modify SAM for downstream functions by merely adjusting two parameters, they usually current two easy options: PerSAM and PerSAM-F.
• Analysis of Personalization. They add annotations to PerSeg, a brand-new segmentation dataset containing quite a few classes in varied circumstances. Moreover, they check their technique utilizing efficient video object segmentation.
• Improved Steady Diffusion Personalization. The segmentation of the goal merchandise within the few-shot images reduces background noise and enhances DreamBooth’s skill to generate customized content material.
Try the Paper and Code. Don’t overlook to hitch our 21k+ ML SubReddit, Discord Channel, and Email Newsletter, the place we share the newest AI analysis information, cool AI initiatives, and extra. You probably have any questions concerning the above article or if we missed something, be happy to e mail us at Asif@marktechpost.com
🚀 Check Out 100’s AI Tools in AI Tools Club
Aneesh Tickoo is a consulting intern at MarktechPost. He’s at the moment pursuing his undergraduate diploma in Knowledge Science and Synthetic Intelligence from the Indian Institute of Know-how(IIT), Bhilai. He spends most of his time engaged on initiatives aimed toward harnessing the ability of machine studying. His analysis curiosity is picture processing and is enthusiastic about constructing options round it. He loves to attach with folks and collaborate on attention-grabbing initiatives.