A novel household of auxiliary duties primarily based on the successor measure to enhance the representations that deep reinforcement studying brokers purchase
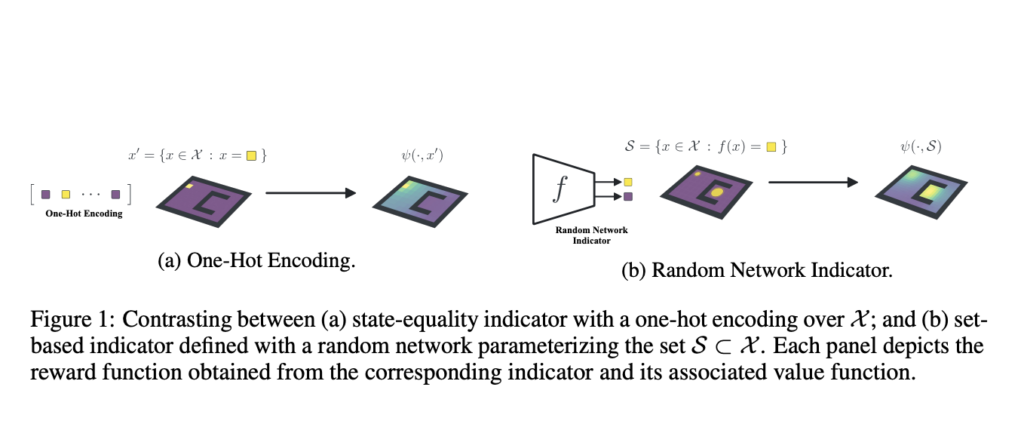
In deep reinforcement studying, an agent makes use of a neural community to map observations to a coverage or return prediction. This community’s operate is to show observations right into a sequence of progressively finer traits, which the ultimate layer then linearly combines to get the specified prediction. The agent’s illustration of its present state is how most individuals view this alteration and the intermediate traits it creates. Based on this attitude, the training agent carries out two duties: illustration studying, which entails discovering useful state traits, and credit score task, which entails translating these options into exact predictions.
Fashionable RL strategies sometimes incorporate equipment that incentivizes studying good state representations, akin to predicting speedy rewards, future states, or observations, encoding a similarity metric, and information augmentation. Finish-to-end RL has been proven to acquire good efficiency in all kinds of issues. It’s often possible and fascinating to accumulate a sufficiently wealthy illustration earlier than performing credit score task; illustration studying has been a core element of RL since its inception. Utilizing the community to forecast extra duties associated to every state is an environment friendly strategy to study state representations.
A set of properties akin to the first parts of the auxiliary job matrix could also be demonstrated as being induced by extra duties in an idealized setting. Thus, the discovered illustration’s theoretical approximation error, generalization, and stability could also be examined. It might come as a shock to learn the way little is thought about their conduct in larger-scale environment. It’s nonetheless decided how using extra duties or increasing the community’s capability would have an effect on the scaling options of illustration studying from auxiliary actions. This essay seeks to shut that data hole. They use a household of extra incentives which may be sampled as a place to begin for his or her technique.
Researchers from McGill College, Université de Montréal, Québec AI Institute, College of Oxford and Google Analysis particularly apply the successor measure, which expands the successor illustration by substituting set inclusion for state equality. On this scenario, a household of binary capabilities over states serves as an implicit definition for these units. Most of their analysis is concentrated on binary operations obtained from randomly initialized networks, which have already been proven to be helpful as random cumulants. Regardless of the likelihood that their findings would additionally apply to different auxiliary rewards, their strategy has a number of benefits:
- It may be simply scaled up utilizing extra random community samples as additional duties.
- It’s straight associated to the binary reward capabilities present in deep RL benchmarks.
- It’s partially comprehensible.
Predicting the expected return of the random coverage for the related auxiliary incentives is the true extra job; within the tabular setting, this corresponds to proto-value capabilities. They check with their strategy as proto-value networks in consequence. They analysis how effectively this strategy works within the arcade studying setting. When utilized with linear operate approximation, they study the traits discovered by PVN and show how effectively they symbolize the temporal construction of the setting. Total, they uncover that PVN solely wants a small portion of interactions with the setting reward operate to yield state traits wealthy sufficient to assist linear worth estimates equal to these of DQN on numerous video games.
They found in ablation analysis that increasing the worth community’s capability considerably enhances the efficiency of their linear brokers and that bigger networks can deal with extra jobs. In addition they uncover, considerably unexpectedly, that their technique works greatest with what might seem like a modest variety of extra duties: the smallest networks they analyze create their greatest representations from 10 or fewer duties, and the most important, from 50 to 100 duties. They conclude that particular duties might lead to representations which are far richer than anticipated and that the influence of any given job on fixed-size networks nonetheless must be absolutely understood.
Take a look at the Paper. Don’t overlook to hitch our 21k+ ML SubReddit, Discord Channel, and Email Newsletter, the place we share the newest AI analysis information, cool AI initiatives, and extra. You probably have any questions relating to the above article or if we missed something, be at liberty to electronic mail us at Asif@marktechpost.com
🚀 Check Out 100’s AI Tools in AI Tools Club
Aneesh Tickoo is a consulting intern at MarktechPost. He’s at the moment pursuing his undergraduate diploma in Knowledge Science and Synthetic Intelligence from the Indian Institute of Expertise(IIT), Bhilai. He spends most of his time engaged on initiatives geared toward harnessing the ability of machine studying. His analysis curiosity is picture processing and is captivated with constructing options round it. He loves to attach with folks and collaborate on fascinating initiatives.