How RallyPoint and AWS are personalizing job suggestions to assist navy veterans and repair suppliers transition again into civilian life utilizing Amazon Personalize
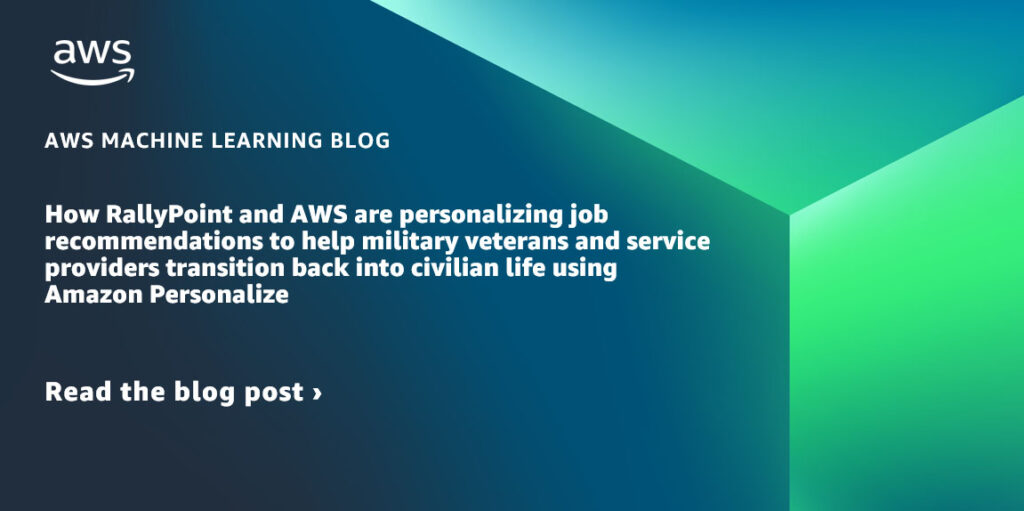
This put up was co-written with Dave Gowel, CEO of RallyPoint. In his personal phrases, “RallyPoint is a web-based social {and professional} community for veterans, service members, relations, caregivers, and different civilian supporters of the US armed forces. With two million members on the platform, the corporate gives a cushty place for this deserving inhabitants to attach with one another and applications designed to help them.”
All those that serve – and people who help them – usually face quite a lot of employment challenges when a servicemember transitions again into civilian life. RallyPoint has recognized the transition interval to a civilian profession as a significant alternative to enhance the standard of life for this inhabitants by creating automated and compelling job suggestions. Nevertheless, the staff traditionally employed a rule-based curation technique to suggest jobs all through its person expertise, which doesn’t permit members to get job suggestions customized to their particular person expertise, experience, and pursuits.
“To enhance this expertise for its members, we at RallyPoint wished to discover how machine studying (ML) may assist. We don’t need our servicemembers, veterans, and their family members to waste time looking for a satisfying civilian profession path after they determine to depart the navy. It ought to be a simple course of. We would like our members to inform us about their navy experiences, any faculties they’ve attended, and their private preferences. Then by leveraging what we all know from our thousands and thousands of navy and veteran members, related open jobs ought to be simply surfaced as an alternative of laboriously searched. This free service for our members can also be anticipated to drive income by a minimum of seven figures from employers in search of the appropriate navy and veteran expertise, permitting us to construct extra free capabilities for our members.”
This weblog put up summarizes how the Amazon Machine Learning Solution Lab (MLSL) partnered with RallyPoint to drive a 35% enchancment in customized profession suggestions and a 66x enhance in protection, amongst different enhancements for RallyPoint members from the present rule-based implementation.
“MLSL helped RallyPoint save and enhance the lives of the US navy group. Lucky to work on a number of complicated and impactful tasks with MLSL to help essentially the most deserving of populations, RallyPoint accelerated development in a number of core organizational metrics within the course of. MLSL’s excessive caliber expertise, tradition, and deal with aiding our realization of measurable and compelling outcomes from machine studying investments enabled us to cut back suicide threat, enhance profession transition, and pace up necessary connections for our service members, veterans, and their households.”
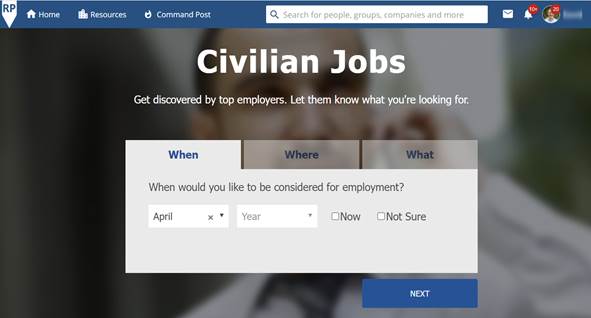
*Picture supplied by the RallyPoint staff.
The next sections cowl the enterprise and technical challenges, the strategy taken by the AWS and RallyPoint groups, and the efficiency of applied answer that leverages Amazon Personalize.
Amazon Personalize makes it simple for builders to construct purposes able to delivering a wide selection of personalization experiences, together with particular product suggestions, customized product re-ranking, and customised direct advertising and marketing. Amazon Personalize is a totally managed ML service that goes past inflexible, static rule-based suggestion techniques by coaching, tuning, and deploying customized ML fashions to ship extremely personalized suggestions to clients throughout industries equivalent to retail and media and leisure.
Enterprise and Technical challenges
A number of enterprise challenges impressed this partnership. Probably the most pertinent was the clickthrough charge on the highest 10 advisable jobs on the RallyPoint web site. RallyPoint analyzed person engagement inside their platform and found that they wanted to extend the variety of related jobs that customers are clicking. The concept is that the extra related a advisable job is, the upper the chance of members making use of to these jobs, resulting in improved employment outcomes.
The following problem was to extend the engagement by members on job providers provided on the location. RallyPoint provides the chance for individuals to “Construct your model and interact the navy group, promote your services and products, run recruitment advertising and marketing campaigns, put up jobs, and search veteran expertise.” They as soon as once more recognized a possibility to use AWS Personalize to assist extra individuals transition to civilian life, and sought to enhance their click-to-customer conversion numbers, main to higher outcomes for RallyPoint’s direct clients.
From a technical perspective, like many conventional recommender system issues, knowledge sparsity and an extended tail was a problem to beat. The pattern set of de-identified, already publicly shared knowledge included 1000’s of anonymized person profiles, with greater than fifty user-metadata factors, however many had inconsistent or lacking meta-data/profile info. To deal with this, the staff leveraged the Amazon Personalize chilly begin suggestion performance for related customers.
Answer overview
To unravel the issue, MLSL collaborated with RallyPoint to assemble a customized Amazon Personalize pipeline for RallyPoint. A few of the providers used embody Amazon Simple Storage Service (Amazon S3), Amazon SageMaker Notebook Instances, and Amazon Personalize. The next diagram illustrates the answer structure.
The anonymized uncooked knowledge used for the answer consisted of a historical past of interactions with job postings together with metadata on person profiles and job positions. This was saved in S3. The MLSL staff used Amazon SageMaker Pocket book Cases to organize knowledge as enter to Amazon Personalize. This step included knowledge preprocessing, characteristic engineering, and creating dataset teams and schemas required for Amazon Personalize. For extra info confer with Creating a Custom dataset group.
The following step was to create an answer in Amazon Personalize. An answer refers back to the mixture of an Amazon Personalize recipe, personalized parameters, and a number of answer variations. For extra info confer with Creating a solution. The staff used the User-Personalization recipe to generate user-specific job suggestions for customers in a validation set. The Amazon Personalize outputs, together with the job suggestions and efficiency metrics, are saved in an Amazon S3 bucket for additional evaluation.
Within the last step, the staff used a pocket book occasion to organize the output suggestions for exterior analysis by human annotators, as described within the Utilizing Area Consultants part.
Analysis of Amazon Personalize outcomes
The efficiency of an Amazon Personalize answer model might be evaluated utilizing offline metrics, online metrics, and A/B testing. Offline metrics permit you to view the consequences of modifying hyperparameters and algorithms used to coach your fashions, calculated towards historic knowledge. On-line metrics are the empirical outcomes noticed in your person’s interactions with real-time suggestions supplied in a dwell setting (equivalent to clickthrough charge). A/B testing is a web-based technique of evaluating the efficiency of a number of answer variations to a default answer. Customers are randomly assigned to both the management (default) group or one of many therapy (take a look at) teams. The management group customers obtain suggestions from the default answer (baseline), whereas every of the therapy teams work together with a distinct answer model. Statistical significance exams are used to check the efficiency metrics (equivalent to clickthrough charge or latency) and enterprise metrics (equivalent to income) to that of the default answer.
Amazon Personalize measures offline metrics throughout coaching an answer model. The staff used offline metrics equivalent to Imply Reciprocal Rank (MRR), normalized discounted cumulative achieve (NCDG@ok), Precision@ok, and Protection. For the definitions of all out there offline metrics, confer with Metric definitions.
Though Amazon Personalize gives an intensive checklist of offline metrics that the staff can use to objectively measure the efficiency of options throughout coaching, on-line metrics and A/B testing are advisable to trace and validate mannequin efficiency. One caveat to those exams is that they require customers to work together with Amazon Personalize suggestions in actual time. As a result of the RallyPoint Amazon Personalize mannequin wasn’t deployed previous to this publication, the staff didn’t have outcomes to report for these exams.
Utilizing Area Consultants
A/B testing is the popular technique of analyzing the standard of a suggestion system nonetheless, utilizing area specialists to annotate suggestions is a viable precursor. Since on-line testing was not an choice, to check the robustness of the suggestions, the staff requested area specialists in RallyPoint to annotate the suggestions generated by the fashions and rely the variety of job positions the specialists agreed ought to be advisable (given a person’s info and indicated preferences) because the variety of “right” suggestions. This metric was used to check answer variations. A reputation answer (the present rule-based standards) was used as a baseline which consisted of recommending high 5 hottest job positions to each person. Furthermore, an answer with default settings was used as one other baseline mannequin referred to as Amazon Personalize baseline answer.
Outcomes
Utilizing the perfect performing mannequin resulted in a 35% enchancment within the variety of “right” suggestions over the Amazon Personalize baseline answer and a 54% enchancment over the recognition answer. The staff may additionally obtain a 66x enchancment in protection, 30x enchancment in MRR, and 2x enchancment in precision@10 when in comparison with the recognition answer. Along with the recognition answer, the staff noticed as much as 2x enhance in MRR and precision@10 when in comparison with the Amazon Personalize baseline answer.
Abstract
RallyPoint acknowledged a possibility to higher serve their clients with extra customized profession suggestions. They started their person personalization journey with buyer obsession in thoughts, partnering with the Machine Studying Options Lab. RallyPoint now has the chance to offer their customers extra useful profession suggestions, by this answer. Incorporating this improved suggestion system into their web site will lead to RallyPoint customers seeing extra related jobs of their profession feed, easing the trail to extra fulfilling careers and an improved high quality of life for his or her members.
Use Amazon Personalize to offer an individualized expertise in your customers as we speak! In case you’d wish to collaborate with specialists to convey ML options to your group, contact the Amazon ML Solutions Lab.
Extra sources
For extra details about Amazon Personalize, see the next:
Concerning the Authors
Dave Gowel is an Military veteran and the CEO of RallyPoint. Dave is a graduate of West Level and the US Military Ranger Faculty, served in Iraq as a tank platoon chief, and taught as an assistant professor on the Massachusetts Institute of Know-how ROTC program. RallyPoint is the third expertise firm for which Dave has been CEO.
Matthew Rhodes is a Knowledge Scientist working within the Amazon ML Solutions Lab. He makes a speciality of constructing machine studying pipelines that contain ideas equivalent to pure language processing and pc imaginative and prescient.
Amin Tajgardoon is an Utilized Scientist on the Amazon ML Solutions Lab. He has an intensive background in pc science and machine studying. Specifically, Amin’s focus has been on deep studying and forecasting, prediction clarification strategies, mannequin drift detection, probabilistic generative fashions, and purposes of AI within the healthcare area.
Yash Shah is a Science Supervisor within the Amazon ML Solutions Lab. He and his staff of utilized scientists and machine studying engineers work on a spread of machine studying use instances from healthcare, sports activities, automotive and manufacturing.
Vamshi Krishna Enabothala is a Sr. Utilized AI Specialist Architect at AWS. He works with clients from completely different sectors to speed up high-impact knowledge, analytics, and machine studying initiatives. He’s captivated with suggestion techniques, NLP, and pc imaginative and prescient areas in AI and ML. Exterior of labor, Vamshi is an RC fanatic, constructing RC gear (planes, vehicles, and drones), and in addition enjoys gardening.
Greg Tolmie is an Account Supervisor on the AWS Public Sector ISV companions staff. Greg helps a portfolio of AWS public sector ISV companions to assist them develop and mature their adoption of AWS providers whereas maximizing advantages of the AWS associate community.