Mastering the Artwork of Video Filters with AI Neural Preset: A Neural Community Strategy
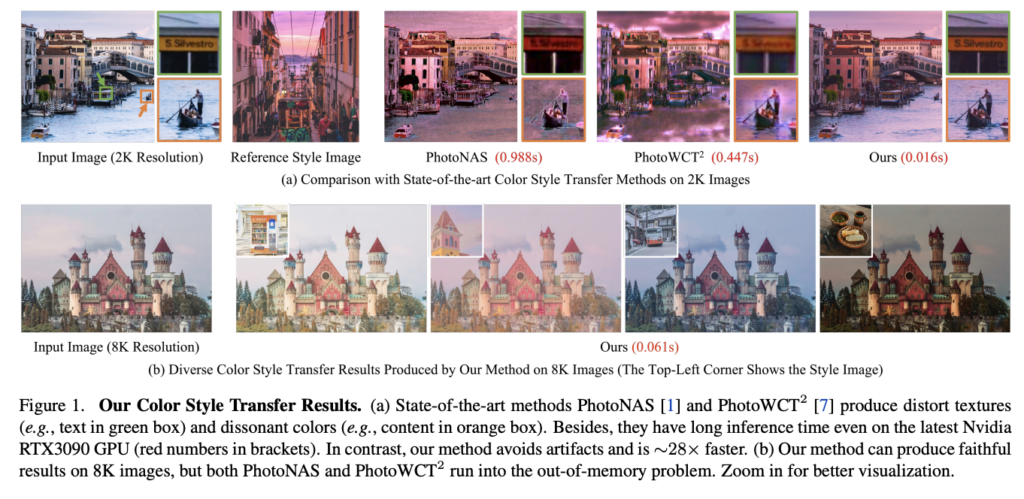
With tens of millions of pictures and video content material posted every day, visible filters have turn into a necessary function of social media platforms, permitting customers to reinforce and customise their video content material with numerous results and changes. These filters have revolutionized the best way we talk and share experiences, offering us with the power to create visually interesting and fascinating content material that captures our viewers’s consideration.
Furthermore, with the rise of AI, these filters have turn into much more subtle, permitting us to govern video content material in beforehand inconceivable methods with just a few clicks. AI-powered video filters can mechanically alter lighting, coloration steadiness, and different parts of a video, permitting creators to realize a professional-quality look with out the necessity for intensive technical data.
Though very highly effective, these filters are designed with pre-defined parameters, so they can not generate constant coloration types for pictures with various appearances. Due to this fact, cautious changes by the customers are nonetheless vital. To handle this drawback, coloration type switch strategies have been launched to mechanically map the colour type from a well-retouched picture (i.e., the type picture) to a different (i.e., the enter picture).
Present strategies, nonetheless, produce outcomes affected by artifacts like coloration and texture inconsistencies and require a big period of time and assets to run. Because of this, a novel framework for coloration type transferring termed Neural Preset has been developed.
An outline of the workflow is depicted within the determine under.

The proposed technique differs from the present state-of-the-art strategies, using Deterministic Neural Colour Mapping (DNCM) as an alternative of convolutional fashions for coloration mapping. DNCM makes use of an image-adaptive coloration mapping matrix that multiplies the pixels of the identical coloration to provide a particular coloration and successfully eliminates unrealistic artifacts. Moreover, DNCM features independently on every pixel, requiring a small reminiscence footprint and supporting high-resolution inputs. In contrast to typical 3D filters that depend on the regression of tens of 1000’s of parameters, DNCM can mannequin arbitrary coloration mappings utilizing only some hundred learnable parameters.
Neural Preset works in two distinct phases, permitting for fast switching between totally different types. The underlying construction depends on the encoder E, which predicts parameters employed within the normalization and stylization phases.
The primary stage creates an nDNCM from the enter picture, normalizing the colours and mapping the picture to a color-style area representing the content material. The second stage builds an sDNCM from the type picture, which stylizes the normalized picture to the specified goal coloration type. This design ensures that the parameters of sDNCM could be saved as coloration type presets and utilized by totally different enter pictures. Moreover, the enter picture could be styled utilizing quite a lot of color-style presets after being normalized with nDNCM.
A comparability of the proposed method with the state-of-the-art strategies is introduced under.

Based on the authors, Neural Preset outperforms state-of-the-art strategies considerably in numerous elements, comparable to correct outcomes for 8K pictures, constant coloration type switch outcomes throughout video frames, and ∼28× speedup on an Nvidia RTX3090 GPU, supporting real-time performances at 4K decision.
This was the abstract of Neural Preset, an AI framework for real-time and color-consistent high-quality type switch.
If you’re or wish to study extra about this work, yow will discover a hyperlink to the paper and the mission web page.
Try the Paper and Project. All Credit score For This Analysis Goes To the Researchers on This Mission. Additionally, don’t neglect to hitch our 16k+ ML SubReddit, Discord Channel, and Email Newsletter, the place we share the most recent AI analysis information, cool AI initiatives, and extra.
Daniele Lorenzi acquired his M.Sc. in ICT for Web and Multimedia Engineering in 2021 from the College of Padua, Italy. He’s a Ph.D. candidate on the Institute of Info Know-how (ITEC) on the Alpen-Adria-Universität (AAU) Klagenfurt. He’s presently working within the Christian Doppler Laboratory ATHENA and his analysis pursuits embrace adaptive video streaming, immersive media, machine studying, and QoS/QoE analysis.