Synth2: Boosting Visible-Language Fashions with Artificial Captions and Picture Embeddings by Researchers from Google DeepMind
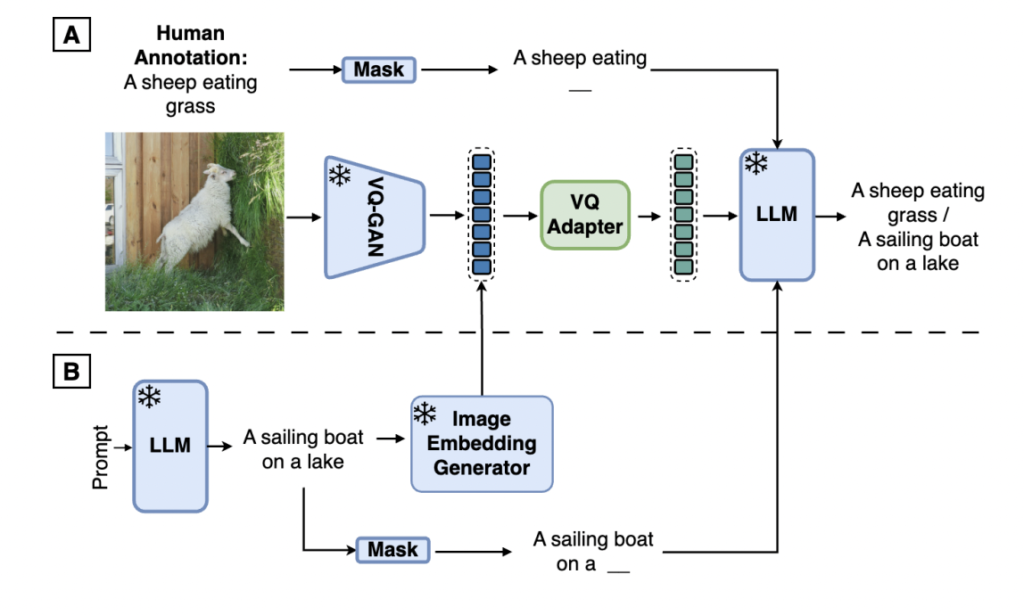
VLMs are potent instruments for greedy visible and textual knowledge, promising developments in duties like picture captioning and visible query answering. Restricted knowledge availability hampers their efficiency. Latest strides present that pre-training VLMs on bigger image-text datasets improves downstream duties. But, creating such datasets faces challenges: shortage of paired knowledge, excessive curation prices, low variety, and noisy internet-sourced knowledge.
Earlier research reveal the effectiveness of VLMs in duties like picture captioning, using various architectures, and pretraining methods. Latest developments in high-quality picture turbines have sparked curiosity in utilizing generative fashions for artificial knowledge technology. This pattern impacts varied laptop imaginative and prescient duties, together with semantic segmentation, human movement understanding, and picture classification. This research additionally explores integrating data-driven generative fashions inside VLMs, emphasizing effectivity by producing picture embeddings straight built-in into the mannequin, displaying superiority over present approaches.
The researchers from Google DeepMind have proposed Synth2. This technique leverages pre-trained generative textual content and picture fashions to create artificial paired knowledge for VLMs, addressing knowledge shortage, price, and noise challenges. It generates each textual content and pictures synthetically, avoiding reliance on real-world knowledge. The method operates on the embedding stage, bypassing expensive pixel-space rendering, thus enhancing effectivity with out compromising efficiency. Pre-training the text-to-image mannequin on the identical dataset used for VLM coaching ensures truthful analysis and prevents unintended information switch.
Synth2 leverages pre-trained generative textual content and picture fashions to create artificial paired knowledge for VLM coaching. It consists of parts for Caption Technology, using LLMs with class-based prompting for various captions, and Picture Technology, using a managed text-to-image generator skilled on the identical dataset because the VLM to make sure truthful analysis. The Synth2 VLM structure integrates VQ-GAN backbones for environment friendly interplay with synthetically generated picture embeddings, bypassing pixel-space processing and enabling seamless coaching. Additionally, a Perceiver Resampler part facilitates cross-attention between VQ tokens and language tokens within the VLM, aiding in efficient multimodal representations.
In evaluating artificial pictures for VLM coaching, Synth2 considerably improves efficiency over baselines, even with a smaller quantity of human-annotated pictures. Artificial pictures successfully substitute actual ones, enhancing VLM capabilities. Synth2 additionally outperforms state-of-the-art strategies like ITIT and DC, attaining aggressive outcomes with lowered knowledge utilization and computational assets. This highlights Synth2’s effectiveness and effectivity in enhancing VLM efficiency.
In conclusion, the researchers from Google DeepMind have proposed Synth2, which makes use of artificial image-text pairs to reinforce VLM coaching. Outcomes present improved VLM efficiency in comparison with baselines, with enhanced knowledge effectivity and scalability. This technique gives customization for particular domains and addresses resource-intensive knowledge acquisition challenges. The findings underscore the potential of artificial knowledge technology in advancing visible language understanding, suggesting avenues for additional exploration.
Try the Paper. All credit score for this analysis goes to the researchers of this challenge. Additionally, don’t overlook to observe us on Twitter. Be part of our Telegram Channel, Discord Channel, and LinkedIn Group.
For those who like our work, you’ll love our newsletter..
Don’t Neglect to hitch our 38k+ ML SubReddit