This AI Paper Proposes Infini-Gram: A Groundbreaking Strategy to Scale and Improve N-Gram Fashions Past Conventional Limits
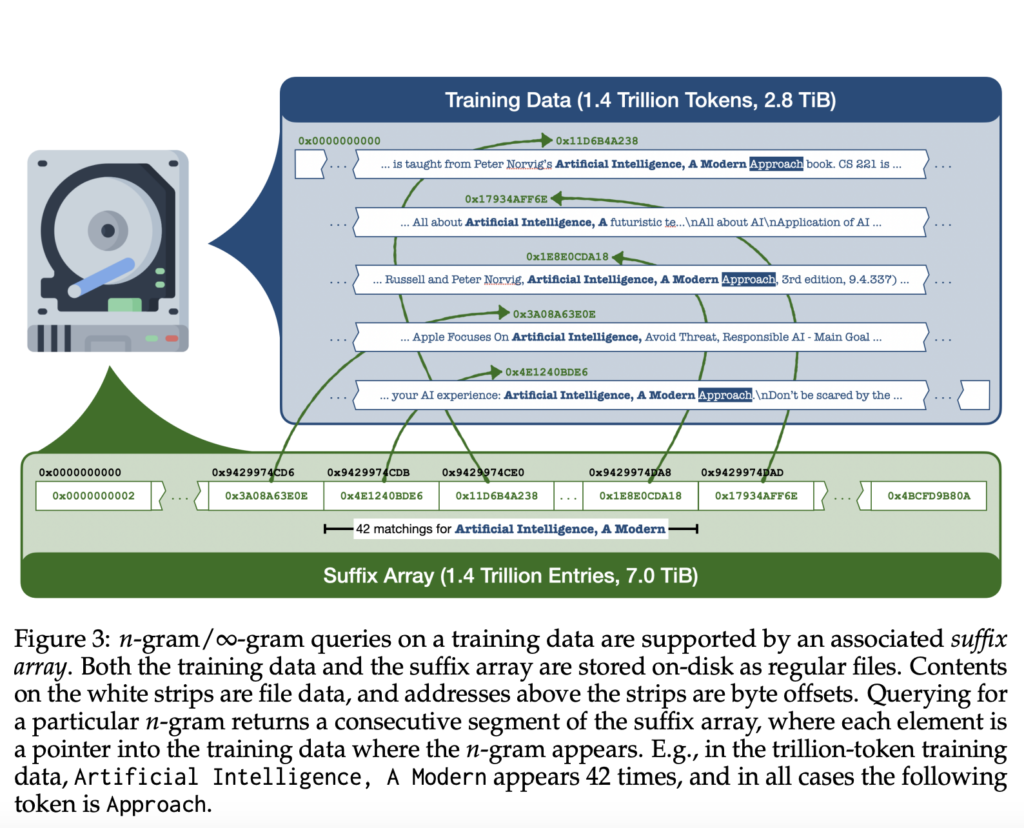
Pretrained on trillion-token corpora, massive neural language fashions (LLMs) have achieved outstanding efficiency strides (Touvron et al., 2023a; Geng & Liu, 2023). Nevertheless, the scalability advantages of such information for conventional n-gram language fashions (LMs) nonetheless must be explored. This paper from the College of Washington and Allen Institute for Synthetic Intelligence delves into the relevance of n-gram LMs within the period of neural LLMs and introduces groundbreaking developments of their modernization.
The authors affirm the continued utility of n-gram LMs in textual content evaluation and enhancing neural LLMs. To deal with this, they modernized conventional n-gram LMs by scaling coaching information to an unprecedented 1.4 trillion tokens, rivaling the dimensions of main open-source textual content corpora (Collectively, 2023; Soldaini et al., 2023). This represents the biggest n-gram LM thus far. Departing from historic constraints on n (e.g., n ≤ 5), the authors spotlight some great benefits of bigger n’s worth. Determine 1 illustrates the improved predictive capability of n-gram LMs with bigger n values, difficult standard limitations. Consequently, they introduce the idea of an ∞-gram LM, with unbounded n, using a backoff variant (Jurafsky & Martin, 2000) for improved accuracy.
The ∞-gram LM leverages a suffix array, changing impractical n-gram depend tables. This implementation, known as the infini-gram engine, achieves outstanding effectivity with 7 bytes of storage per token. The suffix array, constructed on 1.4 trillion tokens utilizing an 80-core CPU node in beneath three days, ensures low-latency, resource-efficient querying at lower than 20 milliseconds for n-gram counting. The ∞-gram engine, a testomony to innovation, makes on-disk indexes integral to inference.
The ∞-gram LM, a conceptual extension of n-gram LMs, employs backoff judiciously to boost predictive accuracy. Sparsity in ∞-gram estimates necessitate interpolation with neural LMs, addressing perplexity considerations. The paper introduces question sorts supported by Infini-gram, showcasing spectacular latency benchmarks in Desk 1.
Constructing on the suffix array implementation, the paper outlines environment friendly strategies for n-gram counting, incidence place retrieval, and doc identification. Sharding methods cut back latency proportional to the variety of shards, optimizing processing instances. Intelligent optimizations, reminiscent of reusing search outcomes and on-disk search, additional improve the velocity of ∞-gram computation.
Infini-gram’s utility throughout various neural LMs, together with GPT-2, GPT-Neo, LLaMA-2, and SILO, demonstrates constant perplexity enhancements (Desk 2). The paper underscores the importance of knowledge variety, revealing ∞-gram’s efficacy in complementing neural LMs throughout totally different mannequin collection.
Analyses with ∞-gram make clear human-written and machine-generated textual content. Notably, ∞-gram displays excessive accuracy in predicting the following token based mostly on human-written doc prefixes. The paper establishes a optimistic correlation between neural LMs and ∞-gram, suggesting the latter’s potential to boost LM efficiency in predicting human-written textual content.
The paper concludes with a visionary outlook, presenting preliminary purposes of the Infini-gram engine. From understanding textual content corpora to mitigating copyright infringement, the chances are various. The authors anticipate additional insightful analyses and revolutionary purposes fueled by Infini-gram.
Try the Paper and Model. All credit score for this analysis goes to the researchers of this undertaking. Additionally, don’t neglect to comply with us on Twitter and Google News. Be a part of our 36k+ ML SubReddit, 41k+ Facebook Community, Discord Channel, and LinkedIn Group.
For those who like our work, you’ll love our newsletter..
Don’t Neglect to affix our Telegram Channel
Vineet Kumar is a consulting intern at MarktechPost. He’s at the moment pursuing his BS from the Indian Institute of Know-how(IIT), Kanpur. He’s a Machine Studying fanatic. He’s obsessed with analysis and the newest developments in Deep Studying, Laptop Imaginative and prescient, and associated fields.