This AI Paper from Harvard Explores the Frontiers of Privateness in AI: A Complete Survey of Giant Language Fashions’ Privateness Challenges and Options
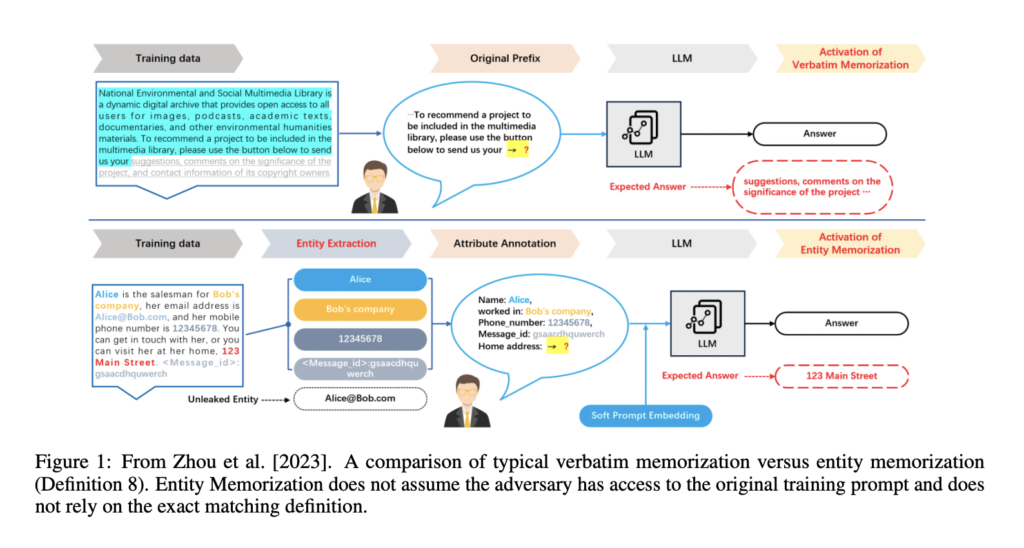
Privateness issues have grow to be a major problem in AI analysis, significantly within the context of Giant Language Fashions (LLMs). The SAFR AI Lab at Harvard Enterprise Faculty was surveyed to discover the intricate panorama of privateness points related to LLMs. The researchers centered on red-teaming fashions to focus on privateness dangers, combine privateness into the coaching course of, effectively delete knowledge from skilled fashions, and mitigate copyright points. Their emphasis lies on technical analysis, encompassing algorithm improvement, theorem proofs, and empirical evaluations.
The survey highlights the challenges of distinguishing fascinating “memorization” from privacy-infringing cases. The researchers focus on the constraints of verbatim memorization filters and the complexities of honest use legislation in figuring out copyright violation. Additionally they spotlight researchers’ technical mitigation methods, similar to knowledge filtering to stop copyright infringement.
The survey gives insights into varied datasets utilized in LLM coaching, together with the AG Information Corpus and BigPatent-G, which consist of reports articles and US patent paperwork. The researchers additionally focus on the authorized discourse surrounding copyright points in LLMs, emphasizing the necessity for extra options and modifications to soundly deploy these fashions with out risking copyright violations. They acknowledge the problem in quantifying inventive novelty and meant use, underscoring the complexities of figuring out copyright violation.
The researchers focus on using differential privateness, which provides noise to the information to stop the identification of particular person customers. Additionally they focus on federated studying, which permits fashions to be skilled on decentralized knowledge sources with out compromising privateness. The survey additionally highlights machine unlearning, which includes eradicating delicate knowledge from skilled fashions to adjust to privateness laws.
The researchers display the effectiveness of differential privateness in mitigating privateness dangers related to LLMs. Additionally they present that federated studying can prepare fashions on decentralized knowledge sources with out compromising privateness. The survey highlights machine unlearning to take away delicate knowledge from skilled fashions to adjust to privateness laws.
The survey gives a complete overview of the privateness challenges in Giant Language Fashions, providing technical insights and mitigation methods. It underscores the necessity for continued analysis and improvement to deal with the intricate intersection of privateness, copyright, and AI expertise. The proposed methodology provides promising options to mitigate privateness dangers related to LLMs, and the efficiency and outcomes display the effectiveness of those options. The survey highlights the significance of addressing privateness issues in LLMs to make sure these fashions’ protected and moral deployment.
Try the Paper and Github. All credit score for this analysis goes to the researchers of this mission. Additionally, don’t overlook to observe us on Twitter. Be part of our 36k+ ML SubReddit, 41k+ Facebook Community, Discord Channel, and LinkedIn Group.
For those who like our work, you’ll love our newsletter..
Don’t Neglect to affix our Telegram Channel
Muhammad Athar Ganaie, a consulting intern at MarktechPost, is a proponet of Environment friendly Deep Studying, with a concentrate on Sparse Coaching. Pursuing an M.Sc. in Electrical Engineering, specializing in Software program Engineering, he blends superior technical information with sensible purposes. His present endeavor is his thesis on “Enhancing Effectivity in Deep Reinforcement Studying,” showcasing his dedication to enhancing AI’s capabilities. Athar’s work stands on the intersection “Sparse Coaching in DNN’s” and “Deep Reinforcemnt Studying”.