The Rise of Imaginative and prescient Transformers. Is the period of ResNet coming to an finish? | by Nate Cibik
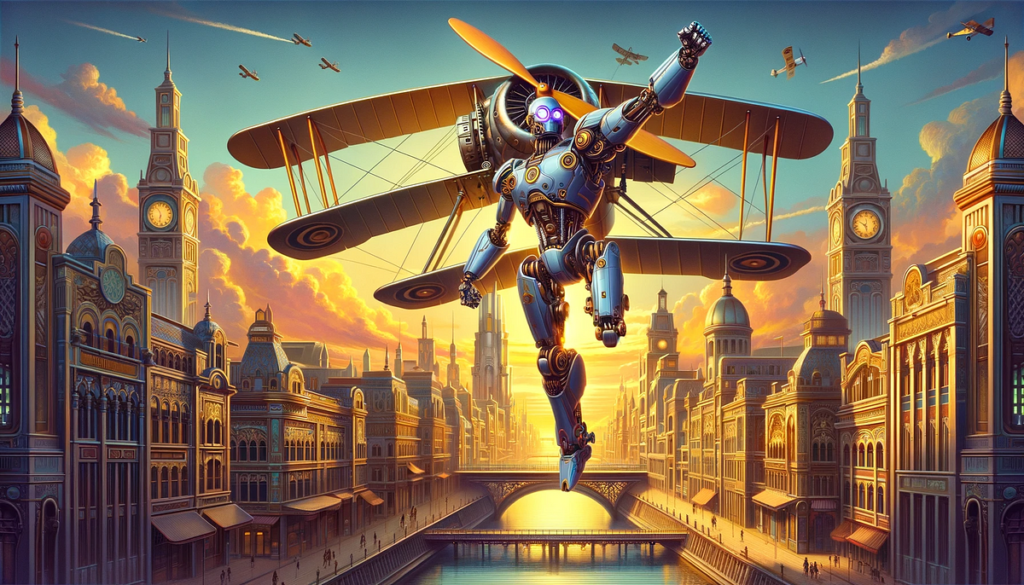
And so, it seems that the reply shouldn’t be a combat to the demise between CNNs and Transformers (see the numerous overindulgent eulogies for LSTMs), however relatively one thing a bit extra romantic. Not solely does the adoption of 2D convolutions in hierarchical transformers like CvT and PVTv2 conveniently create multiscale options, cut back the complexity of self-attention, and simplify structure by assuaging the necessity for positional encoding, however these fashions additionally make use of residual connections, one other inherited trait of their progenitors. The complementary strengths of transformers and CNNs have been introduced collectively in viable offspring.
So is the period of ResNet over? It will definitely appear so, though any paper will certainly want to incorporate this indefatigable spine for comparability for a while to come back. It is very important keep in mind, nonetheless, that there aren’t any losers right here, only a new era of highly effective and transferable characteristic extractors for all to get pleasure from, in the event that they know the place to look. Parameter environment friendly fashions like PVTv2 democratize analysis of extra advanced architectures by providing highly effective characteristic extraction with a small reminiscence footprint, and should be added to the record of ordinary backbones for benchmarking new architectures.
Future Work
This text has targeted on how the cross-pollination of convolutional operations and self-attention has given us the evolution of hierarchical characteristic transformers. These fashions have proven dominant efficiency and parameter effectivity at small scales, making them splendid characteristic extraction backbones (particularly in parameter-constrained environments). Nevertheless, there’s a lack of exploration into whether or not the efficiencies and inductive biases that these fashions capitalize on at smaller scales can switch to large-scale success and threaten the dominance of pure ViTs at a lot larger parameter counts.
Massive Multimodal Fashions (LMMS) like Massive Language and Visible Assistant (LLaVA) and different functions that require a pure language understanding of visible knowledge depend on Contrastive Language–Picture Pretraining (CLIP) embeddings generated from ViT-L options, and due to this fact inherit the strengths and weaknesses of ViT. If analysis into scaling hierarchical transformers reveals that their advantages, similar to multiscale options that improve fine-grained understanding, allow them to to attain higher or related efficiency with larger parameter effectivity than ViT-L, it might have widespread and instant sensible impression on something utilizing CLIP: LMMs, robotics, assistive applied sciences, augmented/digital actuality, content material moderation, training, analysis, and lots of extra functions affecting society and trade could possibly be improved and made extra environment friendly, decreasing the barrier for improvement and deployment of those applied sciences.