Unveiling Schrödinger’s Reminiscence: Dynamic Reminiscence Mechanisms in Transformer-Primarily based Language Fashions
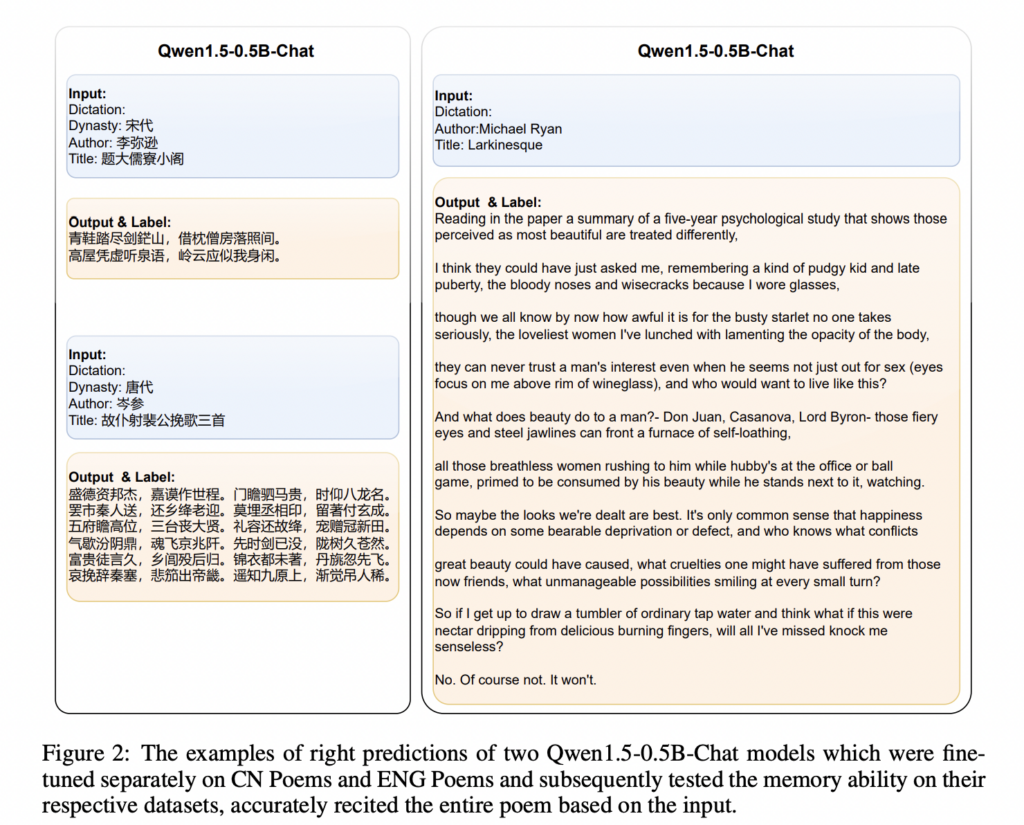
LLMs exhibit exceptional language skills, prompting questions on their reminiscence mechanisms. Not like people, who use reminiscence for every day duties, LLMs’ “reminiscence” is derived from enter somewhat than saved externally. Analysis efforts have aimed to enhance LLMs’ retention by extending context size and incorporating exterior reminiscence programs. Nevertheless, these strategies don’t totally make clear how reminiscence operates inside these fashions. The occasional provision of outdated data by LLMs signifies a type of reminiscence, although its exact nature is unclear. Understanding how LLM reminiscence differs from human reminiscence is crucial for advancing AI analysis and its functions.
Hong Kong Polytechnic College researchers use the Common Approximation Theorem (UAT) to elucidate reminiscence in LLMs. They suggest that LLM reminiscence, termed “Schrödinger’s reminiscence,” is just observable when queried, as its presence stays indeterminate in any other case. Utilizing UAT, they argue that LLMs dynamically approximate previous data primarily based on enter cues, resembling reminiscence. Their research introduces a brand new technique to evaluate LLM reminiscence skills and compares LLMs’ reminiscence and reasoning capacities to these of people, highlighting each similarities and variations. The research additionally offers theoretical and experimental proof supporting LLMs’ reminiscence capabilities.
The UAT types the premise of deep studying and explains reminiscence in Transformer-based LLMs. UAT exhibits that neural networks can approximate any steady operate. In Transformer fashions, this precept is utilized dynamically primarily based on enter knowledge. Transformer layers alter their parameters as they course of data, permitting the mannequin to suit features in response to completely different inputs. Particularly, the multi-head consideration mechanism modifies parameters to deal with and retain data successfully. This dynamic adjustment allows LLMs to exhibit memory-like capabilities, permitting them to recall and make the most of previous particulars when responding to queries.
The research explores the reminiscence capabilities of LLMs. First, it defines reminiscence as requiring each enter and output: reminiscence is triggered by enter, and the output could be right, incorrect, or forgotten. LLMs exhibit reminiscence by becoming enter to a corresponding output, very similar to human recall. Experiments utilizing Chinese language and English poem datasets examined fashions’ means to recite poems primarily based on minimal enter. Outcomes confirmed that bigger fashions with higher language understanding carried out considerably higher. Moreover, longer enter textual content lowered reminiscence accuracy, indicating a correlation between enter size and reminiscence efficiency.
The research argues that LLMs possess reminiscence and reasoning skills much like human cognition. Like people, LLMs dynamically generate outputs primarily based on realized data somewhat than storing fastened data. The researchers recommend that human brains and LLMs operate as dynamic fashions that alter to inputs, fostering creativity and adaptableness. Limitations in LLM reasoning are attributed to mannequin measurement, knowledge high quality, and structure. The mind’s dynamic becoming mechanism, exemplified by circumstances like Henry Molaison’s, permits for steady studying, creativity, and innovation, paralleling LLMs’ potential for complicated reasoning.
In conclusion, the research demonstrates that LLMs, supported by their Transformer-based structure, exhibit reminiscence capabilities much like human cognition. LLM reminiscence, termed “Schrödinger’s reminiscence,” is revealed solely when particular inputs set off it, reflecting the UAT in its dynamic adaptability. The analysis validates LLM reminiscence via experiments and compares it with human mind operate, discovering parallels of their dynamic response mechanisms. The research means that LLMs’ reminiscence operates like human reminiscence, turning into obvious solely via particular queries, and explores the similarities and variations between human and LLM cognitive processes.
Try the Paper. All credit score for this analysis goes to the researchers of this venture. Additionally, don’t neglect to observe us on Twitter and be part of our Telegram Channel and LinkedIn Group. For those who like our work, you’ll love our newsletter..
Don’t Overlook to hitch our 50k+ ML SubReddit