Source2Synth: A New AI Approach for Artificial Information Era and Curation Grounded in Actual Information Sources
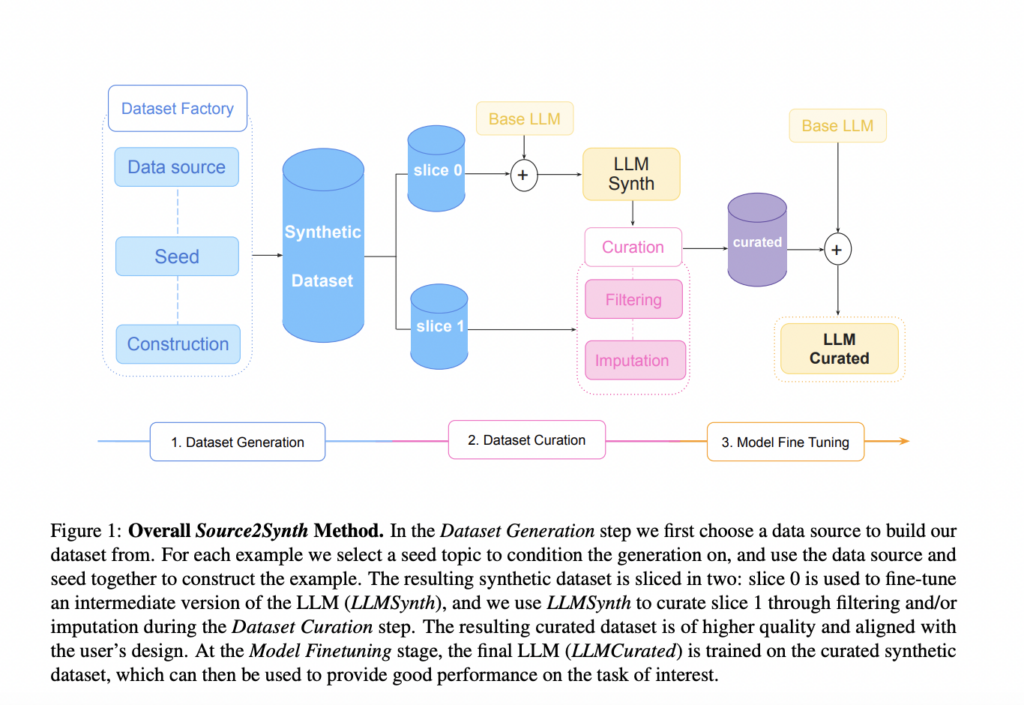
Massive Language Fashions (LLMs) have demonstrated spectacular efficiency in duties like Pure Language Processing, era, and textual content synthesis. Nonetheless, they nonetheless encounter main difficulties in additional difficult circumstances. These are assignments that decision for utilizing instruments to unravel issues, coping with structured knowledge, or finishing up complicated multi-step reasoning. As an example, though LLMs are adept at comprehending unstructured textual content, they’ve hassle using and decoding organized knowledge, resembling spreadsheets, tables, and databases. As well as, subpar efficiency is ceaselessly achieved on duties like multi-hop query answering (MHQA), which requires combining knowledge from a number of sources. Equally, LLMs nonetheless discover it difficult to finish duties that require the usage of instruments, together with utilizing SQL to reply tabular inquiries.
To beat these points, a brand new method referred to as Source2Synth has been launched by researchers from Meta, Oxford College, and College School London. The first good thing about Source2Synth is its capability to impart new expertise to LLMs with out the necessity for costly and time-consuming human annotations. Standard approaches to enhancing LLM efficiency ceaselessly name for quite a lot of guide annotation, which is dear and troublesome to scale, significantly for sophisticated jobs. This requirement has been eliminated by Source2Synth, which creates artificial knowledge that imitates precise conditions and thought processes.
So as to create artificial cases with intermediate reasoning steps, Source2Synth makes use of a particular knowledge supply, resembling tables from the web or related articles. Since these examples are primarily based on precise knowledge, the artificial knowledge is assured to be diversified, practical, and factually right. The strategy’s predominant step is making a seed matter, which may be an entity or a factual assertion, after which growing it right into a complete instance. The instance comprises the directions for the duty, the steps wanted to unravel the issue utilizing reasoning, and the answer. Via this process, Source2Synth is ready to generate intricate, practical knowledge factors that mimic the best way LLMs should deal with structured knowledge or perform multi-step actions.
The strategy that Source2Synth makes use of to reinforce dataset high quality is an integral part. Low-quality examples can deteriorate mannequin efficiency, and never all generated knowledge factors are equally invaluable. So as to deal with this, Source2Synth makes use of filtering methods decided by how answerable the artificial cases are. For instance, the instance is discarded if the generated knowledge doesn’t end in the appropriate response inside a sure variety of trials. This high quality management process ensures that solely wonderful examples, people who assist in the LLM’s acquisition of the mandatory expertise, are stored for the final spherical of fine-tuning.
The method has been applied in two distinctive and demanding fields, that are as follows,
- Multi-Hop Query Answering (MHQA): To answer a single query, the LLM on this area analyzes and synthesizes knowledge from a number of sources. When Source2Synth was evaluated on HotPotQA, a dataset created for multi-hop reasoning, it outperformed baseline fashions that have been adjusted by standard methods by 22.57%.
- Answering questions with structured knowledge is named tabular query answering (TQA), and it ceaselessly requires SQL queries to speak with tables. WikiSQL is a dataset that focuses on utilizing SQL to reply questions on tables. Source2Synth was examined on it and achieved a 25.51% enchancment over baseline fashions.
The outcomes have demonstrated how Source2Synth can improve LLM efficiency on difficult duties with out requiring massive quantities of human annotations on datasets. For coaching LLMs in domains requiring subtle reasoning and power utilization, Source2Synth provides a scalable methodology by producing grounded, practical examples and rigorously filtering the dataset to make sure top quality.
In conclusion, Source2Synth is a singular methodology for imparting new information to LLMs, significantly in conditions the place human annotation just isn’t possible. This technique solves the present constraints of LLMs in difficult duties like multi-step reasoning and structured knowledge manipulation by guaranteeing that solely high-quality examples are utilized for fine-tuning and by rooting artificial knowledge era in real-world sources for validation.
Try the Paper. All credit score for this analysis goes to the researchers of this venture. Additionally, don’t neglect to observe us on Twitter and be part of our Telegram Channel and LinkedIn Group. When you like our work, you’ll love our newsletter..
Don’t Neglect to affix our 50k+ ML SubReddit
Tanya Malhotra is a last 12 months undergrad from the College of Petroleum & Vitality Research, Dehradun, pursuing BTech in Laptop Science Engineering with a specialization in Synthetic Intelligence and Machine Studying.
She is a Information Science fanatic with good analytical and demanding considering, together with an ardent curiosity in buying new expertise, main teams, and managing work in an organized method.