Harmonics of Studying: A Mathematical Principle for the Rise of Fourier Options in Studying Techniques Like Neural Networks
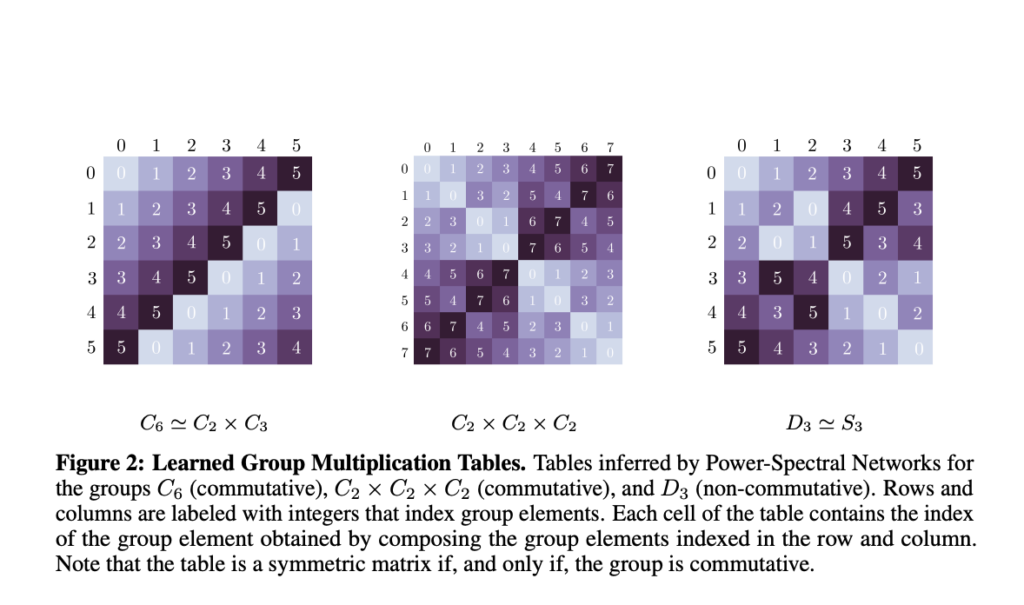
Synthetic neural networks (ANNs) present a outstanding sample when skilled on pure knowledge irrespective of actual initialization, dataset, or coaching goal; fashions skilled on the identical knowledge area converge to related discovered patterns. For instance, for various picture fashions, the preliminary layer weights are likely to converge to Gabor filters and color-contrast detectors. Many such options counsel international illustration that goes past organic and synthetic programs, and these options are noticed within the visible cortex. These findings are sensible and well-established within the discipline of machines that may interpret literature however lack theoretical explanations.
Localized variations of canonical 2D Fourier foundation features are probably the most noticed common options in picture fashions, e.g. Gabor filters or wavelets. When imaginative and prescient fashions are skilled on duties like environment friendly coding, classification, temporal coherence, and next-step prediction objectives, these Fourier options pop up within the mannequin’s preliminary layers. Other than this, Non-localized Fourier options have been noticed in networks skilled to unravel duties the place cyclic wraparound is allowed, for instance, modular arithmetic, extra normal group compositions, or invariance to the group of cyclic translations.
Researchers from KTH, Redwood Heart for Theoretical Neuroscience, and UC Santa Barbara launched a mathematical rationalization for the rise of Fourier options in studying programs like neural networks. This rise is as a result of downstream invariance of the learner that turns into insensitive to sure transformations, e.g., planar translation or rotation. The crew has derived theoretical ensures concerning Fourier options in invariant learners that can be utilized in several machine-learning fashions. This derivation relies on the idea that invariance is a basic bias that may be injected implicitly and typically explicitly into studying programs as a result of symmetries in pure knowledge.
The usual discrete Fourier remodel is a particular case of extra normal Fourier transforms on teams, which could be outlined by changing the idea of harmonics with totally different unitary group representations. A set of earlier theoretical works is fashioned for sparse coding fashions, deriving the situations below which sparse linear combos are used to recuperate the unique bases that generate knowledge with the assistance of a community. The proposed idea covers numerous conditions and neural community architectures that assist to set a basis for a studying idea of representations in synthetic and organic neural programs.
The crew gave two casual theorems on this paper, the primary one states that if a parametric perform of a sure type is invariant within the enter variable to the motion of a finite group G, then every element of its weights W coincides with a harmonic of G as much as a linear transformation. The second theorem states that if a parametric perform is sort of invariant to G in response to some useful bounds and the weights are orthonormal, then the multiplicative desk of G could be recovered from W. Furthermore, a mannequin is applied to fulfill the necessity of the proposed idea and skilled by means of totally different studying on a aim that helps invariance and extraction of the multiplicative desk of G from its weights.
In conclusion, researchers launched a mathematical rationalization for the rise of Fourier options in studying programs like neural networks. Additionally, they proved that if a machine studying mannequin of a particular type is invariant to a finite group, then its weights are intently associated to the Fourier remodel on that group, and the algebraic construction of an unknown group could be recovered from an invariant mannequin. Future work consists of the research of analogs of the proposed idea on actual numbers which is an fascinating space that will probably be aligned extra in the direction of the present practices within the discipline.
Take a look at the Paper. All credit score for this analysis goes to the researchers of this venture. Additionally, don’t overlook to comply with us on Twitter. Be part of our Telegram Channel, Discord Channel, and LinkedIn Group.
When you like our work, you’ll love our newsletter..
Don’t Overlook to affix our 42k+ ML SubReddit
Sajjad Ansari is a ultimate 12 months undergraduate from IIT Kharagpur. As a Tech fanatic, he delves into the sensible purposes of AI with a concentrate on understanding the affect of AI applied sciences and their real-world implications. He goals to articulate advanced AI ideas in a transparent and accessible method.