M-RewardBench: A Multilingual Strategy to Reward Mannequin Analysis, Analyzing Accuracy Throughout Excessive and Low-Useful resource Languages with Sensible Outcomes
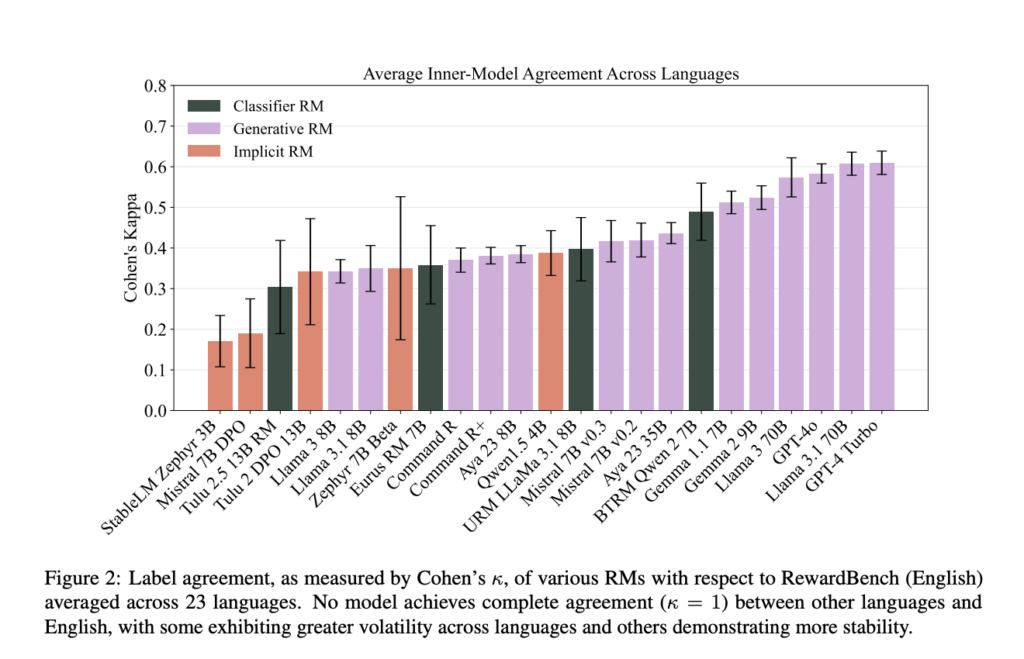
Giant language fashions (LLMs) have reworked fields starting from customer support to medical help by aligning machine output with human values. Reward fashions (RMs) play an necessary position on this alignment, basically serving as a suggestions loop the place fashions are guided to offer human-preferred responses. Whereas many developments have optimized these fashions for English, a broader problem exists in adapting RMs to multilingual contexts. This adaptation is important, given the worldwide consumer base that more and more depends on LLMs throughout various languages for numerous duties, together with on a regular basis data, security tips, and nuanced conversations.
A core situation in LLM improvement lies in adapting RMs to carry out persistently throughout totally different languages. Conventional reward fashions, primarily educated on English-language information, usually should catch up when prolonged to different languages. This limitation creates a efficiency hole that restricts these fashions’ applicability, significantly for non-English customers who rely upon language fashions for correct, culturally related, and protected responses. The present hole in RM capabilities underscores the necessity for multilingual benchmarks and analysis instruments to make sure fashions serve a worldwide viewers extra successfully.
Present analysis instruments, equivalent to RewardBench, give attention to assessing fashions in English for basic capabilities like reasoning, chat performance, and consumer security. Whereas this benchmark has established a baseline for evaluating English-based RMs, it should handle the multilingual dimensions needed for broader applicability. RewardBench, because it stands, doesn’t absolutely account for duties involving translation or cross-cultural responses. This highlights a essential space for enchancment, as correct translations and culturally aligned responses are foundational for a significant consumer expertise throughout totally different languages.
Researchers from Writesonic, Allen Institute for AI, Bangladesh College of Engineering and Know-how, ServiceNow, Cohere For AI Neighborhood, Cohere, and Cohere For AI developed the M-RewardBench, a brand new multilingual analysis benchmark designed to check RMs throughout a spectrum of 23 languages. The dataset, spanning 2,870 choice cases, consists of languages from eight distinctive scripts and a number of language households, offering a rigorous multilingual take a look at atmosphere. M-RewardBench goals to bridge the RM analysis hole by overlaying languages from diversified typological backgrounds, bringing new insights into how LLMs carry out throughout non-English languages in important areas equivalent to security, reasoning, chat functionality, and translation.
M-RewardBench methodology comprehensively evaluates multilingual reward fashions, using each machine-generated and human-verified translations for accuracy. The researchers crafted subsets based mostly on process issue and language complexity, translating and adapting RewardBench prompts throughout 23 languages. The benchmark consists of Chat, Chat-Onerous, Security, and Reasoning classes to evaluate RM’s capabilities in on a regular basis and complicated conversational settings. To measure the influence of translation high quality, the analysis staff used two translation methods, Google Translate and NLLB 3.3B, demonstrating that improved translation can considerably improve RM efficiency by as much as 3%.
The research revealed substantial efficiency disparities, significantly between English and non-English contexts. Generative reward fashions, equivalent to GPT-4-Turbo, carried out comparatively properly, attaining an 83.5% accuracy rating, whereas different RM sorts, equivalent to classifier-based fashions, struggled with the shift to multilingual duties. The outcomes point out that generative fashions are higher suited to multilingual alignment, though a median efficiency drop of 8% when transitioning from English to non-English duties stays. Additionally, the efficiency of fashions diversified considerably by language, with high-resource languages like Portuguese attaining the next accuracy (68.7%) in comparison with lower-resource languages like Arabic (62.8%).
A number of key insights emerged from M-RewardBench, underscoring areas for enchancment in multilingual RM improvement. For instance, RMs confirmed the next diploma of label consistency throughout languages for reasoning duties than for basic chat conversations, suggesting that sure forms of content material could also be extra adaptable to multilingual contexts. This perception factors to a necessity for specialised benchmarks inside M-RewardBench to judge several types of content material, particularly as fashions broaden into underrepresented languages with distinctive grammatical buildings.
Key Takeaways from the analysis:
- Dataset Scope: M-RewardBench spans 23 languages, eight language households, and a pair of,870 choice cases, making it one of the complete multilingual RM analysis instruments obtainable.
- Efficiency Gaps: Generative RMs achieved increased common scores, with a big 83.5% in multilingual settings, however general efficiency dropped by as much as 13% for non-English duties.
- Job-Particular Variations: Chat-Onerous duties confirmed the best efficiency degradation (5.96%), whereas reasoning duties had the least, highlighting that process complexity impacts RM accuracy throughout languages.
- Translation High quality Impression: Greater-quality translations improved RM accuracy by as much as 3%, emphasizing the necessity for refined translation strategies in multilingual contexts.
- Consistency in Excessive-Useful resource Languages: Fashions carried out higher in high-resource languages (e.g., Portuguese, 68.7%) and confirmed consistency points in lower-resource languages, equivalent to Arabic (62.8%).
- Benchmark Contribution: M-RewardBench supplies a brand new framework for assessing LLMs in non-English languages, setting a basis for future enhancements in RM alignment throughout cultural and linguistic contexts.
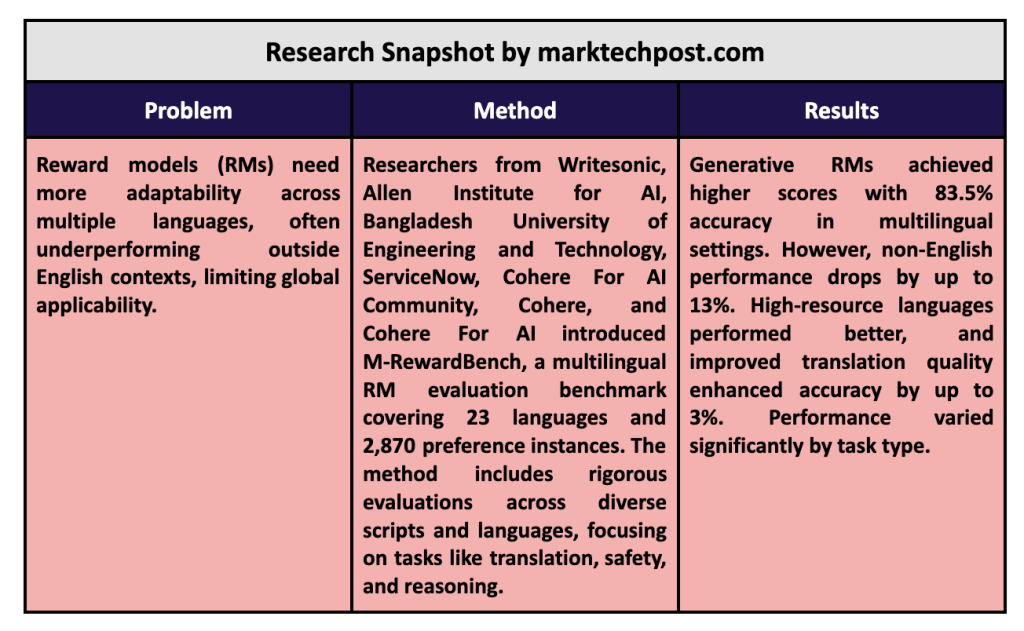
In conclusion, the analysis behind M-RewardBench illustrates a essential want for language fashions to align extra intently with human preferences throughout languages. By offering a benchmark tailor-made for multilingual contexts, this analysis lays the groundwork for future enhancements in reward modeling, particularly in dealing with cultural nuances and making certain language consistency. The findings reinforce the significance of growing RMs that reliably serve a worldwide consumer base, the place language variety and translation high quality are central to efficiency.
Try the Paper, Project, and GitHub. All credit score for this analysis goes to the researchers of this challenge. Additionally, don’t neglect to observe us on Twitter and be part of our Telegram Channel and LinkedIn Group. For those who like our work, you’ll love our newsletter.. Don’t Neglect to affix our 55k+ ML SubReddit.
[Upcoming Live Webinar- Oct 29, 2024] The Best Platform for Serving Fine-Tuned Models: Predibase Inference Engine (Promoted)
Asif Razzaq is the CEO of Marktechpost Media Inc.. As a visionary entrepreneur and engineer, Asif is dedicated to harnessing the potential of Synthetic Intelligence for social good. His most up-to-date endeavor is the launch of an Synthetic Intelligence Media Platform, Marktechpost, which stands out for its in-depth protection of machine studying and deep studying information that’s each technically sound and simply comprehensible by a large viewers. The platform boasts of over 2 million month-to-month views, illustrating its reputation amongst audiences.