Mechanistic Unlearning: A New AI Technique that Makes use of Mechanistic Interpretability to Localize and Edit Particular Mannequin Parts Related to Factual Recall Mechanisms
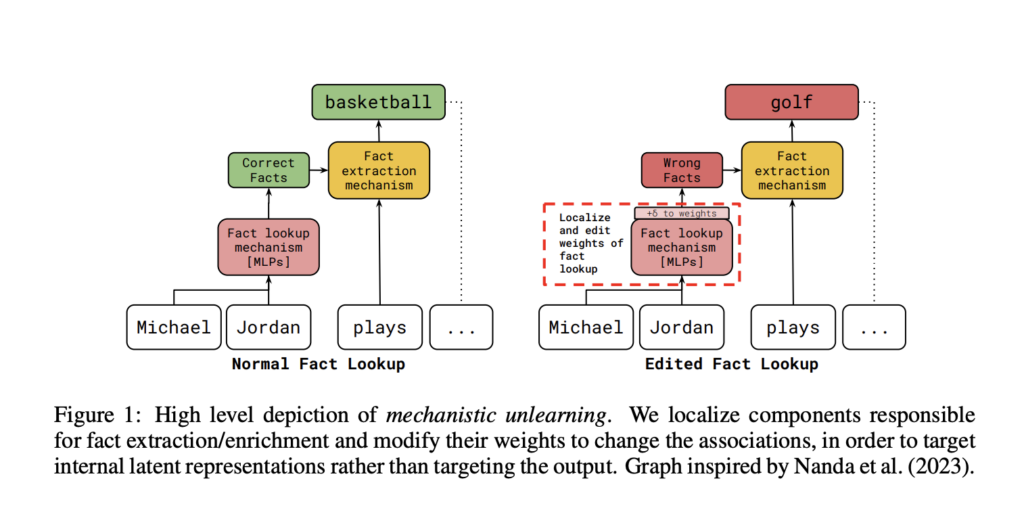
Giant language fashions (LLMs) typically study the issues that we don’t need them to study and perceive information. It’s necessary to seek out methods to take away or regulate this data to maintain AI correct, exact, and in management. Nonetheless, modifying or “unlearning” particular information in these fashions may be very powerful. The standard strategies to do that usually find yourself affecting different data or common data within the mannequin, which might have an effect on its total skills. Moreover, the adjustments made might not at all times final.
In latest works, researchers have used strategies like causal tracing to find key elements for output era, whereas quicker strategies like attribution patching assist pinpoint necessary elements extra rapidly. Enhancing and unlearning strategies attempt to take away or change sure data in a mannequin to maintain it secure and truthful. However typically, fashions can study again or present undesirable data. Present strategies for information modifying and unlearning usually have an effect on different capabilities of the mannequin and lack robustness, as slight variations in prompts can nonetheless elicit the unique information. Even with security measures, they may nonetheless produce dangerous responses to sure prompts, displaying that it’s nonetheless arduous to totally management their conduct.
A workforce of researchers from the College of Maryland, Georgia Institute of Expertise, College of Bristol, and Google DeepMind suggest Mechanistic unlearning. Mechanistic Unlearning is a brand new AI methodology that makes use of mechanistic interpretability to localize and edit particular mannequin elements related to factual recall mechanisms. This strategy goals to make edits extra sturdy and scale back unintended uncomfortable side effects.
The research examines strategies for eradicating data from AI fashions and finds that many fail when prompts or outputs shift. By concentrating on particular elements of fashions like Gemma-7B and Gemma-2-9B which might be accountable for truth retrieval, a gradient-based strategy proves simpler and environment friendly. This methodology reduces hidden reminiscence higher than others, requiring only some mannequin adjustments whereas generalizing throughout numerous knowledge. By concentrating on these elements, the tactic ensures that the undesirable information is successfully unlearned and resists relearning makes an attempt. The researchers reveal that this strategy results in extra sturdy edits throughout totally different enter/output codecs and reduces the presence of latent information in comparison with current strategies.
The researchers carried out experiments to check strategies for unlearning and modifying data in two datasets: Sports activities Info and CounterFact. Within the Sports activities Info dataset, they labored on eradicating associations with basketball athletes and altering the sports activities of 16 athletes to golf. Within the CounterFact dataset, they centered on swapping right solutions with incorrect ones for 16 info. They used two fundamental strategies: Output Tracing (which incorporates Causal Tracing and Attribution Patching) and Reality Lookup localization. The outcomes confirmed that guide localization led to higher accuracy and energy, particularly in multiple-choice exams. The tactic of guide interpretability was additionally robust towards makes an attempt to relearn the data. Moreover, evaluation of the underlying information recommended that efficient modifying makes it tougher to get well earlier data within the mannequin’s layers. Weight masking exams confirmed that optimization strategies largely change parameters associated to extracting info relatively than these used for trying up info, which emphasizes the necessity to enhance the very fact lookup course of for higher robustness. Thus, this strategy goals to make edits extra sturdy and scale back unintended uncomfortable side effects.
In conclusion, this paper presents a promising answer to the issue of strong information unlearning in LLMs through the use of Mechanistic interpretability to exactly goal and edit particular mannequin elements, thereby enhancing the effectiveness and robustness of the unlearning course of. The proposed work additionally suggests unlearning/modifying as a possible testbed for various interpretability strategies, which could sidestep the inherent lack of floor fact in interpretability.
Try the Paper. All credit score for this analysis goes to the researchers of this challenge. Additionally, don’t neglect to comply with us on Twitter and be a part of our Telegram Channel and LinkedIn Group. Should you like our work, you’ll love our newsletter.. Don’t Overlook to hitch our 55k+ ML SubReddit.
[Upcoming Live Webinar- Oct 29, 2024] The Best Platform for Serving Fine-Tuned Models: Predibase Inference Engine (Promoted)
Divyesh is a consulting intern at Marktechpost. He’s pursuing a BTech in Agricultural and Meals Engineering from the Indian Institute of Expertise, Kharagpur. He’s a Knowledge Science and Machine studying fanatic who needs to combine these main applied sciences into the agricultural area and remedy challenges.