Google DeepMind Researchers Suggest GenRM: Coaching Verifiers with Subsequent-Token Prediction to Leverage the Textual content Technology Capabilities of LLMs
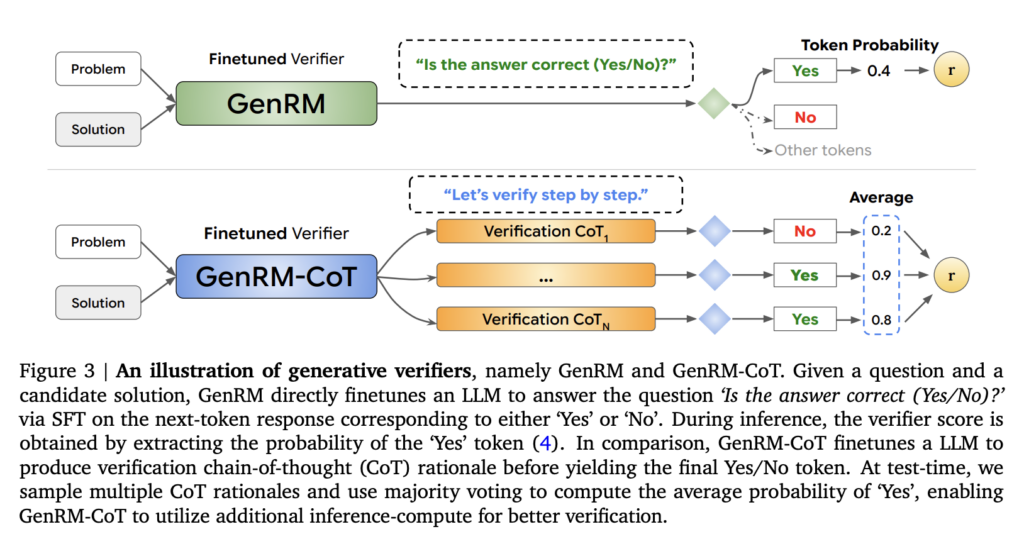
Generative AI, an space of synthetic intelligence, focuses on creating methods able to producing human-like textual content and fixing advanced reasoning duties. These fashions are important in varied functions, together with pure language processing. Their main operate is to foretell subsequent phrases in a sequence, generate coherent textual content, and even resolve logical and mathematical issues. Nevertheless, regardless of their spectacular capabilities, generative AI fashions usually need assistance with the accuracy and reliability of their outputs, which is especially problematic in reasoning duties the place a single error can invalidate a whole resolution.
One important subject inside this subject is the tendency of generative AI fashions to provide outputs that, whereas assured and convincing, might should be corrected. This problem is important in areas the place precision is paramount, similar to training, finance, and healthcare. The core of the issue lies within the fashions’ lack of ability to persistently generate appropriate solutions, which undermines their potential in high-stakes functions. Enhancing the accuracy and reliability of those AI methods is thus a precedence for researchers who goal to reinforce the trustworthiness of AI-generated options.
Current strategies to handle these points contain discriminative reward fashions (RMs), which classify potential solutions as appropriate or incorrect primarily based on their assigned scores. These fashions, nevertheless, want to completely leverage the generative skills of huge language fashions (LLMs). One other frequent method is the LLM-as-a-Decide methodology, the place pre-trained language fashions consider the correctness of options. Whereas this methodology faucets into the generative capabilities of LLMs, it usually fails to match the efficiency of extra specialised verifiers, significantly in reasoning duties requiring nuanced judgment.
Researchers from Google DeepMind, College of Toronto, MILA and UCLA have launched a novel method known as Generative Reward Modeling (GenRM). This methodology redefines the verification course of by framing it as a next-token prediction job, a basic functionality of LLMs. Not like conventional discriminative RMs, GenRM integrates the text-generation strengths of LLMs into the verification course of, permitting the mannequin to generate and consider potential options concurrently. This method additionally helps Chain-of-Thought (CoT) reasoning, the place the mannequin generates intermediate reasoning steps earlier than arriving at a closing determination. The GenRM methodology, subsequently, not solely assesses the correctness of options but additionally enhances the general reasoning course of by enabling extra detailed and structured evaluations.
The GenRM methodology employs a unified coaching method combining resolution era and verification. That is achieved by coaching the mannequin to foretell the correctness of an answer via next-token prediction, a way that leverages the inherent generative skills of LLMs. In follow, the mannequin generates intermediate reasoning steps—CoT rationales—that are then used to confirm the ultimate resolution. This course of integrates seamlessly with current AI coaching strategies, permitting for the simultaneous enchancment of era and verification capabilities. Moreover, the GenRM mannequin advantages from further inference-time computation, similar to majority voting aggregating a number of reasoning paths to reach on the most correct resolution.
The efficiency of the GenRM mannequin, significantly when paired with CoT reasoning, considerably surpasses conventional verification strategies. In a collection of rigorous assessments, together with duties associated to grade-school math and algorithmic problem-solving, the GenRM mannequin demonstrated a exceptional enchancment in accuracy. Particularly, the researchers reported a 16% to 64% improve within the share of appropriately solved issues in comparison with discriminative RMs and LLM-as-a-Decide strategies. For instance, when verifying outputs from the Gemini 1.0 Professional mannequin, the GenRM method improved the problem-solving success fee from 73% to 92.8%. This substantial efficiency enhance highlights the mannequin’s capacity to mitigate errors that normal verifiers usually overlook, significantly in advanced reasoning situations. Moreover, the researchers noticed that the GenRM mannequin scales successfully with elevated dataset measurement and mannequin capability, additional enhancing its applicability throughout varied reasoning duties.
In conclusion, the introduction of the GenRM methodology by researchers at Google DeepMind marks a major development in generative AI, significantly in addressing the verification challenges related to reasoning duties. The GenRM mannequin affords a extra dependable and correct method to fixing advanced issues by unifying resolution era and verification right into a single course of. This methodology improves the accuracy of AI-generated options and enhances the general reasoning course of, making it a useful device for future AI functions throughout a number of domains. As generative AI continues to evolve, the GenRM method offers a stable basis for additional analysis and improvement, significantly in areas the place precision and reliability are essential.
Take a look at the Paper. All credit score for this analysis goes to the researchers of this venture. Additionally, don’t neglect to observe us on Twitter and be a part of our Telegram Channel and LinkedIn Group. For those who like our work, you’ll love our newsletter..
Don’t Overlook to hitch our 50k+ ML SubReddit
Here’s a extremely beneficial webinar from our sponsor: ‘Building Performant AI Applications with NVIDIA NIMs and Haystack’
Nikhil is an intern marketing consultant at Marktechpost. He’s pursuing an built-in twin diploma in Supplies on the Indian Institute of Know-how, Kharagpur. Nikhil is an AI/ML fanatic who’s all the time researching functions in fields like biomaterials and biomedical science. With a powerful background in Materials Science, he’s exploring new developments and creating alternatives to contribute.