This AI Paper from Apple Introduces the Basis Language Fashions that Energy Apple Intelligence Options: AFM-on-Machine and AFM-Server
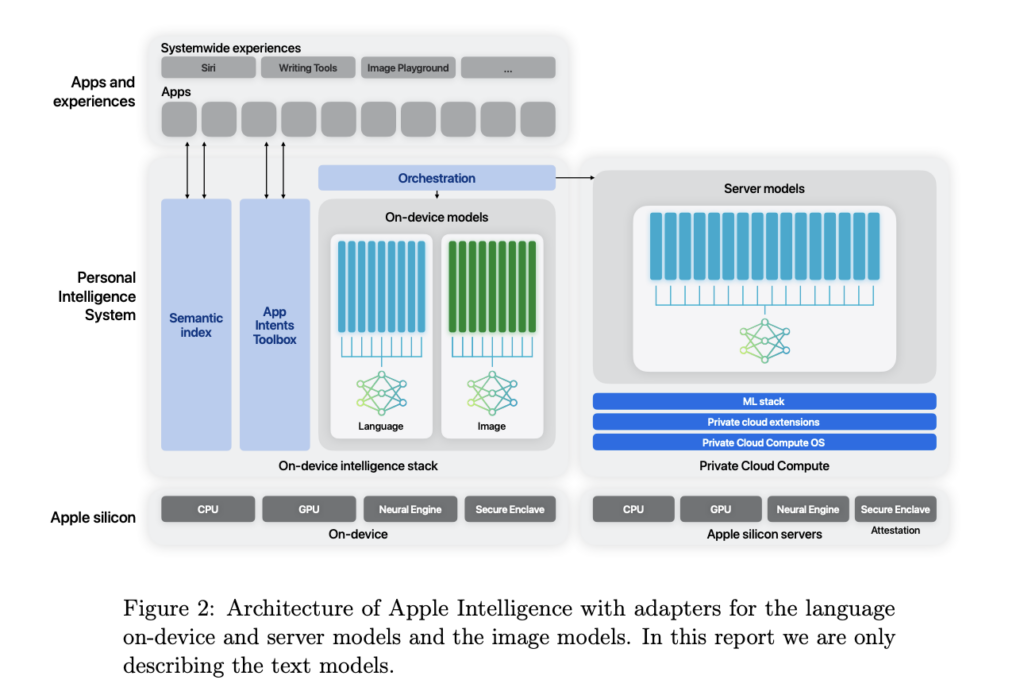
In AI, growing language fashions that may effectively and precisely carry out numerous duties whereas guaranteeing consumer privateness and moral concerns is a big problem. These fashions should deal with numerous knowledge sorts and functions with out compromising efficiency or safety. Making certain that these fashions function inside moral frameworks and preserve consumer belief provides one other layer of complexity to the duty.
Conventional AI fashions typically rely closely on huge server-based computations, resulting in challenges in effectivity and latency. Present strategies embrace numerous types of transformer architectures, that are neural networks designed for processing knowledge sequences. Mixed with subtle coaching processes and knowledge preprocessing methods, these architectures intention to enhance mannequin efficiency and reliability. Nonetheless, these strategies typically fall brief in balancing effectivity, accuracy, and moral concerns, particularly in real-time functions on private gadgets.
Researchers from Apple have launched two main language fashions: a 3 billion parameter mannequin optimized for on-device utilization and a bigger server-based mannequin designed for Apple’s Personal Cloud Compute. These fashions are crafted to steadiness effectivity, accuracy, and accountable AI rules, specializing in enhancing consumer experiences with out compromising on privateness and moral requirements. Introducing these fashions signifies a step in the direction of extra environment friendly and user-centric AI options.
The on-device mannequin employs pre-normalization with RMSNorm, grouped-query consideration with eight key-value heads, and SwiGLU activation for effectivity. RoPE positional embeddings assist long-context processing. The coaching utilized a various dataset combination, together with licensed knowledge from publishers, open-source datasets, and publicly obtainable internet knowledge. Pre-training was performed on 6.3 trillion tokens for the server mannequin and a distilled model for the on-device mannequin. The server mannequin underwent continued pre-training at a sequence size of 8192 with a mix that upweights math and code knowledge. The context-lengthening stage used sequences of 32768 tokens with artificial long-context Q&A knowledge. Publish-training concerned supervised fine-tuning (SFT) and reinforcement studying from human suggestions (RLHF) to boost instruction-following and conversational capabilities.
The efficiency of those fashions has been rigorously evaluated, demonstrating robust capabilities throughout numerous benchmarks. The on-device mannequin scored 61.4 on the HELM MMLU 5-shot benchmark, whereas the server mannequin scored 75.4. As well as, the server mannequin confirmed spectacular leads to GSM8K with a rating of 72.4, ARC-c with 69.7, and HellaSwag with 86.9. The AFM-server additionally excelled within the Winogrande benchmark with a rating of 79.2. These outcomes point out vital enhancements in instruction following, reasoning, and writing duties. Moreover, the analysis highlights a dedication to moral AI, with in depth measures taken to stop the perpetuation of stereotypes and biases, guaranteeing strong and dependable mannequin efficiency.
The analysis addresses the challenges of growing environment friendly and accountable AI fashions. The proposed strategies and applied sciences reveal vital developments in AI mannequin efficiency and moral concerns. These fashions supply helpful contributions to the sphere by specializing in effectivity and moral AI, showcasing how superior AI could be applied in user-friendly and accountable methods.
In conclusion, the paper offers a complete overview of Apple’s improvement and implementation of superior language fashions. It addresses the crucial downside of balancing effectivity, accuracy, and moral concerns in AI. The researchers’ proposed strategies considerably enhance mannequin efficiency whereas specializing in consumer privateness and accountable AI rules. This work represents a big development within the subject, providing a strong framework for future AI developments.
Take a look at the Paper. All credit score for this analysis goes to the researchers of this undertaking. Additionally, don’t overlook to observe us on Twitter and be part of our Telegram Channel and LinkedIn Group. If you happen to like our work, you’ll love our newsletter..
Don’t Overlook to hitch our 47k+ ML SubReddit
Discover Upcoming AI Webinars here
Nikhil is an intern advisor at Marktechpost. He’s pursuing an built-in twin diploma in Supplies on the Indian Institute of Know-how, Kharagpur. Nikhil is an AI/ML fanatic who’s all the time researching functions in fields like biomaterials and biomedical science. With a powerful background in Materials Science, he’s exploring new developments and creating alternatives to contribute.