Enhancing Advertising and marketing Combine Modelling with Causal AI | by Ryan O’Sullivan | Jun, 2024
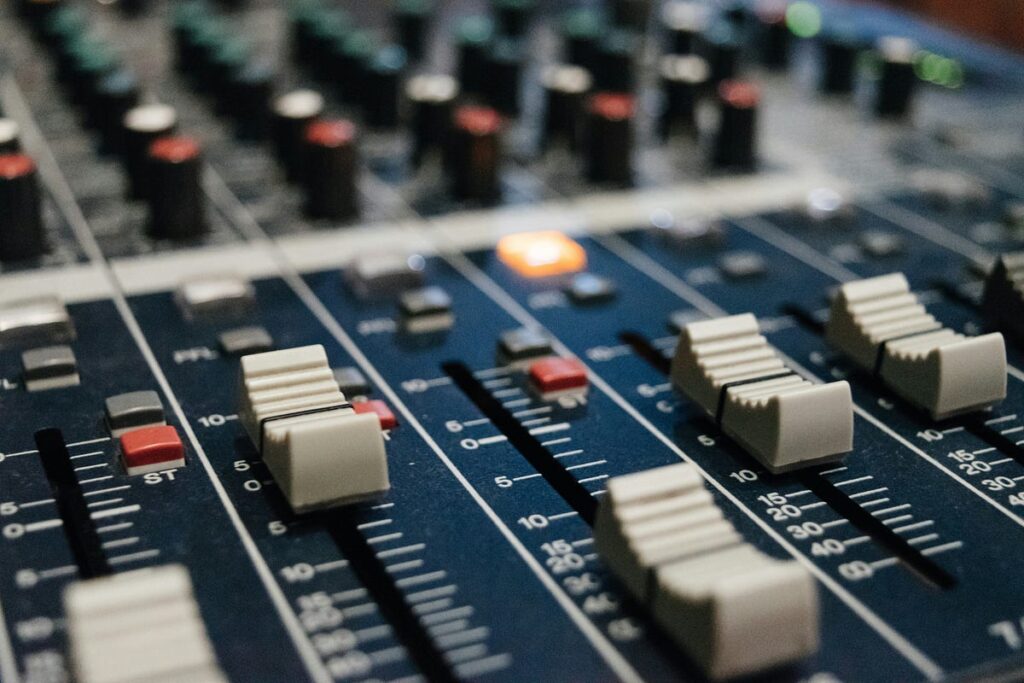
Welcome to my collection on Causal AI, the place we’ll discover the mixing of causal reasoning into machine studying fashions. Anticipate to discover quite a lot of sensible functions throughout completely different enterprise contexts.
Within the final article we lined validating the causal influence of the artificial management methodology. On this article we’ll transfer onto enhancing advertising and marketing combine modelling with Causal AI.
In case you missed the final article on artificial controls, test it out right here:
Ongoing challenges with digital monitoring has led to a latest resurgence in advertising and marketing combine modelling (MMM). On the latest Causal AI convention, Judea Pearl prompt that advertising and marketing often is the first business to undertake Causal AI. So I made a decision it was time begin writing about my learnings from the final 7 years by way of how MMM, Causal AI and experimentation intersect.
The next areas will likely be explored:
- What’s MMM?
- How can Causal AI improve MMM?
- What experiments can we run to finish the triangulation?
- Excellent challenges inside advertising and marketing measurement.
The total pocket book could be discovered right here:
MMM is a statistical framework used to estimate how a lot every advertising and marketing channel contributes to gross sales. It’s closely influenced by econometrics and in its easiest kind is a regression mannequin. Let’s cowl the fundamentals of the important thing elements!
Regression
A regression mannequin is constructed the place the dependent variable/goal (often gross sales) is predicted based mostly on a number of impartial variables/options — These often embody the spend on completely different advertising and marketing channels and exterior components that will impact demand.
The coefficients of the spend variables point out how a lot they contribute to gross sales.
The PyMC advertising and marketing package deal in python is a superb place to start out exploring MMM:
Advert inventory
Advert inventory refers back to the lingering impact of promoting spend (or adverting spend) on client behaviour. It helps mannequin the long-term results of promoting. It’s not frequent behaviour to hurry to buy a product the primary time you hear a few model — the concept of advert inventory is that the impact of promoting is cumulative.
The commonest advert inventory methodology is geometric decay, which assumes that the influence of promoting decays at a continuing fee over time. Though that is comparatively simple to implement, it isn’t very versatile. It’s value trying out the Weibull methodology which is far more versatile — The PyMC advertising and marketing package deal has carried out it so you should definitely test it out:
Saturation
Saturation within the context of promoting refers back to the thought of diminishing returns. Growing advertising and marketing spend can enhance buyer acquisition, however as time goes on it turns into tougher to affect new audiences.
There are a number of saturation strategies we may use. The Michaelis-Menton operate is a standard one — You can too verify this out within the PyMC advertising and marketing package deal:
MMM frameworks often use a flat regression mannequin. Nonetheless, there are some complexities to how advertising and marketing channels work together with one another. Is there a software from our Causal AI toolbox which may also help with this?
Causal graphs
Causal graphs are nice at disentangling causes from correlations which make them an excellent software for coping with the complexities of how advertising and marketing channels work together with one another.
If you’re unfamiliar with causal graphs, use my earlier article to rise up to hurry:
Understanding the advertising and marketing graph
Estimating the causal graph in conditions the place you’ve got poor area information accessible is difficult. However we will use causal discovery to assist get us began – Take a look at my earlier article on causal discovery to seek out out extra:
Causal discovery has its limitations and may simply be used to create a beginning speculation for the graph. Fortunately, there’s a huge quantity of area information round how advertising and marketing channels work together with one another that we will construct in!
Under I share the information I’ve picked up from working with advertising and marketing specialists through the years…
- PPC (paid search) has a damaging impact on search engine optimization (natural search). The extra we spend on PPC the much less search engine optimization clicks we get. Nonetheless, we’ve an essential confounder….demand! A flat regression mannequin won’t choose up this intricacy usually resulting in an overestimation of PPC.
- Social spend has a robust impact on social clicks, the extra we spend the extra prospects click on on social advertisements. Nonetheless, some prospects could view an social advert and the following day go to your web site by way of PPC, search engine optimization or Direct. A flat regression mannequin won’t choose up this halo impact.
- An identical case could be made for model spend, the place you goal prospects with long term branding messages however no direct name to motion to click on. These prospects could go to your web site by way of PPC, search engine optimization or Direct at a later stage after turning into conscious of your model.
- The clicks are mediators. If we run a flat regression and embody mediators, this may trigger points when estimating causal results. I received’t cowl this matter in an excessive amount of element right here, however utilizing causal graphs permits us to fastidiously management for the appropriate variables when estimating causal results.
Hopefully you’ll be able to see from the examples above that utilizing a causal graph as an alternative of a flat regression will critically improve your resolution. The flexibility to calculate counterfactuals and carry out interventions additionally make it very engaging!
It’s value noting that it’s nonetheless value incorporating the advert inventory and saturation transformations into your framework.
When working with observational knowledge, we must also be striving to run experiments to assist validate assumptions and complement our causal estimates. There are three most important checks accessible to make use of in acquisition advertising and marketing. Let’s dive into them!
Conversion elevate checks
Social platforms like Fb and Snapchat let you run conversion elevate checks. That is an AB take a look at the place we measure the uplift in conversion utilizing a remedy vs management group. These could be very helpful on the subject of evaluating the counterfactual out of your causal graph for social spend.
Geo elevate checks
Geo elevate checks can be utilized to estimate the impact of promoting blackouts or if you begin utilizing a brand new channel. This may be notably helpful for model digital and TV the place there isn’t any direct name to motion to measure. I cowl this in far more element within the final article:
Change again testing
PPC campaigns could be scheduled to be turned on and off hourly. This creates an excellent alternative for switchback testing. Schedule PPC campaigns to be turned on and off every hour for just a few weeks, after which calculate the distinction between the variety of PPC + search engine optimization clicks within the off vs on interval. It will assist you to perceive how a lot of PPC could be captured by search engine optimization, and subsequently consider the counterfactual out of your causal graphs for PPC spend.
I believe operating experiments is an effective way to tweak after which achieve confidence in your causal graph. However outcomes is also used to calibrate your mannequin. Check out how the PyMC group have approached this:
Immediately I went into how one can improve MMM with Causal AI. Nonetheless, Causal AI can’t remedy all the challenges inside acquisition advertising and marketing— And there are many them sadly!
- Spend following the demand forecast — One motive for advertising and marketing spend being extremely correlated with gross sales quantity could be right down to the advertising and marketing group spending in-line with a requirement forecast. One resolution right here is to randomly shift spend by -10% to +10% every week so as to add some variation. As you’ll be able to think about, the advertising and marketing group often aren’t too eager on this strategy!
- Estimating demand — Demand is a necessary variable in our mannequin. Nonetheless, it may be very tough to gather knowledge on. An affordable choice is extracting google development knowledge on a search time period which aligns to the product you might be promoting.
- Long run results of name — Long run results of name are exhausting to seize as there often isn’t a lot sign round this. Long run geo elevate checks may also help right here.
- Multi-collinearity — That is truly one of many largest issues. All the variables we’ve are extremely correlated. Utilizing ridge regression can alleviate this a bit, however it will possibly nonetheless be an issue. A causal graph may also help a bit too because it important breaks the issue down into smaller fashions.
- Purchase-in from the advertising and marketing group — In my expertise this will likely be your largest problem. Causal graphs provide a pleasant visible approach of participating the advertising and marketing group. It additionally creates a possibility so that you can construct up a relationship while working with them to agree the intricacies of the graph.
I’ll shut issues off there — It could be nice to listen to what you assume within the feedback!