Establishing an AI/ML middle of excellence
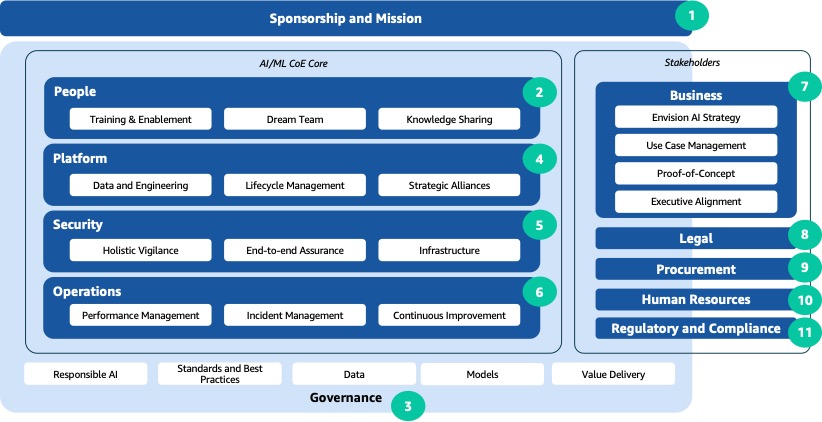
The speedy developments in synthetic intelligence and machine studying (AI/ML) have made these applied sciences a transformative power throughout industries. In response to a McKinsey study, throughout the monetary companies {industry} (FSI), generative AI is projected to ship over $400 billion (5%) of {industry} income in productiveness advantages. As maintained by Gartner, greater than 80% of enterprises can have AI deployed by 2026. At Amazon, we imagine innovation (rethink and reinvent) drives improved buyer experiences and environment friendly processes, resulting in elevated productiveness. Generative AI is a catalyst for enterprise transformation, making it crucial for FSI organizations to determine where generative AI’s current capabilities could deliver the largest worth for FSI clients.
Organizations throughout industries face numerous challenges implementing generative AI throughout their group, equivalent to lack of clear enterprise case, scaling past proof of idea, lack of governance, and availability of the correct expertise. An efficient strategy that addresses a variety of noticed points is the institution of an AI/ML middle of excellence (CoE). An AI/ML CoE is a devoted unit, both centralized or federated, that coordinates and oversees all AI/ML initiatives inside a company, bridging enterprise technique to worth supply. As noticed by Harvard Enterprise Evaluate, an AI/ML CoE is already established in 37% of large companies within the US. For organizations to achieve success of their generative AI journey, there may be rising significance for coordinated collaboration throughout strains of companies and technical groups.
This put up, together with the Cloud Adoption Framework for AI/ML and Well-Architected Machine Learning Lens, serves as a information for implementing an efficient AI/ML CoE with the target to seize generative AI’s prospects. This consists of guiding practitioners to outline the CoE mission, forming a management workforce, integrating moral tips, qualification and prioritization of use circumstances, upskilling of groups, implementing governance, creating infrastructure, embedding safety, and enabling operational excellence.
What’s an AI/ML CoE?
The AI/ML CoE is answerable for partnering with strains of enterprise and end-users in figuring out AI/ML use circumstances aligned to enterprise and product technique, recognizing widespread reusable patterns from totally different enterprise models (BUs), implementing a company-wide AI/ML imaginative and prescient, and deploying an AI/ML platform and workloads on probably the most applicable mixture of computing {hardware} and software program. The CoE workforce synergizes enterprise acumen with profound technical AI/ML proficiency to develop and implement interoperable, scalable options all through the group. They set up and implement greatest practices encompassing design, growth, processes, and governance operations, thereby mitigating dangers and ensuring strong enterprise, technical, and governance frameworks are constantly upheld. For ease of consumption, standardization, scalability, and worth supply, the outputs of an AI/ML CoE could be of two sorts: steerage equivalent to revealed steerage, greatest practices, classes realized, and tutorials, and capabilities equivalent to individuals expertise, instruments, technical options, and reusable templates.
The next are advantages of building an AI/ML CoE:
- Quicker time to market via a transparent path to manufacturing
- Maximized return on investments via delivering on the promise of generative AI enterprise outcomes
- Optimized threat administration
- Structured upskilling of groups
- Sustainable scaling with standardized workflows and tooling
- Higher assist and prioritization of innovation initiatives
The next determine illustrates the important thing parts for establishing an efficient AI/ML CoE.
Within the following sections, we focus on every numbered element intimately.
1. Sponsorship and mission
The foundational step in organising an AI/ML CoE is securing sponsorship from senior management, establishing management, defining its mission and targets, and aligning empowered management.
Set up sponsorship
Set up clear management roles and construction to offer decision-making processes, accountability, and adherence to moral and authorized requirements:
- Govt sponsorship – Safe assist from senior management to champion AI/ML initiatives
- Steering committee – Kind a committee of key stakeholders to supervise the AI/ML CoE’s actions and strategic course
- Ethics board – Create a board to handle moral and accountable AI issues in AI/ML growth and deployment
Outline the mission
Making the mission customer- or product-focused and aligned with the group’s total strategic objectives helps define the AI/ML CoE’s function in reaching them. This mission, often set by the manager sponsor in alignment with the heads of enterprise models, serves as a tenet for all CoE actions, and incorporates the next:
- Mission assertion – Clearly articulate the aim of the CoE in advancing buyer and product outcomes making use of AI/ML applied sciences
- Strategic targets – Define tangible and measurable AI/ML objectives that align with the group’s total strategic objectives
- Worth proposition – Quantify the anticipated enterprise worth Key Efficiency Indicators (KPIs) equivalent to price financial savings, income good points, person satisfaction, time financial savings, and time-to-market
2. Individuals
In response to a Gartner report, 53% of enterprise, practical, and technical groups price their technical acumen on generative AI as “Intermediate” and 64% of senior management price their ability as “Novice.” By growing personalized options tailor-made to the particular and evolving wants of the enterprise, you’ll be able to foster a tradition of steady development and studying and domesticate a deep understanding of AI and ML applied sciences, together with generative AI ability growth and enablement.
Coaching and enablement
To assist educate staff on AI/ML ideas, instruments, and methods, the AI/ML CoE can develop coaching applications, workshops, certification programs, and hackathons. These applications could be tailor-made to totally different ranges of experience and designed to assist staff perceive tips on how to use AI/ML to unravel enterprise issues. Moreover, the CoE might present a mentoring platform to staff who’re fascinated with additional enhancing their AI/ML expertise, develop certification applications to acknowledge staff who’ve achieved a sure degree of proficiency in AI/ML, and supply ongoing coaching to maintain the workforce up to date with the most recent applied sciences and methodologies.
Dream workforce
Cross-functional engagement is important to realize well-rounded AI/ML options. Having a multidisciplinary AI/ML CoE that mixes {industry}, enterprise, technical, compliance, and operational experience helps drive innovation. It harnesses the 360 view potential of AI in reaching an organization’s strategic enterprise objectives. Such a various workforce with AI/ML experience might embody roles equivalent to:
- Product strategists – Be sure that all merchandise, options, and experiments are cohesive to the general transformation technique
- AI researchers – Make use of specialists within the area to drive innovation and discover cutting-edge methods equivalent to generative AI
- Knowledge scientists and ML engineers – Develop capabilities for information preprocessing, mannequin coaching, and validation
- Area specialists – Collaborate with professionals from enterprise models who perceive the particular functions and enterprise want
- Operations – Develop KPIs, display worth supply, and handle machine studying operations (MLOPs) pipelines
- Challenge managers – Appoint venture managers to implement initiatives effectively
Data sharing
By fostering collaboration inside the CoE, inside stakeholders, enterprise unit groups, and exterior stakeholders, you’ll be able to allow data sharing and cross-disciplinary teamwork. Encourage data sharing, set up a data repository, and facilitate cross-functional initiatives to maximise the affect of AI/ML initiatives. Some instance key actions to foster data sharing are:
- Cross-functional collaborations – Promote teamwork between specialists in generative AI and enterprise unit domain-specific professionals to innovate on cross-functional use circumstances
- Strategic partnerships – Examine partnerships with analysis establishments, universities, and {industry} leaders specializing in generative AI to benefit from their collective experience and insights
3. Governance
Set up governance that permits the group to scale worth supply from AI/ML initiatives whereas managing threat, compliance, and safety. Moreover, pay particular consideration to the altering nature of the danger and value that’s related to the event in addition to the scaling of AI.
Accountable AI
Organizations can navigate potential moral dilemmas related to generative AI by incorporating issues equivalent to equity, explainability, privateness and safety, robustness, governance, and transparency. To supply ethical integrity, an AI/ML CoE helps combine strong tips and safeguards throughout the AI/ML lifecycle in collaboration with stakeholders. By taking a proactive approach, the CoE supplies moral compliance but in addition builds belief, enhances accountability, and mitigates potential dangers equivalent to veracity, toxicity, information misuse, and mental property issues.
Requirements and greatest practices
Persevering with its stride in direction of excellence, the CoE helps outline widespread requirements, industry-leading practices, and tips. These embody a holistic strategy, overlaying information governance, mannequin growth, moral deployment, and ongoing monitoring, reinforcing the group’s dedication to accountable and moral AI/ML practices. Examples of such requirements embody:
- Growth framework – Establishing standardized frameworks for AI growth, deployment, and governance supplies consistency throughout initiatives, making it simpler to undertake and share greatest practices.
- Repositories – Centralized code and mannequin repositories facilitate the sharing of greatest practices and industry standard solutions in coding requirements, enabling groups to stick to constant coding conventions for higher collaboration, reusability, and maintainability.
- Centralized data hub – A central repository housing datasets and analysis discoveries to function a complete data middle.
- Platform – A central platform equivalent to Amazon SageMaker for creation, coaching, and deployment. It helps handle and scale central insurance policies and requirements.
- Benchmarking and metrics – Defining standardized metrics and benchmarking to measure and examine the efficiency of AI fashions, and the enterprise worth derived.
Knowledge governance
Data governance is an important operate of an AI/ML CoE, equivalent to ensuring information is collected, used, and shared in a accountable and reliable method. Knowledge governance is important for AI functions, as a result of these functions typically use giant quantities of information. The standard and integrity of this information are crucial to the accuracy and equity of AI-powered choices. The AI/ML CoE helps outline best practices and guidelines for information preprocessing, mannequin growth, coaching, validation, and deployment. The CoE ought to guarantee that information is correct, full, and up-to-date; the info is protected against unauthorized entry, use, or disclosure; and information governance insurance policies display the adherence to regulatory and inside compliance.
Mannequin oversight
Mannequin governance is a framework that determines how an organization implements insurance policies, controls entry to fashions, and tracks their exercise. The CoE helps guarantee that fashions are developed and deployed in a secure, reliable, and moral style. Moreover, it might probably affirm that mannequin governance insurance policies display the group’s dedication to transparency, fostering belief with clients, companions, and regulators. It could possibly additionally present safeguards personalized to your software necessities and ensure accountable AI insurance policies are applied utilizing companies equivalent to Guardrails for Amazon Bedrock.
Worth supply
Handle the AI/ML initiative return on funding, platform and companies bills, environment friendly and efficient use of sources, and ongoing optimization. This requires monitoring and analyzing use case-based worth KPIs and expenditures associated to information storage, mannequin coaching, and inference. This consists of assessing the efficiency of assorted AI fashions and algorithms to establish cost-effective, resource-optimal options equivalent to utilizing AWS Inferentia for inference and AWS Trainium for coaching. Setting KPIs and metrics is pivotal to gauge effectiveness. Some instance KPIs are:
- Return on funding (ROI) – Evaluating monetary returns towards investments justifies useful resource allocation for AI initiatives
- Enterprise affect – Measuring tangible enterprise outcomes like income uplift or enhanced buyer experiences validates AI’s worth
- Challenge supply time – Monitoring time from venture initiation to completion showcases operational effectivity and responsiveness
4. Platform
The AI/ML CoE, in collaboration with the enterprise and expertise groups, may help construct an enterprise-grade and scalable AI platform, enabling organizations to function AI-enabled companies and merchandise throughout enterprise models. It could possibly additionally assist develop customized AI options and assist practitioners adapt to alter in AI/ML growth.
Knowledge and engineering structure
The AI/ML CoE helps arrange the correct information flows and engineering infrastructure, in collaboration with the expertise groups, to speed up the adoption and scaling of AI-based options:
- Excessive-performance computing sources – Highly effective GPUs equivalent to Amazon Elastic Compute Cloud (Amazon EC2) instances, powered by the most recent NVIDIA H100 Tensor Core GPUs, are important for coaching complicated fashions.
- Knowledge storage and administration – Implement strong information storage, processing, and administration techniques equivalent to AWS Glue and Amazon OpenSearch Service.
- Platform – Utilizing cloud platforms can present flexibility and scalability for AI/ML initiatives for duties equivalent to SageMaker, which may help present end-to-end ML functionality throughout generative AI experimentation, information prep, mannequin coaching, deployment, and monitoring. This additional helps speed up generative AI workloads from experimentation to manufacturing. Amazon Bedrock is a neater solution to construct and scale generative AI functions with basis fashions (FMs). As a totally managed service, it provides a selection of high-performing FMs from main AI firms together with AI21 Labs, Anthropic, Cohere, Meta, Stability AI, and Amazon.
- Growth instruments and frameworks – Use industry-standard AI/ML frameworks and instruments equivalent to Amazon CodeWhisperer, Apache MXNet, PyTorch, and TensorFlow.
- Model management and collaboration instruments – Git repositories, venture administration instruments, and collaboration platforms can facilitate teamwork, equivalent to AWS CodePipeline and Amazon CodeGuru.
- Generative AI frameworks – Make the most of state-of-the-art basis fashions, instruments, brokers, data bases, and guardrails out there on Amazon Bedrock.
- Experimentation platforms – Deploy platforms for experimentation and mannequin growth, permitting for reproducibility and collaboration, equivalent to Amazon SageMaker JumpStart.
- Documentation – Emphasize the documentation of processes, workflows, and greatest practices inside the platform to facilitate data sharing amongst practitioners and groups.
Lifecycle administration
Inside the AI/ML CoE, the emphasis on scalability, availability, reliability, efficiency, and resilience is prime to the success and flexibility of AI/ML initiatives. Implementation and operationalization of a lifecycle administration system equivalent to MLOps may help automate deployment and monitoring, leading to improved reliability, time to market, and observability. Utilizing instruments like Amazon SageMaker Pipelines for workflow administration, Amazon SageMaker Experiments for managing experiments, and Amazon Elastic Kubernetes Service (Amazon EKS) for container orchestration permits adaptable deployment and administration of AI/ML functions, fostering scalability and portability throughout numerous environments. Equally, using serverless architectures equivalent to AWS Lambda empowers automated scaling primarily based on demand, decreasing operational complexity whereas providing flexibility in useful resource allocation.
Strategic alliances in AI companies
The choice to purchase or construct options includes trade-offs. Shopping for provides velocity and comfort by utilizing pre-built instruments, however might lack customization. Alternatively, constructing supplies tailor-made options however calls for time and sources. The stability hinges on the venture scope, timeline, and long-term wants, reaching optimum alignment with organizational objectives and technical necessities. The choice, ideally, could be primarily based on a radical evaluation of the particular downside to be solved, the group’s inside capabilities, and the realm of the enterprise focused for development. For instance, if the enterprise system helps set up uniqueness after which builds to distinguish available in the market, or if the enterprise system helps a regular commoditized enterprise course of, then buys to save lots of.
By partnering with third-party AI service suppliers, equivalent to AWS Generative AI Competency Partners, the CoE can use their experience and expertise to speed up the adoption and scaling of AI-based options. These partnerships may help the CoE keep updated with the most recent AI/ML analysis and traits, and might present entry to cutting-edge AI/ML instruments and applied sciences. Moreover, third-party AI service suppliers may help the CoE establish new use circumstances for AI/ML and might present steerage on tips on how to implement AI/ML options successfully.
5. Safety
Emphasize, assess, and implement safety and privateness controls throughout the group’s information, AI/ML, and generative AI workloads. Combine safety measures throughout all facets of AI/ML to establish, classify, remediate, and mitigate vulnerabilities and threats.
Holistic vigilance
Primarily based on how your group is utilizing generative AI options, scope the security efforts, design resiliency of the workloads, and apply relevant security controls. This consists of using encryption methods, multifactor authentication, risk detection, and common safety audits to verify information and techniques stay protected towards unauthorized entry and breaches. Common vulnerability assessments and risk modeling are essential to handle rising threats. Methods equivalent to mannequin encryption, utilizing safe environments, and steady monitoring for anomalies may help defend towards adversarial assaults and malicious misuse. To watch the mannequin for threats detection, you should use instruments like Amazon GuardDuty. With Amazon Bedrock, you have got full management over the info you utilize to customise the muse fashions to your generative AI functions. Knowledge is encrypted in transit and at relaxation. Consumer inputs and mannequin outputs should not shared with any mannequin suppliers; preserving your information and functions safe and personal.
Finish-to-end assurance
Imposing the safety of the three crucial parts of any AI system (inputs, mannequin, and outputs) is crucial. Establishing clearly outlined roles, safety insurance policies, requirements, and tips throughout the lifecycle may help handle the integrity and confidentiality of the system. This consists of implementation of industry best practice measures and {industry} frameworks, equivalent to NIST, OWASP-LLM, OWASP-ML, MITRE Atlas. Moreover, evaluate and implement necessities equivalent to Canada’s Private Info Safety and Digital Paperwork Act (PIPEDA) and European Union’s Normal Knowledge Safety Regulation (GDPR). You should use instruments equivalent to Amazon Macie to find and defend your delicate information.
Infrastructure (information and techniques)
Given the sensitivity of the info concerned, exploring and implementing entry and privacy-preserving methods is important. This includes methods equivalent to least privilege entry, information lineage, preserving solely related information to be used case, and figuring out and classifying delicate information to allow collaboration with out compromising particular person information privateness. It’s important to embed these methods inside the AI/ML growth lifecycle workflows, keep a secure data and modeling environment, and keep in compliance with privateness rules and defend delicate data. By integrating security-focused measures into the AI/ML CoE’s methods, the group can higher mitigate dangers related to information breaches, unauthorized entry, and adversarial assaults, thereby offering integrity, confidentiality, and availability for its AI belongings and delicate data.
6. Operations
The AI/ML CoE must give attention to optimizing the effectivity and development potential of implementing generative AI inside the group’s framework. On this part, we focus on a number of key facets geared toward driving profitable integration whereas upholding workload efficiency.
Efficiency administration
Setting KPIs and metrics is pivotal to gauge effectiveness. Common evaluation of those metrics lets you observe progress, establish traits, and foster a tradition of continuous enchancment inside the CoE. Reporting on these insights supplies alignment with organizational targets and informs decision-making processes for enhanced AI/ML practices. Options equivalent to Bedrock integration with Amazon CloudWatch, helps observe and handle utilization metrics, and construct personalized dashboards for auditing.
An instance KPI is mannequin accuracy: assessing fashions towards benchmarks supplies dependable and reliable AI-generated outcomes.
Incident administration
AI/ML options want ongoing management and commentary to handle any anomalous actions. This requires establishing processes and techniques throughout the AI/ML platform, ideally automated. A standardized incident response technique must be developed and applied in alignment with the chosen monitoring resolution. This consists of parts equivalent to formalized roles and tasks, information sources and metrics to be monitored, techniques for monitoring, and response actions equivalent to mitigation, escalation, and root trigger evaluation.
Steady enchancment
Outline rigorous processes for generative AI mannequin growth, testing, and deployment. Streamline the event of generative AI fashions by defining and refining strong processes. Repeatedly consider the AI/ML platform efficiency and improve generative AI capabilities. This includes incorporating suggestions loops from stakeholders and end-users and dedicating sources to exploratory analysis and innovation in generative AI. These practices drive continuous enchancment and hold the CoE on the forefront of AI innovation. Moreover, implement generative AI initiatives seamlessly by adopting agile methodologies, sustaining complete documentation, conducting common benchmarking, and implementing {industry} greatest practices.
7. Enterprise
The AI/ML CoE helps drive enterprise transformation by repeatedly figuring out precedence ache factors and alternatives throughout enterprise models. Aligning enterprise challenges and alternatives to personalized AI/ML capabilities, the CoE drives speedy growth and deployment of high-value options. This alignment to actual enterprise wants permits step-change worth creation via new merchandise, income streams, productiveness, optimized operations, and buyer satisfaction.
Envision an AI technique
With the target to drive enterprise outcomes, set up a compelling multi-year imaginative and prescient and technique on how the adoption of AI/ML and generative AI methods can remodel main sides of the enterprise. This consists of quantifying the tangible worth at stake from AI/ML when it comes to revenues, price financial savings, buyer satisfaction, productiveness, and different very important efficiency indicators over an outlined strategic planning timeline, equivalent to 3–5 years. Moreover, the CoE should safe buy-in from executives throughout enterprise models by making the case for the way embracing AI/ML will create aggressive benefits and unlock step-change enhancements in key processes or choices.
Use case administration
To identify, qualify, and prioritize the most promising AI/ML use cases, the CoE facilitates an ongoing discovery dialogue with all enterprise models to floor their highest-priority challenges and alternatives. Every complicated enterprise situation or alternative have to be articulated by the CoE, in collaboration with enterprise unit leaders, as a well-defined downside and alternative assertion that lends itself to an AI/ML-powered resolution. These alternatives set up clear success metrics tied to enterprise KPIs and description the potential worth affect vs. implementation complexity. A prioritized pipeline of high-potential AI/ML use circumstances can then be created, rating alternatives primarily based on anticipated enterprise profit and feasibility.
Proof of idea
Earlier than enterprise full manufacturing growth, prototype proposed options for high-value use circumstances via managed proof of idea (PoC) initiatives centered on demonstrating preliminary viability. Fast suggestions loops throughout these PoC phases permit for iteration and refinement of approaches at a small scale previous to wider deployment. The CoE establishes clear success standards for PoCs, in alignment with enterprise unit leaders, that map to enterprise metrics and KPIs for final resolution affect. Moreover, the CoE can have interaction to share experience, reusable belongings, greatest practices, and requirements.
Govt alignment
To supply full transparency, the enterprise unit govt stakeholders have to be aligned with AI/ML initiatives, and have common reporting with them. This manner, any challenges that have to be escalated could be rapidly resolved with executives who’re conversant in the initiatives.
8. Authorized
The authorized panorama of AI/ML and generative AI is complicated and evolving, presenting a myriad of challenges and implications for organizations. Points equivalent to information privateness, mental property, legal responsibility, and bias require cautious consideration inside the AI/ML CoE. As rules wrestle to maintain tempo with technological developments, the CoE should accomplice with the group’s authorized workforce to navigate this dynamic terrain to implement compliance and accountable growth and deployment of those applied sciences. The evolving panorama calls for that the CoE, working in collaboration with the authorized workforce, develops complete AI/ML governance insurance policies overlaying the whole AI/ML lifecycle. This course of includes enterprise stakeholders in decision-making processes and common audits and critiques of AI/ML techniques to validate compliance with governance insurance policies.
9. Procurement
The AI/ML CoE must work with partners, each Impartial Software program Distributors (ISV) and System Integrators (SI) to assist with the purchase and construct methods. They should accomplice with the procurement workforce to develop a variety, onboarding, administration, and exit framework. This consists of buying applied sciences, algorithms, and datasets (sourcing dependable datasets is essential for coaching ML fashions, and buying cutting-edge algorithms and generative AI instruments enhances innovation). This can assist accelerated growth of capabilities wanted for enterprise. Procurement methods should prioritize moral issues, information safety, and ongoing vendor assist to offer sustainable, scalable, and accountable AI integration.
10. Human Sources
Accomplice with Human Sources (HR) on AI/ML expertise administration and pipeline. This includes cultivating expertise to know, develop, and implement these applied sciences. HR may help bridge the technical and non-technical divide, fostering interdisciplinary collaboration, constructing a path for onboarding new expertise, coaching them, and rising them on each skilled and expertise. They’ll additionally handle moral issues via compliance coaching, upskill staff on the most recent rising applied sciences, and handle the affect of job roles which are crucial for continued success.
11. Regulatory and compliance
The regulatory panorama for AI/ML is quickly evolving, with governments worldwide racing to determine governance regimes for the growing adoption of AI functions. The AI/ML CoE wants a centered strategy to remain up to date, derive actions, and implement regulatory legislations equivalent to Brazil’s Normal Private Knowledge Safety Legislation (LGPD), Canada’s Private Info Safety and Digital Paperwork Act (PIPEDA), and the European Union’s Normal Knowledge Safety Regulation (GDPR), and frameworks equivalent to ISO 31700, ISO 29100, ISO 27701, Federal Info Processing Requirements (FIPS), and NIST Privateness Framework. Within the US, regulatory actions embody mitigating dangers posed by the elevated adoption of AI, defending employees affected by generative AI, and offering stronger shopper protections. The EU AI Act consists of new evaluation and compliance necessities.
As AI rules proceed to take form, organizations are suggested to determine accountable AI as a C-level precedence, set and implement clear governance insurance policies and processes round AI/ML, and contain numerous stakeholders in decision-making processes. The evolving rules emphasize the necessity for complete AI governance insurance policies that cowl the whole AI/ML lifecycle, and common audits and critiques of AI techniques to handle biases, transparency, and explainability in algorithms. Adherence to requirements fosters belief, mitigates dangers, and promotes accountable deployment of those superior applied sciences.
Conclusion
The journey to establishing a profitable AI/ML middle of excellence is a multifaceted endeavor that requires dedication and strategic planning, whereas working with agility and collaborative spirit. Because the panorama of synthetic intelligence and machine studying continues to evolve at a speedy tempo, the creation of an AI/ML CoE represents a vital step in direction of harnessing these applied sciences for transformative affect. By specializing in the important thing issues, from defining a transparent mission to fostering innovation and imposing moral governance, organizations can lay a strong basis for AI/ML initiatives that drive worth. Furthermore, an AI/ML CoE is not only a hub for technological innovation; it’s a beacon for cultural change inside the group, selling a mindset of steady studying, moral accountability, and cross-functional collaboration.
Keep tuned as we proceed to discover the AI/ML CoE subjects in our upcoming posts on this collection. Should you need assistance establishing an AI/ML Heart of Excellence, please reach out to a specialist.
In regards to the Authors
Ankush Chauhan is a Sr. Supervisor, Buyer Options at AWS primarily based in New York, US. He helps Capital Markets clients optimize their cloud journey, scale adoption, and understand the transformative worth of constructing and inventing within the cloud. As well as, he’s centered on enabling clients on their AI/ML journeys together with generative AI. Past work, you will discover Ankush operating, mountaineering, or watching soccer.
Ava Kong is a Generative AI Strategist on the AWS Generative AI Innovation Heart, specializing within the monetary companies sector. Primarily based in New York, Ava has labored carefully with a wide range of monetary establishments on a wide range of use circumstances, combining the most recent in generative AI expertise with strategic insights to boost operational effectivity, drive enterprise outcomes, and display the broad and impactful software of AI applied sciences.
Vikram Elango is a Sr. AI/ML Specialist Options Architect at AWS, primarily based in Virginia, US. He’s at present centered on generative AI, LLMs, immediate engineering, giant mannequin inference optimization, and scaling ML throughout enterprises. Vikram helps monetary and insurance coverage {industry} clients with design and thought management to construct and deploy machine studying functions at scale. In his spare time, he enjoys touring, mountaineering, cooking, and tenting together with his household.
Rifat Jafreen is a Generative AI Strategist within the AWS Generative AI Innovation middle the place her focus is to assist clients understand enterprise worth and operational effectivity by utilizing generative AI. She has labored in industries throughout telecom, finance, healthcare and vitality; and onboarded machine studying workloads for quite a few clients. Rifat can be very concerned in MLOps, FMOps and Accountable AI.
Authors wish to lengthen particular due to Arslan Hussain, David Ping, Jarred Graber, and Raghvender Arni, for his or her assist, experience, and steerage.