Researchers at Apple Suggest ReDrafter: Altering Giant Language Mannequin Effectivity with Speculative Decoding and Recurrent Neural Networks
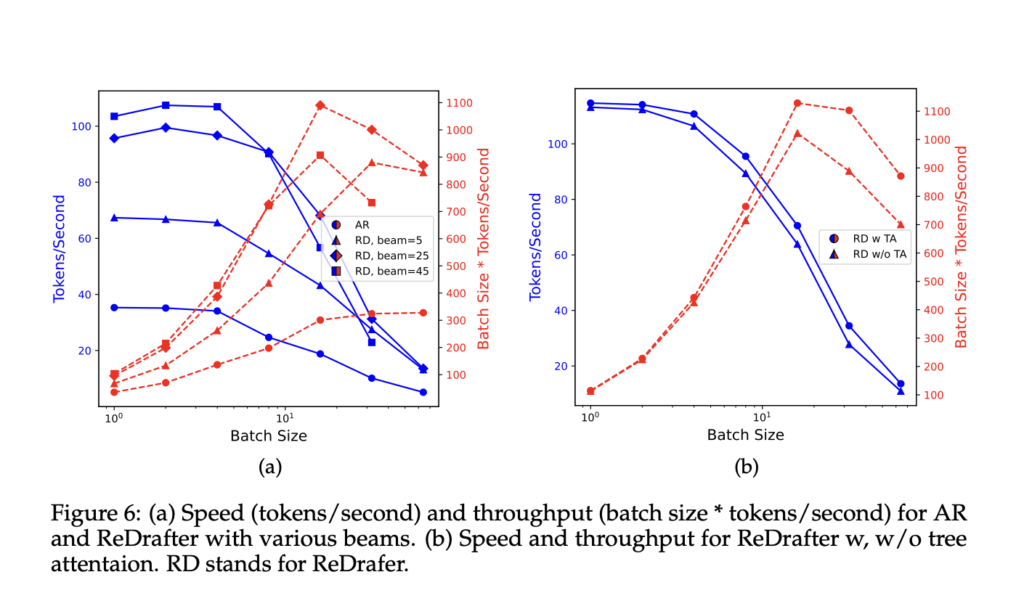
The event and refinement of enormous language fashions (LLMs) mark a big step within the progress of machine studying. These refined algorithms, designed to imitate human language, are on the coronary heart of recent technological conveniences, powering all the pieces from digital assistants to content material creation instruments. Nonetheless, the journey in the direction of creating responsive, correct, and conversational AI has been marred by a big hurdle: the processing velocity of producing textual responses.
Central to addressing this problem are initiatives to scale back the time these LLMs take to provide textual content. The central problem revolves across the fashions’ sequential nature, the place the era of every phrase is dependent upon the completion of its predecessors. This dependency not solely slows down the response time but additionally limits the fashions’ utility in real-time eventualities, a spot that has led to the exploration of speculative decoding strategies. These methods leverage smaller, nimbler fashions to foretell batches of potential subsequent tokens, refined by the bigger goal mannequin. The stability between velocity and accuracy is delicate, demanding an answer that may navigate the complexities of language with out compromising on the standard of output.
A group of researchers from Apple launched ReDrafter, a technique that ingeniously combines the strengths of speculative decoding with the adaptive capabilities of recurrent neural networks (RNNs). ReDrafter distinguishes itself by using a single, versatile draft head with a recurrent dependency design. This design simplifies the inference course of by streamlining the preliminary prediction part, thus decreasing the computational load with out diminishing the mannequin’s depth or the richness of its output. The wonderful thing about ReDrafter lies in its skill to keep up a nuanced understanding of LLMs whereas considerably enhancing their operational effectivity.
ReDrafter’s success lies in its distinctive skill to swiftly sift by and remove suboptimal candidate tokens utilizing beam search, a feat made attainable by its recurrently dependent draft head. This strategy obviates the necessity to assemble complicated, data-dependent tree consideration constructions solely for inference, which is critical for strategies like Medusa. The recurrent nature of ReDrafter’s design permits for a streamlined, environment friendly predictive course of that considerably accelerates response era with out compromising the mannequin’s depth or output high quality.
The group’s empirical evaluation demonstrated ReDrafter’s superiority over current strategies, marking a big development in speculative decoding know-how. By optimizing the velocity and accuracy of textual content era, ReDrafter improves the consumer expertise in real-time functions and opens up new avenues for deploying LLMs throughout varied sectors. Whether or not for fast translation providers, interactive instructional instruments, or buyer assist chatbots, the potential of this innovation is huge, promising a future the place interactions with AI are as easy as these with a human.
ReDrafter’s innovation successfully merges the predictive energy of speculative decoding with the effectivity of RNNs. The researchers have crafted an answer addressing the long-standing textual content era latency problem. This breakthrough underscores the potential of reimagining typical approaches to mannequin design, hinting that the important thing to unlocking the following degree of AI efficiency lies in integrating disparate strategies right into a unified, optimized framework.
In conclusion, the arrival of ReDrafter by the Apple analysis group represents a paradigm shift within the pursuit of environment friendly LLM processing. By ingeniously merging speculative decoding with recurrent neural community methods, this technique transcends conventional obstacles, providing a streamlined, efficient resolution for speedy textual content era. The implications of this improvement improve the responsiveness and applicability of LLMs’ real-time interactions.
Take a look at the Paper. All credit score for this analysis goes to the researchers of this challenge. Additionally, don’t overlook to comply with us on Twitter. Be a part of our Telegram Channel, Discord Channel, and LinkedIn Group.
For those who like our work, you’ll love our newsletter..
Don’t Neglect to affix our 38k+ ML SubReddit
Hiya, My title is Adnan Hassan. I’m a consulting intern at Marktechpost and shortly to be a administration trainee at American Specific. I’m at present pursuing a twin diploma on the Indian Institute of Know-how, Kharagpur. I’m enthusiastic about know-how and wish to create new merchandise that make a distinction.