Amazon AI Researchers Introduce Chronos: A New Machine Studying Framework for Pretrained Probabilistic Time Sequence Fashions
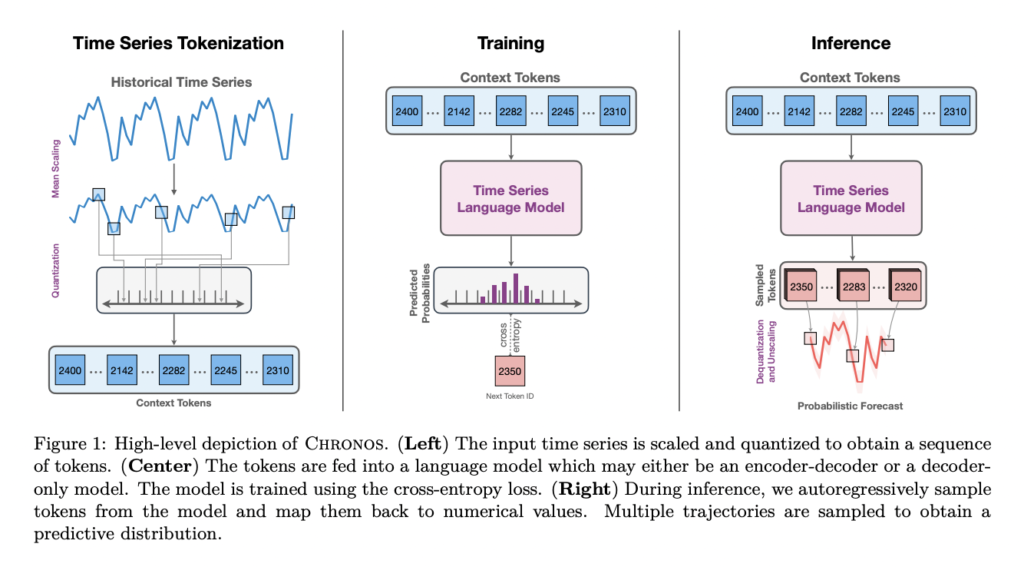
Correct forecasting instruments are essential in industries reminiscent of retail, finance, and healthcare, and they’re always advancing towards higher sophistication and accessibility. Historically anchored by statistical fashions like ARIMA, the area has witnessed a paradigm shift with the appearance of deep studying. These fashionable strategies have unlocked the flexibility to decipher advanced patterns from voluminous and various datasets, albeit at the price of elevated computational demand and experience.
A staff from Amazon Net Companies, in collaboration with UC San Diego, the College of Freiburg, and Amazon Provide Chain Optimization Applied sciences, introduces a revolutionary framework referred to as Chronos. This progressive instrument redefines time sequence forecasting by merging numerical information evaluation with language processing, harnessing the ability of transformer-based language fashions. By simplifying the forecasting pipeline, Chronos opens the door to superior analytics for a wider viewers.
Chronos operates on a singular precept: it tokenizes numerical time sequence information, remodeling it right into a format that pre-trained language fashions can perceive. This course of entails scaling and quantizing the information into discrete bins, just like how phrases kind a vocabulary in language fashions. This tokenization permits Chronos to make use of the identical architectures as pure language processing duties, such because the T5 household of fashions, to forecast future information factors in a time sequence. This method not solely democratizes entry to superior forecasting strategies but in addition improves the effectivity of the forecasting course of.
Chronos’s ingenuity extends to its methodology, which capitalizes on the sequential nature of time sequence information akin to language construction. By treating time sequence forecasting as a language modeling downside, Chronos minimizes the necessity for domain-specific changes. The framework’s skill to know and predict future patterns with out intensive customization represents a big leap ahead. It embodies a minimalist but efficient technique, specializing in forecasting with minimal alterations to the underlying mannequin structure.
The efficiency of Chronos is really spectacular. In a complete benchmark throughout 42 datasets, together with each classical and deep studying fashions, Chronos demonstrated superior efficiency. It outperformed different strategies within the datasets a part of its coaching corpus, exhibiting its skill to generalize from coaching information to real-world forecasting duties. In zero-shot forecasting eventualities, the place fashions predict outcomes for datasets they haven’t been immediately educated on, Chronos confirmed comparable, and typically superior, efficiency in opposition to fashions particularly educated for these datasets. This functionality underscores the framework’s potential to function a common instrument for forecasting throughout numerous domains.
The creation of Chronos by researchers at Amazon Net Companies and their educational companions marks a key second in time sequence forecasting. By bridging the hole between numerical information evaluation and pure language processing, they haven’t solely streamlined the forecasting course of but in addition expanded the potential purposes of language fashions.
Take a look at the Paper. All credit score for this analysis goes to the researchers of this mission. Additionally, don’t neglect to comply with us on Twitter. Be part of our Telegram Channel, Discord Channel, and LinkedIn Group.
If you happen to like our work, you’ll love our newsletter..
Don’t Overlook to hitch our 38k+ ML SubReddit
Muhammad Athar Ganaie, a consulting intern at MarktechPost, is a proponet of Environment friendly Deep Studying, with a give attention to Sparse Coaching. Pursuing an M.Sc. in Electrical Engineering, specializing in Software program Engineering, he blends superior technical data with sensible purposes. His present endeavor is his thesis on “Bettering Effectivity in Deep Reinforcement Studying,” showcasing his dedication to enhancing AI’s capabilities. Athar’s work stands on the intersection “Sparse Coaching in DNN’s” and “Deep Reinforcemnt Studying”.