Unveiling the Simplicity inside Complexity: The Linear Illustration of Ideas in Massive Language Fashions
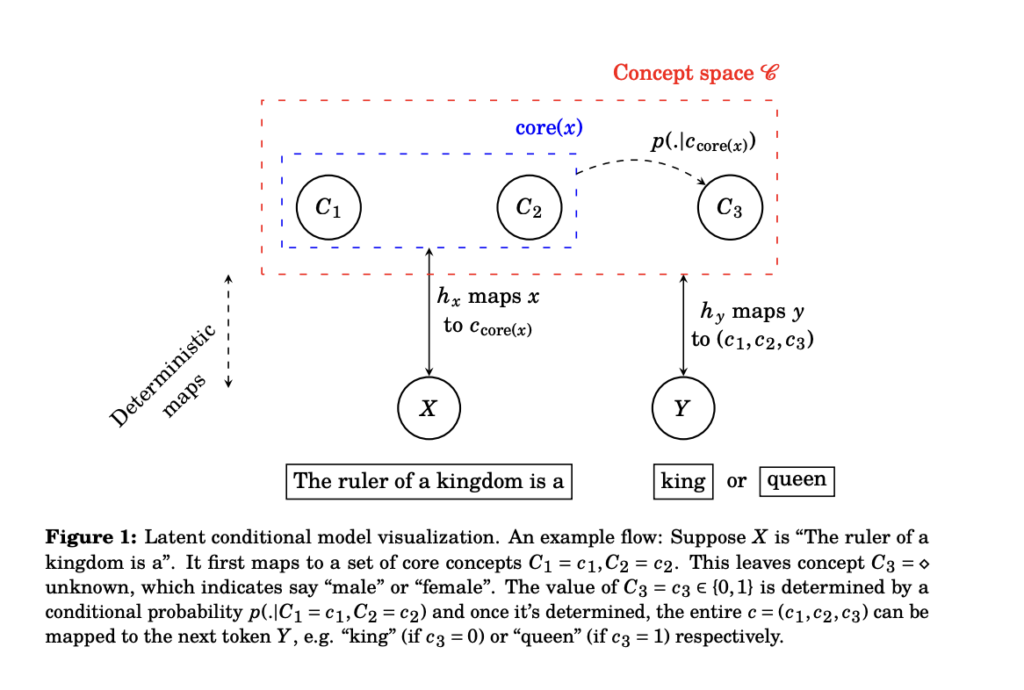
Within the evolving panorama of synthetic intelligence, the examine of how machines perceive and course of human language has unveiled intriguing insights, significantly inside giant language fashions (LLMs). These digital marvels, designed to foretell subsequent phrases or generate textual content, embody a realm of complexity that belies the underlying simplicity of their strategy to language.
A captivating facet of LLMs that has piqued the educational neighborhood’s curiosity is their methodology of idea illustration. Historically, one may anticipate these fashions to make use of intricate mechanisms to encode the nuances of language. Nonetheless, observations reveal a surprisingly simple strategy: ideas are sometimes encoded linearly. The revelation poses an intriguing query: How do complicated fashions symbolize semantic ideas so merely?
Researchers from the College of Chicago and Carnegie Mellon College have proposed a novel perspective to demystify the foundations of linear representations in LLMs to handle the above-posed problem. Their investigation pivots round a conceptual framework, a latent variable mannequin that simplifies understanding of how LLMs predict the subsequent token in a sequence. By way of its elegant abstraction, this mannequin permits for a deeper dive into the mechanics of language processing in these fashions.
The middle of their investigation lies in a speculation that challenges typical understanding. The researchers suggest that the linear illustration of ideas in LLMs will not be an incidental byproduct of their design however relatively a direct consequence of the fashions’ coaching targets and the inherent biases of the algorithms powering them. Particularly, they counsel that the softmax operate mixed with cross-entropy loss, when used as a coaching goal, alongside the implicit bias launched by gradient descent, encourages the emergence of linear idea illustration.
The speculation was examined by way of a sequence of experiments, each in artificial eventualities and real-world information, utilizing the LLaMA-2 mannequin. The outcomes weren’t simply confirming; they had been groundbreaking. Linear representations had been noticed underneath situations predicted by their mannequin, aligning idea and apply. This substantiates the linear illustration speculation and sheds new gentle on the educational and internalizing technique of language in LLMs.
The importance of those findings is that unraveling the components that foster linear illustration opens up a world of potentialities for LLM growth. The intricacies of human language, with its huge array of semantics, will be encoded remarkably straightforwardly. This might probably result in the creating of extra environment friendly and interpretable fashions, revolutionizing how we strategy pure language processing and making it extra accessible and comprehensible.
This examine is a vital hyperlink between the summary theoretical foundations of LLMs and their sensible purposes. By illuminating the mechanisms behind idea illustration, the analysis gives a elementary perspective that may steer future developments within the discipline. It challenges researchers and practitioners to rethink the design and coaching of LLMs, highlighting the importance of simplicity and effectivity in engaging in complicated duties.
In conclusion, exploring the origins of linear representations in LLMs marks a big milestone in our understanding of synthetic intelligence. The collaborative analysis effort sheds gentle on the simplicity underlying the complicated processes of LLMs, providing a recent perspective on the mechanics of language comprehension in machines. This journey into the center of LLMs not solely broadens our understanding but additionally highlights the countless potentialities within the interaction between simplicity and complexity in synthetic intelligence.
Try the Paper. All credit score for this analysis goes to the researchers of this challenge. Additionally, don’t overlook to observe us on Twitter and Google News. Be part of our 38k+ ML SubReddit, 41k+ Facebook Community, Discord Channel, and LinkedIn Group.
If you happen to like our work, you’ll love our newsletter..
Don’t Neglect to affix our Telegram Channel
You may additionally like our FREE AI Courses….