UC Berkeley Analysis Presents a Machine Studying System that Can Forecast at Close to Human Ranges
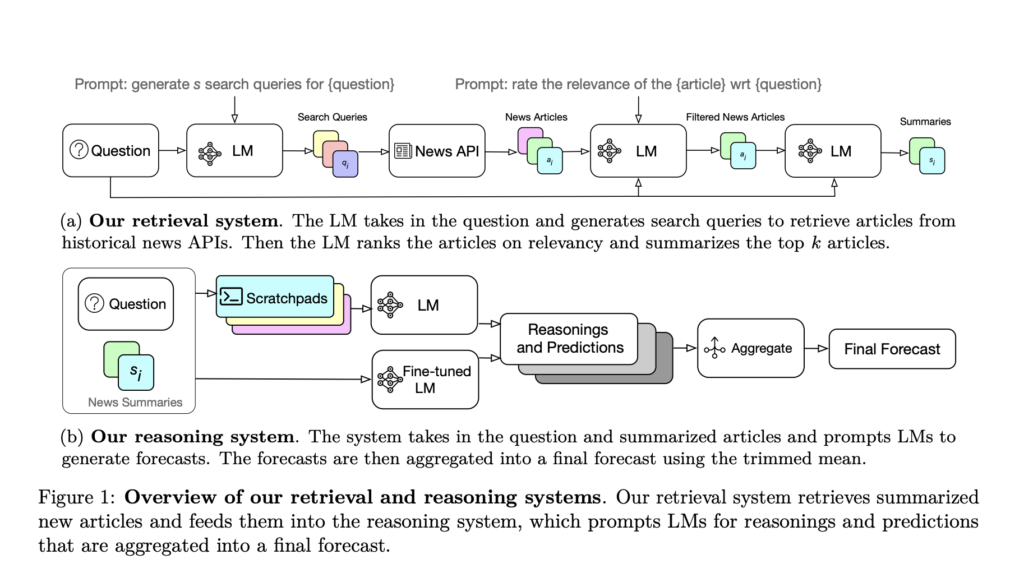
Within the evolving panorama of predictive analytics, the artwork and science of forecasting stand as pivotal instruments for decision-making throughout varied sectors, from authorities coverage to company technique. Forecasting has relied closely on statistical strategies, thriving on ample information and minimal shifts in underlying patterns. Nonetheless, judgmental forecasting has launched a nuanced strategy, leveraging human instinct, area data, and various data sources to foretell future occasions beneath information shortage and uncertainty.
The problem in predictive forecasting lies in its inherent complexity and the constraints of current methodologies. Statistical fashions, whereas highly effective, typically must catch up in situations marked by information shortage or important adjustments in information distribution. Judgmental forecasting, nevertheless, introduces the human component, with all its insights and biases, into the equation. This technique depends on forecasters’ means to synthesize data from different sources, together with historic information and present occasions, to make knowledgeable predictions about future outcomes.
A analysis crew from UC Berkeley has developed a novel LM pipeline, a retrieval-augmented language mannequin system particularly designed for forecasting. This technique automates essential parts of the forecasting course of, together with the retrieval of related data from information sources, the reasoning primarily based on the information gathered, and the aggregation of particular person forecasts right into a complete prediction. The core of this innovation lies in its means to harness web-scale information and the fast parsing capabilities of LMs, providing a scalable and environment friendly various to conventional forecasting strategies.
The system combines completely different approaches to realize complete protection in forecasting by decomposing questions into sub-questions and utilizing search queries. Articles are retrieved from information APIs and filtered primarily based on relevance scores supplied by GPT-3.5-Turbo. The articles are then summarized to suit throughout the context window of the language mannequin. Reasoning is a crucial side of correct forecasting, and the system makes use of scratchpad prompts to information the mannequin’s reasoning course of. It ensembles predictions from completely different fashions to enhance accuracy, and the retrieval and reasoning system is optimized via a hyperparameter sweep, together with optimizing prompts, article summaries, and ensembling strategies. This intricate course of permits for a extra knowledgeable and nuanced strategy to prediction, leveraging language fashions’ huge data and fast processing capabilities.
The researchers are very constructive with the outcomes obtained from the research. On a complete take a look at set, the system achieved a mean Brier rating of .179, intently approaching the human mixture rating of .149, indicating that the language model-based forecasting system intently approximates, and in some situations surpasses, the accuracy of human forecasters aggregated from aggressive platforms. This discovering suggests a major potential for language fashions to contribute to predictive forecasting, providing correct predictions at scale and facilitating extra knowledgeable decision-making processes.
In conclusion, the research presents a compelling case for integrating language fashions within the forecasting area and highlights the potential for these instruments to reinforce predictive accuracy and effectivity. Whereas the journey from analysis to real-world software entails quite a few challenges and concerns, the foundational work laid by the UC Berkeley crew marks a major step ahead within the ongoing efforts for extra dependable and accessible forecasting strategies. The implications of this analysis prolong past tutorial curiosity, promising to affect decision-making processes in authorities, enterprise, and past as we navigate future uncertainties.
Take a look at the Paper. All credit score for this analysis goes to the researchers of this mission. Additionally, don’t neglect to observe us on Twitter and Google News. Be part of our 38k+ ML SubReddit, 41k+ Facebook Community, Discord Channel, and LinkedIn Group.
In the event you like our work, you’ll love our newsletter..
Don’t Overlook to hitch our Telegram Channel
You might also like our FREE AI Courses….
Nikhil is an intern guide at Marktechpost. He’s pursuing an built-in twin diploma in Supplies on the Indian Institute of Know-how, Kharagpur. Nikhil is an AI/ML fanatic who’s at all times researching functions in fields like biomaterials and biomedical science. With a powerful background in Materials Science, he’s exploring new developments and creating alternatives to contribute.