CMU Researchers Introduce OWSM v3.1: A Higher and Quicker Open Whisper-Type Speech Mannequin-Primarily based on E-Branchformer
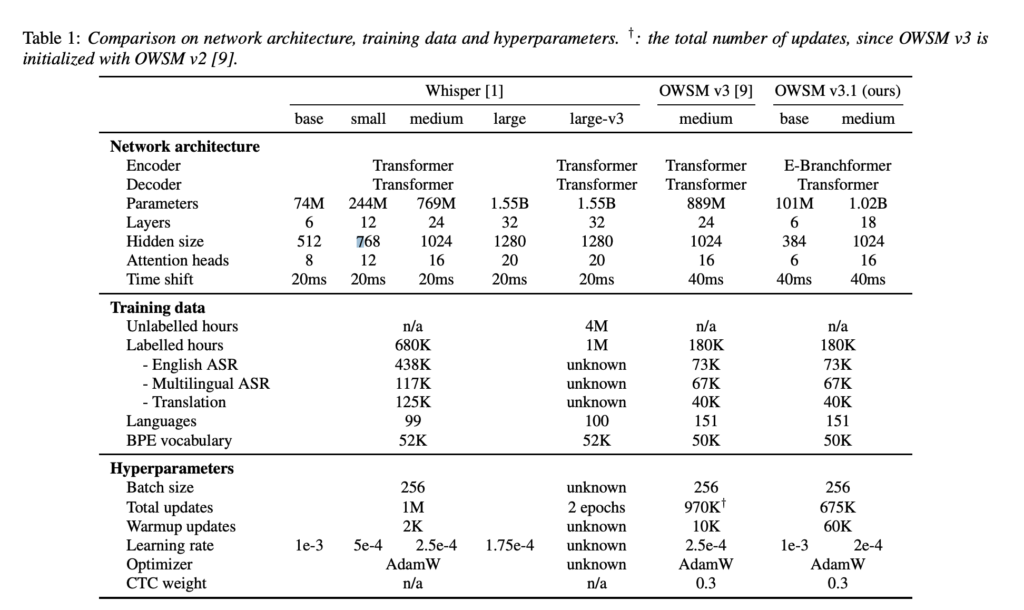
Speech recognition expertise has turn out to be a cornerstone for varied purposes, enabling machines to grasp and course of human speech. The sphere repeatedly seeks developments in algorithms and fashions to enhance accuracy and effectivity in recognizing speech throughout a number of languages and contexts. The principle problem in speech recognition is growing fashions that precisely transcribe speech from varied languages and dialects. Fashions usually need assistance with the variability of speech, together with accents, intonation, and background noise, resulting in a requirement for extra sturdy and versatile options.
Researchers have been exploring varied strategies to boost speech recognition techniques. Current options have usually relied on advanced architectures like Transformers, which, regardless of their effectiveness, face limitations, significantly in processing velocity and the nuanced job of precisely recognizing and deciphering a big selection of speech nuances, together with dialects, accents, and variations in speech patterns.
The Carnegie Mellon College and Honda Analysis Institute Japan analysis crew launched a brand new mannequin, OWSM v3.1, using the E-Branchformer structure to deal with these challenges. OWSM v3.1 is an improved and quicker Open Whisper-style Speech Mannequin that achieves higher outcomes than the earlier OWSM v3 in most analysis circumstances.
The earlier OWSM v3 and Whisper each make the most of the usual Transformer encoder-decoder structure. Nonetheless, latest developments in speech encoders equivalent to Conformer and Branchformer have improved efficiency in speech processing duties. Therefore, the E-Branchformer is employed because the encoder in OWSM v3.1, demonstrating its effectiveness at a scale of 1B parameters. OWSM v3.1 excludes the WSJ coaching knowledge utilized in OWSM v3, which had absolutely uppercased transcripts. This exclusion results in a considerably decrease Phrase Error Charge (WER) in OWSM v3.1. It additionally demonstrates as much as 25% quicker inference velocity.
OWSM v3.1 demonstrated vital achievements in efficiency metrics. It outperformed its predecessor, OWSM v3, in most analysis benchmarks, reaching greater accuracy in speech recognition duties throughout a number of languages. In comparison with OWSM v3, OWSM v3.1 reveals enhancements in English-to-X translation in 9 out of 15 instructions. Though there could also be a slight degradation in some instructions, the typical BLEU rating is barely improved from 13.0 to 13.3.
In conclusion, the analysis considerably strides in the direction of enhancing speech recognition expertise. By leveraging the E-Branchformer structure, the OWSM v3.1 mannequin improves upon earlier fashions when it comes to accuracy and effectivity and units a brand new customary for open-source speech recognition options. By releasing the mannequin and coaching particulars publicly, the researchers’ dedication to transparency and open science additional enriches the sphere and paves the best way for future developments.
Take a look at the Paper and Demo. All credit score for this analysis goes to the researchers of this undertaking. Additionally, don’t neglect to observe us on Twitter and Google News. Be a part of our 36k+ ML SubReddit, 41k+ Facebook Community, Discord Channel, and LinkedIn Group.
In case you like our work, you’ll love our newsletter..
Don’t Overlook to hitch our Telegram Channel
Nikhil is an intern advisor at Marktechpost. He’s pursuing an built-in twin diploma in Supplies on the Indian Institute of Expertise, Kharagpur. Nikhil is an AI/ML fanatic who’s all the time researching purposes in fields like biomaterials and biomedical science. With a powerful background in Materials Science, he’s exploring new developments and creating alternatives to contribute.