Google DeepMind Researchers Introduce GenCast: Diffusion-based Ensemble Forecasting AI Mannequin for Medium-Vary Climate
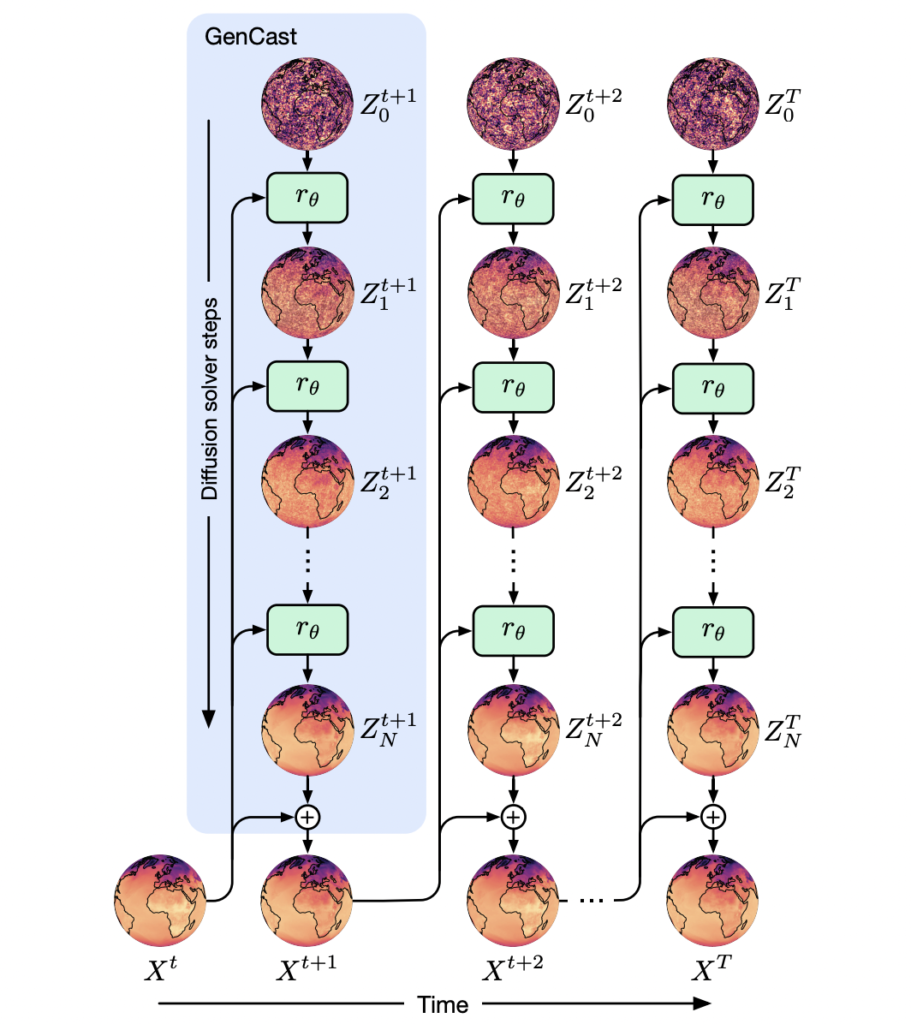
You’ll have missed an enormous growth within the ML climate forecasting revolution over the vacations: GenCast: Google DeepMind’s new generative mannequin! The significance of probabilistic climate forecasting can’t be overstated in varied important domains like flood forecasting, power system planning, and transportation routing. With the ability to precisely gauge the uncertainty in forecasts, particularly regarding excessive occasions, is pivotal for making well-informed selections that contain vital cost-benefit issues and efficient mitigation methods.
Historically, the method to probabilistic forecasting includes creating ensembles from physics-based fashions, which pattern from a joint distribution over spatio-temporally coherent climate trajectories. Nevertheless, this technique might be computationally costly. An interesting various is using machine studying (ML) forecast fashions to generate ensembles. But, the present cutting-edge ML forecast fashions for medium-range climate primarily concentrate on producing deterministic forecasts that decrease mean-squared error.
Regardless of the improved talent scores related to these fashions, they face a limitation when it comes to missing bodily consistency. This limitation turns into extra pronounced at longer lead instances, impacting their skill to characterize the joint distribution of climate occasions exactly.
The paper introduces a novel machine learning-based method for probabilistic climate forecasting referred to as GenCast. This revolutionary technique generates world, 15-day ensemble forecasts that display superior accuracy in comparison with the main operational ensemble forecast, particularly the European Centre for Medium-range Climate Forecasts (ECMWF)’s ENS, all whereas requiring considerably much less computation time. GenCast operates by implicitly modeling the joint chance distribution of the climate state over house and time. It really works on a 1° latitude-longitude grid, using 12-hour time steps, and represents six floor variables and 6 atmospheric variables at 13 vertical strain ranges.
The analysis of GenCast’s forecasts exhibits that it retains detailed patterns and consistency in climate predictions. Comparisons with ENS point out that GenCast’s ensembles are simply as dependable, if no more so. GenCast is environment friendly—it will possibly create a 15-day forecast in a few minute utilizing a Cloud TPU v4. This implies producing a lot of forecasts ( ensemble members) in a short while is feasible with a number of TPUs. This effectivity opens up the potential of utilizing a lot bigger ensembles sooner or later.
In a broader context, GenCast signifies a big development in machine learning-based climate forecasting, demonstrating increased proficiency than the main operational ensemble forecast at a 1° decision. This growth marks a pivotal step towards ushering in a brand new period of ensemble forecasting pushed by machine studying, increasing its relevance and usefulness throughout a various array of domains. Furthermore, as we glance forward, GenCast provides a glimpse into the potential of embracing machine studying to revolutionize our understanding and prediction of advanced climate patterns, with far-reaching implications for varied industries and decision-makers.
Take a look at the Paper. All credit score for this analysis goes to the researchers of this mission. Additionally, don’t overlook to comply with us on Twitter. Be part of our 36k+ ML SubReddit, 41k+ Facebook Community, Discord Channel, and LinkedIn Group.
In the event you like our work, you’ll love our newsletter..
Don’t Overlook to hitch our Telegram Channel
Janhavi Lande, is an Engineering Physics graduate from IIT Guwahati, class of 2023. She is an upcoming information scientist and has been working on the planet of ml/ai analysis for the previous two years. She is most fascinated by this ever altering world and its fixed demand of people to maintain up with it. In her pastime she enjoys touring, studying and writing poems.