AWS Analysis on Specializing Massive Language Fashions: Leveraging Self-Discuss and Automated Analysis Metrics for Enhanced Coaching
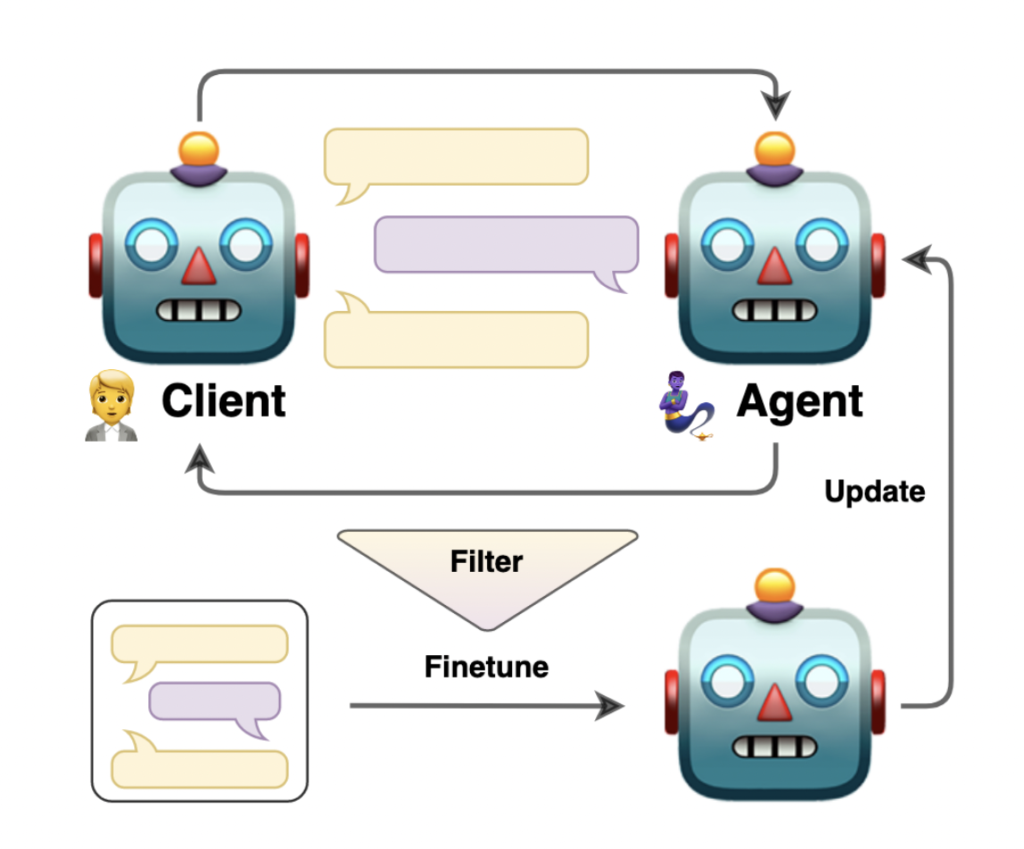
In user-centric functions like private help and buyer help, language fashions are more and more being deployed as dialogue brokers within the quickly advancing area of synthetic intelligence. These brokers are tasked with understanding and responding to numerous consumer queries and duties, a functionality that hinges on their capability to adapt to new situations shortly. Nonetheless, customizing these common language fashions for particular features presents important challenges, primarily because of the want for in depth, specialised coaching information.
Historically, the fine-tuning of those fashions, often called instructing tuning, has relied on human-generated datasets. Whereas efficient, this method faces hurdles just like the restricted availability of related information and the complexities of molding brokers to stick to intricate dialogue workflows. These constraints have been a stumbling block in creating extra responsive and task-oriented dialogue brokers.
Addressing these challenges, a crew of researchers from the IT College of Copenhagen, Pioneer Centre for Synthetic Intelligence, and AWS AI Labs have launched an progressive resolution: the self-talk methodology. This method includes leveraging two variations of a language mannequin that have interaction in a self-generated dialog, every taking up totally different roles throughout the dialogue. Such a technique not solely aids in producing a wealthy and diverse coaching dataset but in addition streamlines fine-tuning the brokers to observe particular dialogue constructions extra successfully.
The core of the self-talk methodology lies in its structured prompting approach. Right here, dialogue flows are transformed into directed graphs, guiding the dialog between the AI fashions. This structured interplay ends in numerous situations, successfully simulating real-world discussions. The dialogues generated from this course of are then meticulously evaluated and refined, yielding a high-quality dataset. This dataset is instrumental in coaching the brokers, permitting them to grasp particular duties and workflows extra exactly.
The efficacy of the self-talk method is obvious in its efficiency outcomes. The approach has proven important promise in enhancing the capabilities of dialogue brokers, notably of their relevance to particular duties. By specializing in the standard of the conversations generated and using rigorous analysis strategies, the researchers have remoted and utilized the best dialogues for coaching functions. This has resulted within the improvement of extra refined and task-oriented dialogue brokers.
Furthermore, the self-talk methodology stands out for its cost-effectiveness and innovation in coaching information era. This method circumvents the reliance on in depth human-generated datasets, providing a extra environment friendly and scalable resolution. The self-talk methodology thus represents a big leap ahead within the discipline of dialogue brokers, opening new avenues for creating AI programs that may deal with specialised duties and workflows with elevated effectiveness and relevance.
In conclusion, the self-talk methodology marks a notable development in AI and dialogue brokers. It showcases an ingenious and resourceful method to overcoming the challenges of specialised coaching information era. This methodology enhances the efficiency of dialogue brokers and broadens the scope of their functions, making them more proficient at dealing with task-specific interactions. As AI programs turn out to be extra refined and responsive, improvements are essential in pushing their capabilities to new heights.
Try the Paper. All credit score for this analysis goes to the researchers of this challenge. Additionally, don’t overlook to observe us on Twitter. Be a part of our 36k+ ML SubReddit, 41k+ Facebook Community, Discord Channel, and LinkedIn Group.
When you like our work, you’ll love our newsletter..
Don’t Neglect to hitch our Telegram Channel
Muhammad Athar Ganaie, a consulting intern at MarktechPost, is a proponet of Environment friendly Deep Studying, with a give attention to Sparse Coaching. Pursuing an M.Sc. in Electrical Engineering, specializing in Software program Engineering, he blends superior technical data with sensible functions. His present endeavor is his thesis on “Enhancing Effectivity in Deep Reinforcement Studying,” showcasing his dedication to enhancing AI’s capabilities. Athar’s work stands on the intersection “Sparse Coaching in DNN’s” and “Deep Reinforcemnt Studying”.