Enhanced Massive Language Fashions as Reasoning Engines | by Anthony Alcaraz | Dec, 2023
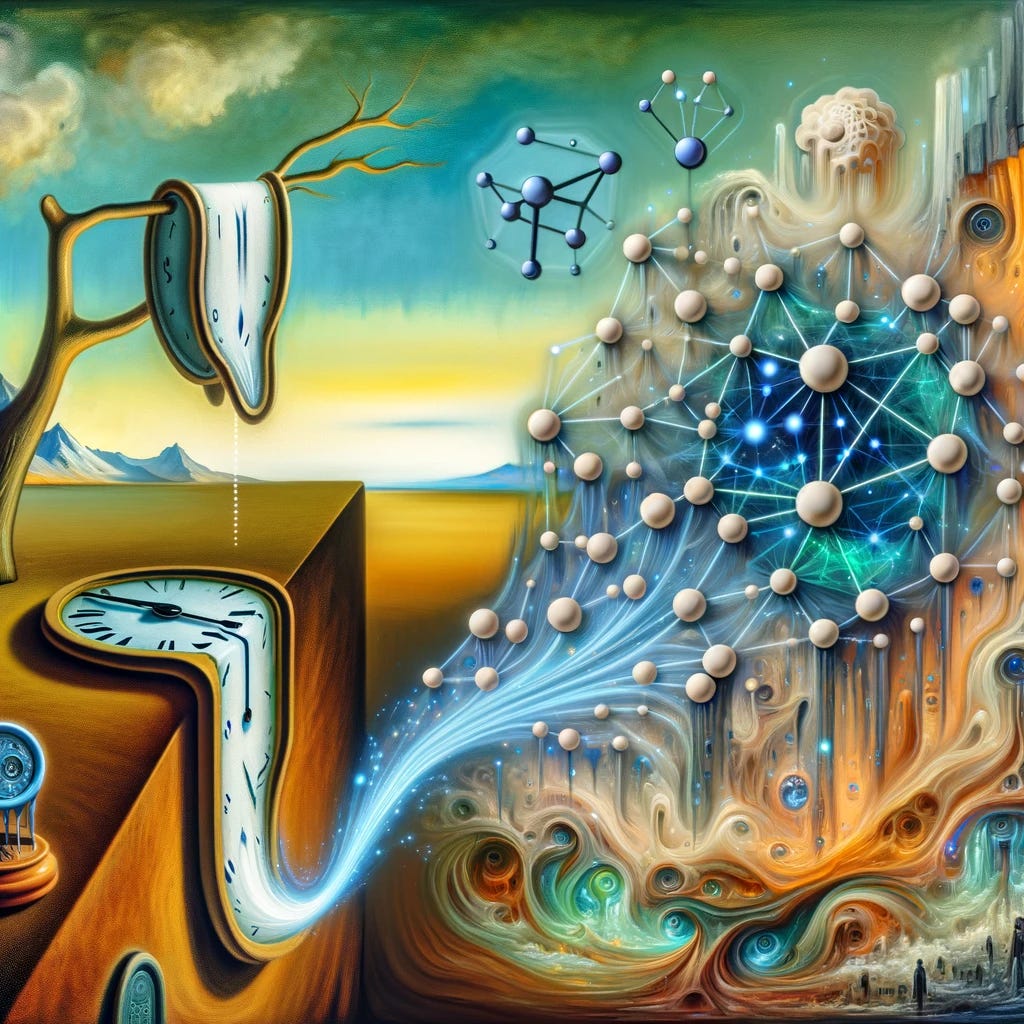
The latest exponential advances in pure language processing capabilities from massive language fashions (LLMs) have stirred great pleasure about their potential to realize human-level intelligence. Their skill to supply remarkably coherent textual content and interact in dialogue after publicity to huge datasets appears to level in direction of versatile, common goal reasoning expertise.
Nevertheless, a rising refrain of voices urges warning in opposition to unchecked optimism by highlighting basic blindspots that restrict neural approaches. LLMs nonetheless often make primary logical and mathematical errors that reveal a scarcity of systematicity behind their responses. Their information stays intrinsically statistical with out deeper semantic constructions.
Extra complicated reasoning duties additional expose these limitations. LLMs wrestle with causal, counterfactual, and compositional reasoning challenges that require going past floor sample recognition. In contrast to people who be taught summary schemas to flexibly recombine modular ideas, neural networks memorize correlations between co-occurring phrases. This ends in brittle generalization outdoors slim coaching distributions.
The chasm underscores how human cognition employs structured symbolic representations to allow systematic composability and causal fashions for conceptualizing dynamics. We purpose by manipulating modular symbolic ideas primarily based on legitimate inference guidelines, chaining logical dependencies, leveraging psychological simulations, and postulating mechanisms relating variables. The inherently statistical nature of neural networks precludes growing such structured reasoning.
It stays mysterious how symbolic-like phenomena emerge in LLMs regardless of their subsymbolic substrate. However clearer acknowledgement of this “hybridity hole” is crucial. True progress requires embracing complementary strengths — the pliability of neural approaches with structured information representations and causal reasoning strategies — to create built-in reasoning methods.
We first define the rising refrain of analyses exposing neural networks’ lack of systematicity, causal comprehension, and compositional generalization — underscoring variations from innate human colleges.
Subsequent, we element salient aspects of the “reasoning hole”, together with struggles with modular talent orchestration, unraveling dynamics, and counterfactual simulation. We floor innate human capacities…