This AI Analysis from Cohere AI Introduces the Combination of Vectors (MoV) and Combination of LoRA (MoLORA) to Mitigate the Challenges Related to Scaling Instruction-Tuned LLMs at Scale
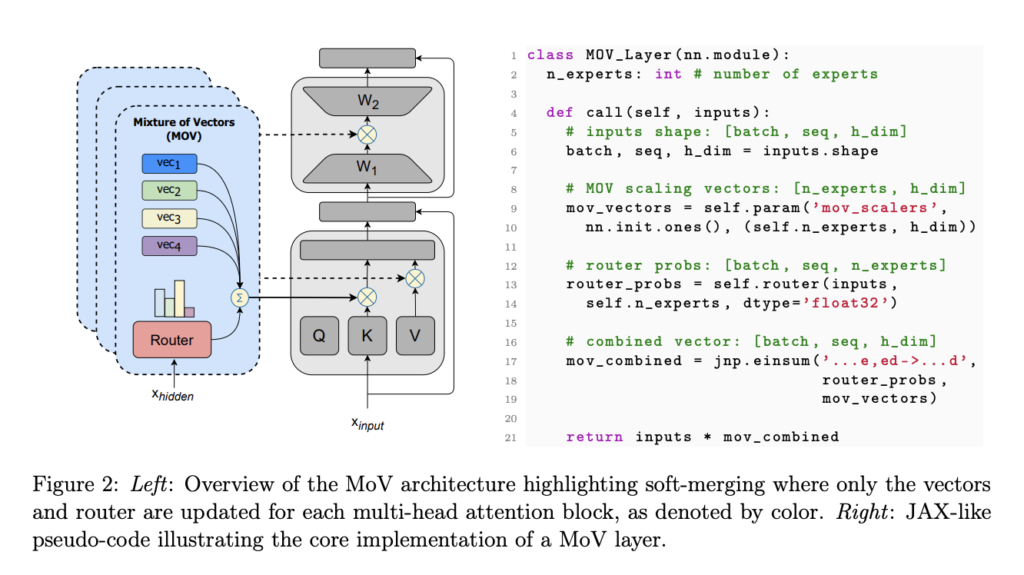
With the rising developments within the subject of Synthetic Intelligence (AI), researchers are always arising with new transformations and improvements. One such pioneering improvement is within the area of Combination of Specialists (MoE) structure, a widely known neural framework recognized for its capability to maximise total efficiency at a continuing computing value.
Nonetheless, when AI fashions get larger, conventional MoEs have bother conserving monitor of each reminiscence skilled. To beat this, in latest analysis, a workforce of Cohere researchers has studied about methods to broaden the capabilities of MoE by presenting a really parameter-efficient model that solves these scalability issues. Light-weight specialists have been mixed with the MoE structure as a way to obtain this.
The advised MoE structure is a extremely efficient method for parameter-efficient fine-tuning (PEFT) because it surpasses the drawbacks of typical fashions. The workforce has shared that incorporating light-weight specialists is the first innovation enabling the mannequin to surpass typical PEFT strategies. Even when updating solely the light-weight specialists, which is lower than 1% of a mannequin with 11 billion parameters, the efficiency demonstrated was akin to full fine-tuning.
The mannequin’s capability to generalize to duties that haven’t been seen earlier than, highlighting its independence from prior activity information, is one wonderful characteristic of the analysis. This implies that the proposed MoE structure isn’t restricted to specific domains and may efficiently modify to new duties.
The outcomes have demonstrated the adaptability of the mixture of expert architects. The advised MoE variant has proven nice efficiency regardless of strict parameter limits, which emphasizes how versatile and efficient MoEs are, particularly in troublesome conditions with constrained assets.
The workforce has summarized their major contributions as follows.
- The analysis presents a singular design incorporating light-weight and modular specialists to enhance the Combination of Specialists (MoEs). This makes it potential to fine-tune dense fashions with low effectivity of lower than 1% parameter updates.
- The advised strategies usually beat typical parameter-efficient strategies in fine-tuning directions, exhibiting higher outcomes on untested duties. Notable enhancements have been achieved by the Combination of (IA)³ Vectors (MoV), which outperforms the usual (IA)³ at 3B and 11B mannequin sizes by as much as 14.57% and eight.39%, respectively. This superiority holds true for a wide range of scales, skilled variations, mannequin sorts, and trainable parameter budgets.
- The examine has proven that, with solely a small proportion of the mannequin parameters up to date, the advised MoV structure can carry out comparably to finish fine-tuning at massive scales. Outcomes from 8 beforehand unpublished duties have proven aggressive efficiency with far decrease computational prices, simply 0.32% and 0.86% of the parameters within the 3B and 11B fashions, respectively.
- In-depth ablation research have been carried out to systematically assess the effectiveness of a number of MoE architectures and Parameter-Environment friendly Advantageous-Tuning (PEFT) strategies, which spotlight how delicate MoE is to hyperparameter optimization and canopy a variety of mannequin sizes, adapter sorts, skilled counts, and routing methods.
Take a look at the Paper and Github. All credit score for this analysis goes to the researchers of this mission. Additionally, don’t overlook to affix our 34k+ ML SubReddit, 41k+ Facebook Community, Discord Channel, and Email Newsletter, the place we share the most recent AI analysis information, cool AI initiatives, and extra.
If you like our work, you will love our newsletter..
Tanya Malhotra is a remaining yr undergrad from the College of Petroleum & Power Research, Dehradun, pursuing BTech in Pc Science Engineering with a specialization in Synthetic Intelligence and Machine Studying.
She is a Information Science fanatic with good analytical and significant considering, together with an ardent curiosity in buying new expertise, main teams, and managing work in an organized method.